The use of artificial intelligence (AI) has boosted development in the world today by enhancing various sectors like health, finance, and self-driving cars, among others. The requirement for more computational power increases with a growing number of AI applications. NVIDIA is known to produce state-of-the-art supercomputers optimized for heavy-duty AI workloads. DGX and HGX are two famous product lines under NVIDIA’s supercomputer portfolio. This piece seeks to bring out disparities between NVIDIA DGX and NVIDIA HGX supercomputers so that institutions or scholars can have a clear understanding when choosing the best fit for their AI computations requirements.
What are the key differences between NVIDIA DGX and NVIDIA HGX?
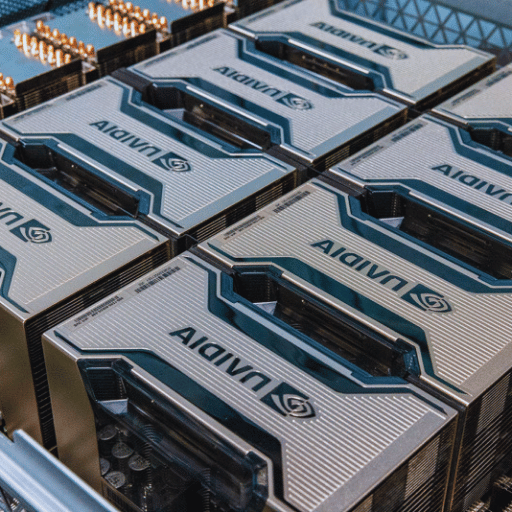
Overview of NVIDIA DGX and HGX Systems
AI development and training systems are what NVIDIA DGX was made for. These systems have integrated hardware and software stacks that are optimized for deep learning as well as GPU-accelerated tasks. To this end, it includes pre-configured settings and easy deployment protocols, all supported by a software ecosystem from NVIDIA, which makes them perfect for turnkey solutions in research or enterprise environments.
On the other hand, High-Performance Computing (HPC) servers were built with scalability in mind – enterprises such as data centers where AI workloads may be distributed across many machines. NVIDIA’s design of these servers allows them to be customized according to specific needs; this is achieved through modularity features that make use possible within large-scale infrastructures like those found at data centers. As opposed to being limited only to certain types of CPUs or networking configurations as they would have been if designed specifically for one purpose like training models on massive amounts of data using deep neural networks – they can work with any CPU architecture depending on what works best given different cases but still offer flexibility alongside support needed while working within an organization’s unique setup.
Detailed Comparison: NVIDIA DGX vs. NVIDIA HGX
Goals and Applications
- NVIDIA DGX: AI development, research, and training with plug-and-play solutions.
- NVIDIA HGX: HPC and scalable AI infrastructure for different customization options.
Hardware Compatibility
- NVIDIA DGX: Integrated hardware-software stacks with preset configurations.
- NVIDIA HGX: Component-based design that can be flexibly combined with various CPU architectures.
Support & Deployment
- NVIDIA DGX: Simple deployment procedures backed by NVIDIA’s vast software ecosystem.
- NVIDIA HGX: Customizable computing for tailored deployments and integration is required.
Scalability
- NVIDIA DGX: Works best in small to medium-sized enterprises or research facilities.
- NVIDIA HGX: Scalable across large data centers involving distributed AI workloads.
Customization Potential
- NVIDIA DGX: Not much can be customized here since it is designed to work straight from the box.
- NVIDIA HGX: Can be highly customized to suit specific needs or requirements of users.
Performance Optimization
- NVIDIA DGX: It has been optimized mainly for deep learning and GPU-accelerated tasks in general.
- NVIDIA HGX: It has been optimized primarily for high performance scalability.
How does AI performance differ between the NVIDIA HGX and the NVIDIA DGX?
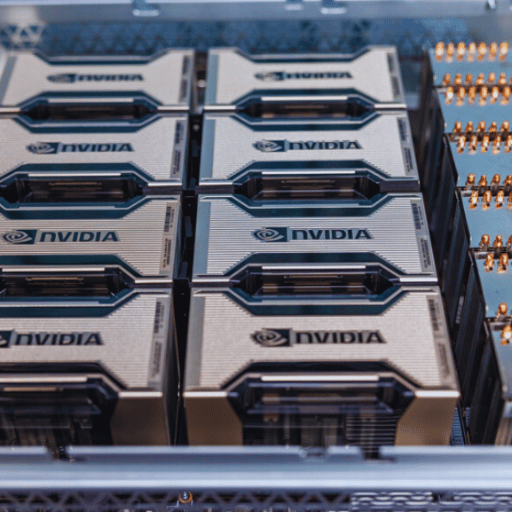
The Requirements of Generative AI
Generating AI (generally applied to training large-scale language models or creating advanced artificial intelligence applications) needs a lot of computation power as well as efficiency in processing large amounts of information.
- Performance & Throughput: In comparison with each other, the two systems differ mainly in terms of performance and throughput. While DGX can be used for low latency inference when it is necessary to carry out real-time generation tasks, on the other hand, HGX is designed specifically with attention paid towards extensive datasets that are very computationally intensive and typically run on big clusters or supercomputers. This means that this platform will handle them much better than any other system available today would do so because its architecture allows integration into CPUs having different configurations, thus making possible support for diverse combinations such as those involving AMD EPYC processors together with multiple A100 GPUs interlinked through NVLink.
