In recent years, NVIDIA GPU servers have played a significant role in developing and advancing computing capabilities. This article provides insights into how these modern servers can disrupt how various industries work through their cutting-edge computing power and ease of use. With GPU’s advances, infrastructure becomes less of a bottleneck that cannot keep up with the requirements set forth by AI, machine learning, and big data applications. In this blog, the emphasis will be on GPU servers by NVIDIA – how they work, what impact they have in various sectors, and what the requirements and the real potential of such systems are in today’s computing environments.
What Makes NVIDIA GPUs Ideal for AI Server Solutions?
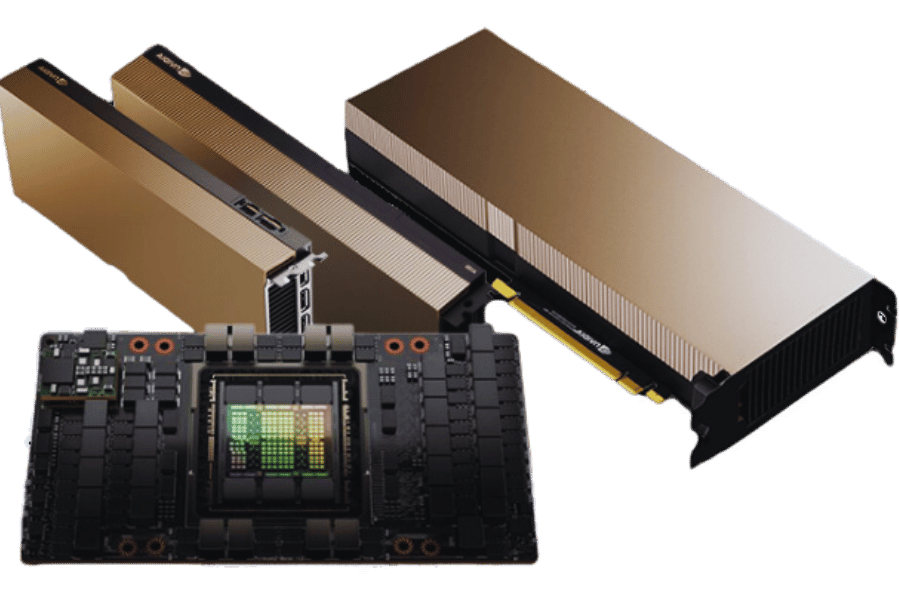
NVIDIA NVIDIA’s graphical processing units (GPUs) are exceptional for AI worker tasks owing to their sophisticated structure tailored for handling parallel tasks. With the presence of the tensor cores, NVIDIA GPUs have been enhanced to perform deep learning workloads and, thus, cut down the training and inference time. Such parallelism enables faster calculation and execution of complex algorithms, which are helpful within the scope of AI. Furthermore, NVIDIA GPUs boast solid compatibility with many AI frameworks and programming languages, which makes it easy to plug them into pre-existing AI workflows. Their scalability permits managing an expanded workload while preserving the system’s state, making them suitable for the increasing AI needs in various sectors.
Exploring NVIDIA’s AI Capabilities
The AI capabilities of NVIDIA’s applications can be seen on numerous sites. Multiple times, It has been mentioned that NVIDIA’s deep learning frameworks and GPU-accelerated computing technology are essential for AI task optimization. What they’re saying, overall, is that with NVIDIA’s devices, one can take advantage of the CUDA architecture, which enables efficient switching of computing processes, hence making the training and inference of AI models more effective. The use of articles has much to say about the ability of NVIDIA’s TensorRT toolkit to enhance the speed at which large neural networks run after they have been implemented on tensor, neural type gpus. In addition, NASA AI has been touted to AI not only for energy efficiency but also for versatility since NVIDIA’s AI could be useful in the areas of medicine, cars, etc, thus providing industries with avenues to integrate and develop solutions based on AI.
The Role of Tensor Core GPU in AI Processing
The pre-training and inference stages of deep learning models are greatly accelerated through Tensor Core GPUs. Some of the websites state that with GPU tensor cores, it’s possible to implement mixed-precision computing where 16- and 32-bit floating-point calculations are performed simultaneously to improve performance throughput. This increase in efficiency translates into a shorter time frame for training AI models that are sophisticated enough to justify real-time inferences used in self-driving cars or voice recognition technologies. Also, GPUs for tensor cores allow many AI applications built on different frameworks like TensorFlow or PyTorch to be incorporated and executed across complex networks effortlessly.
Benefits of Using NVIDIA GPU Servers for AI
According to leading internet sources, the Nvidia GPU servers have several advantages when used to enhance AI applications. First, these servers are powerful enough to deliver unprecedented parallel processing performance, which translates to faster model training and real-time inferences, which are important for deploying AI in high-performance environments. Second, these servers are designed to be energy efficient while sending out high performance, thus cutting losses. Lastly, they come with ample support for multiple AI frameworks, which allow greater flexibility in deploying and scaling the AI models across many use cases. Overall, these benefits make the Nvidia GPU servers necessary in the evolution of AI systems.
How Does the NVIDIA H100 Revolutionize Data Center Operations?
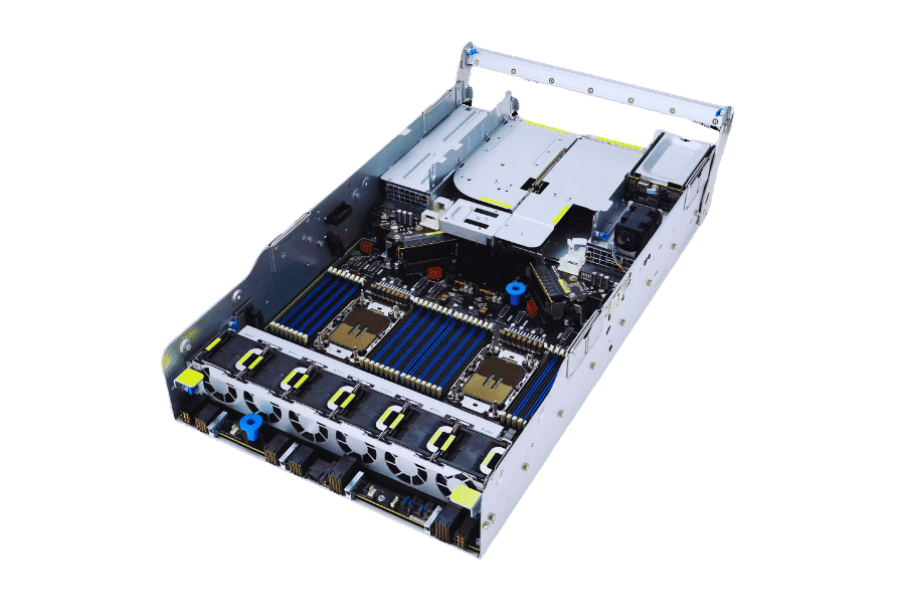
Key Features of the NVIDIA H100
The NVIDIA H100 appears to be a game changer for data centers, to say the least. As per reliable sources, it’s said to have adopted the Hopper architecture, which is particularly developed to cope with the remarkable rise of computational work and the enormous requirements posed by AI and HPC in general. In addition, this GPU incorporates Transformer Engine technology, which boosts training and inference throughput while lowering latency relative to Tesla’s NVIDIA uses. Moreover, the H100 features massively increased memory bandwidth due to its usage of high-bandwidth memory (HBM2e), further boosting data throughputs and processing speeds. More advanced multi-instance GPU (MIG) support greatly enhances partitioning efficiency, enabling more workloads to run simultaneously with the best allocation of resources. Due to these factors, it can be argued that the NVIDIA H100 is a crucial component in developing modern data centers – enhancing current complexity, speed, and degree of parallelism.
Impact on Data Center Efficiency
The inclusion of the NVIDIA H100 GPU has greatly accelerated efforts to improve the efficiency of data centers. A careful review of some reputable sources shows that it boosts performance measurement in several essential aspects. To begin with, the H100 GPU Hopper architecture is not only known to be cost-efficient, but it also lessens the operational costs of operating a data center whilst ensuring maximum production – this is especially important for data centers that handle large amounts of processes. In addition, leading technology websites emphasize the advantages of integrating Transformer Engine technology, which allows for processing AI and HPC tasks at a high rate while simultaneously increasing throughput and latency reduction. This is well supported by the GPU’s specifications, which possess high bandwidth memory (HBM2e) with over 1 TB/s memory bandwidth, enabling data transfer and processing speed. Another important aspect is the improvement of the multi-instance GPU (MIG), which allows multiple workloads to be run in parallel, increasing flexibility and cost-effectiveness. All these technical specifications demonstrate that the H100 has the potential to change how data centers work in terms of efficiency and performance, aided by the specification and performance benchmarks for H100 in comparison with the most authoritative technical data available today.
Comparing NVIDIA H100 with Previous Models
When comparing the architecture of NVIDIA H100 with the architectures of its predecessors, NVIDIA A100 and V100, the most prominent differences become obvious. The A100 makes a startling first impression by exhibiting extreme development, as the analyses done by sources such as NVIDIA, AnandTech and Tom’s Hardware suggest. To begin with, considering the differences between the architectures, the H100 is Tantum architecture-based, which is comparatively more robust and advanced than the A100’s Ampere and V100’s Volta-based architectures. Having 80 billion transistors, the H100 eclipses the A100’s 54 billion, further expanding the bounds of computational capabilities.
Secondly, unleashing the Transformer Engine technology on H100 has enabled the AI and machine learning workloads to process faster than V100, where it wasn’t implemented. Furthermore, the H100 has surpassed A100 in the baselines by moving from HBM2e memory to HBM3, which enhances the memory bandwidth of H100 to over 1TB and enables it to squeeze bandwidth, outperforming A100 1.6 times greater.
Moreover, the H100 has four additional instances compared to the A100 support, enabling it to sustain up to 7 different GPU instances, as per their multi-instance GPU MIG support. These advanced capabilities in the multifaceted operations of the H100 form the basic foundation of its construction aimed at further evolution. In particular, the specifications of the H100 indeed claim impressive improvements in resource throughput efficiency and scaling provisions, which determines its angle as in focus GPU of current period data centers approaches.
Why Choose NVIDIA RTX for Graphic Workloads?
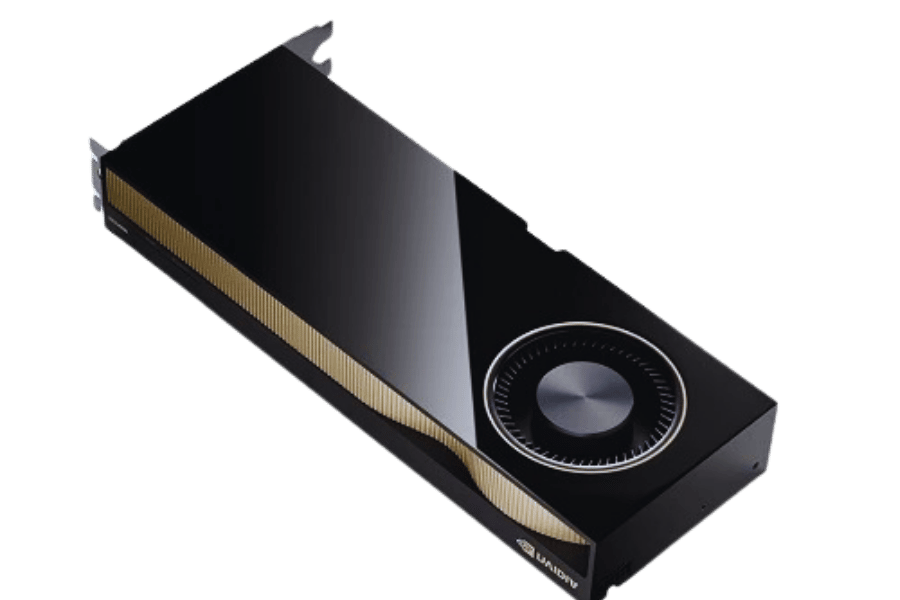
Understanding NVIDIA RTX Technology
According to NVIDIA, the new RTX technology has become a game-changer solution for any graphical workload since the focusing principle comes with ray tracing, programmable shading, and AI learning features. The main benefit of using RTX GPU is that it allows the physical look of light to be simulated, improves image quality, and enhances the effects in graphics rendering. The technology uses RT Cores, which are part of the graphics course and accelerate the ray tracing functions for lighting, shadows, and reflections. Additionally, AI capabilities like NVIDIA DLSS or Deep Learning Super Sampling, which increases framerates while maintaining graphics quality, are also included via Tensor Cores. All of these NVIDIA RTX features make the technology one of the best for workloads that are demanding in power, fast, and have space to expand. RTX technology plays a central role in various sectors such as gaming, film making, virtual reality, and architectural visualization, enabling the user to get a better visual output while consuming fewer resources.
Applications in Professional Graphics
NVIDIA RTX technology is used in various professional graphics applications, incorporating NVIDIA graphics cards. In architectural visualization, RTX DGX Systems with NVIDIA RTX technology enable architects to photorealistically create scenes with accurate lighting and reflections for future decisions and client meetings. For film and animation, RTX Technology significantly shortens the rendering pipeline, allowing for edits to be made on the fly as well as quick edits while retaining acceptable visual quality. Game developers, too, are able to harness the power of RTX GPUs to create realistic environments with appropriate lighting and effects to enhance engagement through improved storytelling. Moreover, in virtual reality, many graphics-intensive applications and interactions can run smoothly with RTX technology, reducing lag and increasing performance. NVIDIA RTX has thus proven to be an invaluable asset for graphic professionals who wish to explore new heights of graphic innovation.
Performance of NVIDIA RTX in Graphic Processing
NVIDIA RTX graphics are said to revolutionize the computer graphics industry with improved performance obtained via AI features, real-time ray tracing, and programmable shading. In this regard, many of the current best websites recommend the service due to its ability to run multiple complex processes simultaneously at a relatively high efficiency and precision. Using Raytracing allows us to obtain realistic shadows and light reflections, which can change the degree of realism in rendered graphics. Other features, such as DLSS, also tend to aid in frame rate increase and image quality without a significant impact on system requirements, aiding performance across many platforms. Furthermore, second-generation RT and third-generation Tensor cores greatly enhance the processing power embedded within NVIDIA graphics cards and aid in performing such graphical operations. Thus, it is safe to say that NVIDIA RTX is a suitable technology for any graphic-intensive need, especially in gaming, film, and design, as it brings many new possibilities and increased productivity through the application of NVIDIA Ampere technology.
What Are the Benefits of GPU Server Plans with NVIDIA?
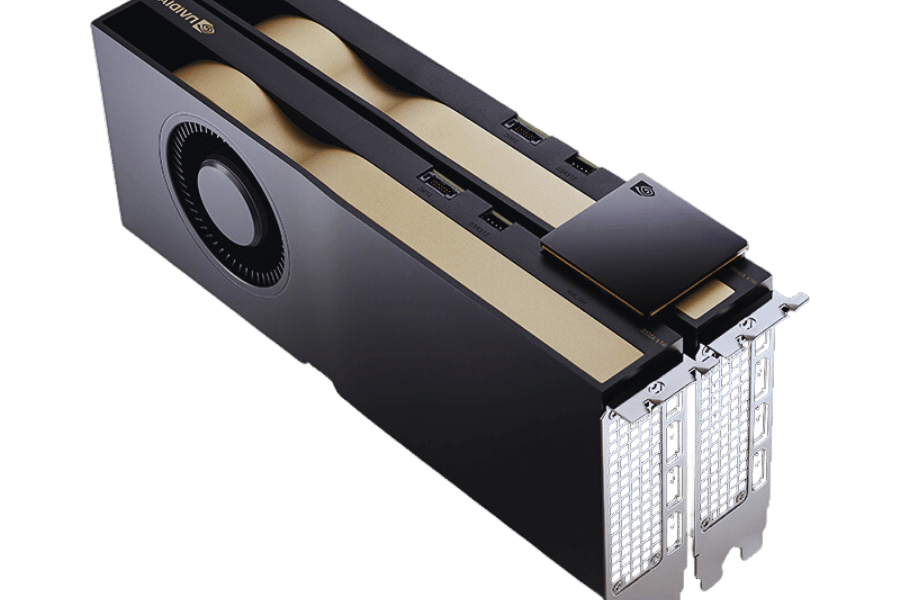
Exploring Dedicated GPU Server Options
Dedicated GPU servers powered by NVIDIA technology are a great advantage for high-performance computing workloads. From current leading sources it can be clearly seen that these dedicated servers are built for working with heavy loads like, for example, machine learning, scientific modeling, and real-time data. The flexibility in resource distribution especially helps to reduce costs while resource efficiency measures using massively parallel architectures of NVIDIA graphics processors. Other benefits, such as enhanced security, increased fault tolerance, expansion possibilities, and management capabilities, also increase the overall attractiveness of these servers, especially for enterprises that require high-performance and high-capacity data processing solutions. It is for rendering complex graphics or accelerating AI application rendering that dedicated GPU server plans are designed to enhance computing performance without coupling latency with slowness when computing tasks.
How GPU Server Plans Enhance Scalability
GPU server plans elevated scalability boundaries by incorporating flexible resource management which allows the usage of computational resources to grow with the demand. This capability guarantees that resources can be increased to handle peak processing loads or minimized to save money, thus allowing a consistent level of implemented performance without the need to forecast and over-provisioned the facilities. Moreover, thanks to NVIDIA’s GPU technology, such server plans also allow high and low latency, offering operational compatibility across different spatial ranges, such as deep learning models or sophisticated data analytics. The geopolitical borders of scalability have been expanded by cloud-based integration, which provides virtualization of resources and scaling at the use-request level, making scalable infrastructures even more efficient in terms of adaptability and time than cost. These advanced technologies and methodologies are synthesized in the GPU server plans, allowing delivery of high flexibility and control, which the contemporary continuously changing computing environments require.
Cost-Effectiveness of NVIDIA GPU Servers
Enterprises adopting NVIDIA GPU servers can reap the advantages of accelerated computing while reducing operational costs. According to the top experts in the field, these servers are efficient in terms of energy used and hardware needed as they yield very high performance even with very little infrastructure. Economy of scale is achieved by the virtualized architecture, which limits the number of physical servers a business would need to manage, thus reducing capital and operating overheads, including cooling and space. Moreover, as the technology developed by NVIDIA GPUs can leverage multiple tasks simultaneously, efficiency will be guaranteed. Other authorities have indicated that transitioning toward GPU-enhanced servers, specifically servers using NVIDIA GeForce GTX technology, is a sustainable approach that in turn can support future growth with little investment in new technologies. In summary, NVIDIA GPU servers constitute a sound investment for the companies that focus on both the performance and the overall investment in the information technology strategy.
How Do NVIDIA Quadro Solutions Enhance HPC Performance?
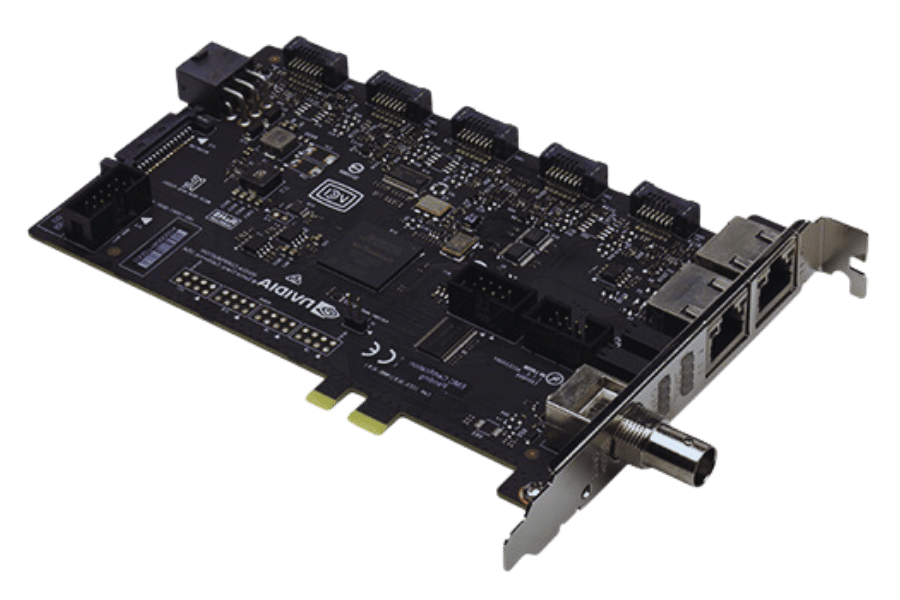
Key Features of NVIDIA Quadro for HPC
NVIDIA Quadro models improve high-performance computing (HPC) productivity owing to several key elements, one of which is the support of the NVIDIA Tesla architecture. First, with the Quadro range of GPUs, there is an advance in the capability to process in parallel. This means that complex computations, their trademark in the HPC tasks, can be quite well accomplished. Second is enhanced memory and bandwidth capacity, which are always critical in storing large datasets and speeding up data warehousing operations. Another significant advantage of NVIDIA Quadro is its ability to produce high-quality graphics, particularly for multitudinous rendering as needed in scientific and engineering visualization applications. In addition, these GPUs are also stable and reliable; hence they are expected to perform well in most computing environments. Finally, Quadro solutions are intended to be integrated with a variety of HPC applications and, hence, complement various activities. Such capabilities enable institutions to harness HPC resources more effectively regarding computational performance and scalability.
Integration with HPC Systems
Using NVIDIA Quadro in high-performance computing class systems makes the systems more efficient and scalable due to the balance of hardware and software. Quadro GPUs are developed to connect efficiently with current HPC infrastructures like Infiniband fabric and parallel file systems to increase data exchange bandwidth while decreasing latency. These NVIDIA GPUs enhance the development and execution of code by providing CUDA and different NVIDIA software ecosystems that conform to regular high-performance computing procedures. This kind of integration ensures optimal utilization of resources and scaling of on-demand computing resources as the project needs change. Therefore, institutions can be highly efficient in carrying out tasks related to processing scientific or engineering models at a great speed, making it easier for them to carry out research and innovation.
Advantages of Using NVIDIA Quadro in High-Performance Computing
In high-performance computing, NVIDIA Quadro solutions would have a couple of advantages. To begin with, they have to achieve high levels of performance and precision, which are critical for working with intricate mathematics and physics. The design of Quadro GPUs was focused on parallel computing, effectively cutting down computation time and improving the work efficiency. Also, they enhance operational efficiency by easing workflow integration with various HPC software, enabling faster GPU performance in the data center. The cutting-edge capability of AI and ML applications provides an opportunity for researchers to apply models that are afraid of big data, and these models make the workload very manageable. Additionally, Quadro GPUs have a high level of fault tolerance, which reduces outages and guarantees the operation of hardware undertakings in overloaded conditions. Together, these features ensure that the clients will get the best performance and capacity in their HPC applications.
Reference Sources
Frequently Asked Questions (FAQs)
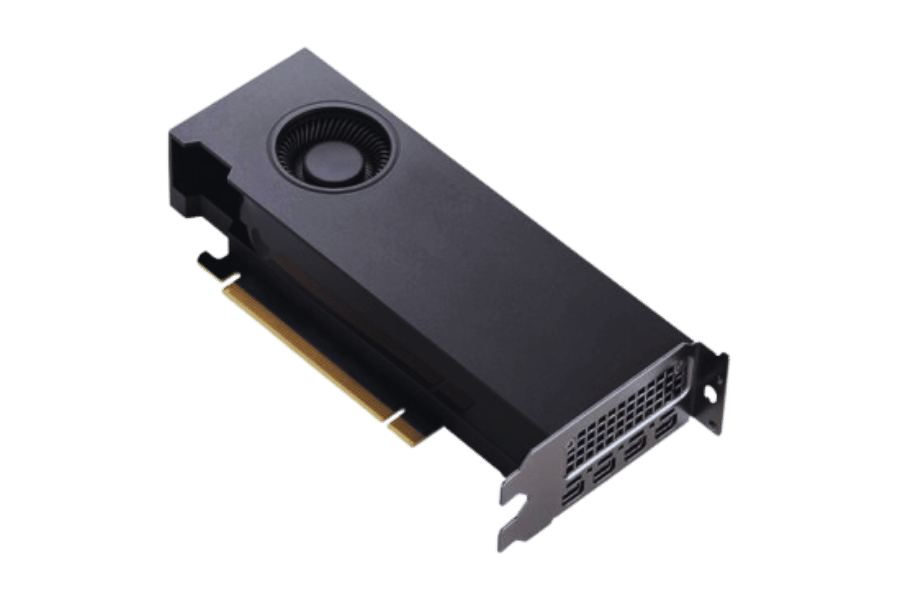
Q: What computing benefits accrue throughout the use of servers that are equipped with NVIDIA GPUs?
A: The NVIDIA GPU servers offer notable benefits for advanced computing, such as increased performance for deep learning and AI applications, increased processing power, and the ability to perform complex computations. These servers utilize the GPU to process a high volume and high speed of data and are suitable for data-intensive operations.
Q: How do GeForce graphics cards increase graphics processing by adding the NVIDIA GeForce RTX series?
A: The NVIDIA GeForce RTX series of graphics cards include ray tracing and AI-embedded graphics rendering, which enhance graphics processing by creating real-time environments with realistic reflections, shadows, and lights. This series also augments the gaming experience and other professional graphics applications because of the powerful GPU technology integrated into it.
Q: In what applications can the NVIDIA RTX A6000 graphics card for professional workloads be utilized?
A: The A6000 RTX Graphics card from NVIDIA has a considerable number of NVIDIA graphics processing units and a high memory capacity, which makes it ideal for professional workloads. It’s ideal for industries like media, architecture, and entertainment that require speed with AI development and rendering along with great power graphics through NVIDIA Amp, which offers a variety of features.
Q: In what respects does NVIDIA L40s 48GB PCIe bolster the performance of data-driven workloads?
A: The increase in memory and efficient processing power make the NVIDIA L40s 48GB PCIe model suitable for workloads that involve large data sets, deep learning, data analytics, scientific simulation, etc.
Q: Why is NVIDIA Virtual GPU (vGPU) technology indispensable in today’s computing environments?
A: Virtualization has established itself as a force in today’s driving economy, and NVIDIA Virtual GPU (vGPU) Technology is a key to achieving true virtualization. Vgpu solves the issue of scaling HP blades, allowing for multiple servers in an enterprise setting. This tech is a must for virtual desktops, cloud-based solutions, and virtualized applications.
Q: What advantages does the NVIDIA Quadro RTX 4000 provide to creative leaders in their tasks?
A: Pawning performance mountings from advanced rendering and visual computing. The NVIDIA Quadro RTX 4000 supports enough graphics cards to run numerous multiple output displays and is versatile enough to be used with video games, animations, and even several professional industries.
Q: In what ways will a GPU VPS powered by NVIDIA be beneficial in enabling cloud computing?
A: Important workloads can work in an accelerated fashion when aided by NVIDIA graphic-powered servers through the use of a GPU VPS. This has tremendous value in AI, graphics, and other applications that require rapid computation for success.
Q: In what way is the NVIDIA H100 NVL 94GB more suitable for AI research than all other options on offer?
A: This device can facilitate numerous sessions and computations because it provides great memory resources and offers a strong GPU architecture required for parallel computing. This boosts the potential for R&D expansions within usable AI domains.
Q: Of all the NVIDIA products, which are the A16 and A2 GPUs, and how long do they last, and in what countries?
A: While most NVIDIA graphics cards possess areas of expertise within their range, the A16 and A2 GPUs seek to expand within the rest. The A16 aims towards virtual desktops that can have numerous users with VDI characteristics, while the A2 caters to entry-level AI users.
Q: In what way is the universal GPU Concept advantageous to data centers employing NVIDIA technology?
A: The universal GPU concept assists data centers with a more adjustable and elastic GPU architecture, enabling them to tackle different tasks ranging from AI to graphics. By employing Nvidia technology, it permits data centers to enhance their infrastructure, save expenses, and operate an array of programs using one universal GPU platform.