- Deployment & Convenience: There is also a distinction between these two platforms regarding deployment options and convenience levels offered by them – while being more flexible than DGXs when it comes down to scalability in terms of size or number. For example, one can start off small, using only a few units, then gradually add more as demand necessitates, but at some point, you may need many units, which might require high-quality cooling systems due to increased power consumption.
In conclusion, while Nvidia HGX provides flexibility needed by organization engaged in wide generative use cases requiring availability always-on unlimited resources scaling across multiple racks full gear up its sleeves pure compute muscle DGx delivers fast time-to-market through simplicity ease-of-use optimization around most common deep learning frameworks libraries pre-installed ready work out box laptop-like form factor quiet operation easy transportability among others
Data Centers Performance Optimization
When it comes to optimizing data center performance with AI, what are the differences between NVIDIA HGX and NVIDIA DGX?
- NVIDIA HGX: The best way to optimize performance in a data center is through scalability that is unmatched by any other platform. This allows for the integration of different types of CPUs as well as large-scale handling and processing of data which means higher throughput being achieved while still improving efficiency at the same time. Such characteristics make this system more flexible than others especially when working with NVIDIA’s DGX.
- NVIDIA DGX: Another option for those who want optimized performance within their data centers would be going for an all-inclusive package like NVIDIA DGX systems. These come ready to use right out of the box hence saving on time needed during setup or installation. They also have built-in hardware and software components designed specifically for deep learning applications thus making them easy to integrate into any existing environment where such capabilities are required most urgently. With these features, organizations can start their AI research programs much faster than expected.
To choose between NVIDIA HGX and DGX depends on what you want your center do: scalability or optimized deep learning capabilities respectively
Distinctive Attributes of HGX for Artificial Intelligence Workloads
NVIDIA HGX has a number of unique features that were created specifically for the improvement of AI workloads. These include:
- Scalability: It can scale horizontally or vertically thus allowing expansion with the growth in size of AI datasets as well as computational requirements.
- Flexibility: Designed with different CPUs in mind so that they can easily fit into any data center environment without causing compatibility issues.
- Throughput: Capable of handling large amounts of information by optimizing its data management functions.
- Advanced networking: It uses high-speed interconnects to reduce latency between components while increasing their data transfer speeds.
- Energy efficiency: Its energy consumption is within acceptable limits but at the same time delivers high performance which cuts down on operation costs. This is especially notable when using NVIDIA HGX A100 and HGX B200 platforms.
- Modularity: Can be upgraded or modified easily to be compatible with future AI technologies hence it’s flexibility also ensures that it remains useful even as AI evolves over time.
What’s the Better Choice for AI Projects: NVIDIA HGX or NVIDIA DGX?
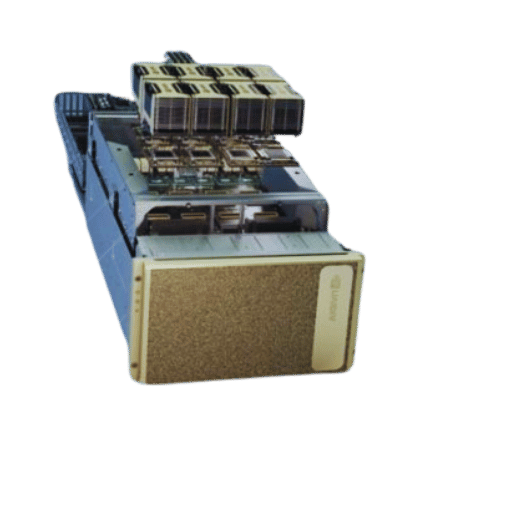
DGX and HGX Platforms’ Advantages and Disadvantages
NVIDIA DGX
Advantages:
- Simplification of deployment – Simplifies installation and setup processes.
- Deep learning optimization – Optimized for Deep Learning, with a complete hardware-software stack designed specifically for machine learning.
- A solution ready to go – It provides an all-in-one package that removes the need for additional configuration.
Disadvantages:
- Scalability is limited– Not much flexibility when it comes to large-scale or customized configurations.
- Expensive– Integrated solutions are priced at a premium.
- Use case specific– It is primarily suited for deep learning and may lack adaptability to other applications.
NVIDIA HGX
Advantages:
- Scalable – Excellent horizontal as well as vertical scaling AI infrastructure.
- Flexible – Supports a variety of CPU architectures which enhances flexibility in deployment.
- High-performance – High throughput along with advanced networking meant for data-intensive workloads.
Disadvantages:
- Complex deployment – More expertise required in configuration and integration, especially while setting up DGX station(s).
- Modular costs- There might be higher costs with custom setups. Energy consumption: Large scale but energy-efficient deployments could still consume significant power.
Assessing Your AI Needs
- Size of the Project: Should you be working on a more turnkey solution (NVIDIA DGX) or scalable infrastructure (NVIDIA HGX)?
- Money Constraints: Determine whether it is best to use all your budget at once with DGX or if HGX will offer better customization options but potentially cost more in the long run.
- Specific Use Case: Is deep learning your only focus? If not, then go for the broadest possible range of artificial intelligence applications – NVIDIA HGX.
- Technical Capacity: Can you manage intricate setups (NVIDIA HGX) or do you require simple installation process provided by NVIDIA DGX?
Required Performance Level: Which one would serve you better between high throughput and advanced networking capabilities brought about by HGX vs optimized deep learning performance offered by DGX?
What are the Specific Features of HGX and DGX?
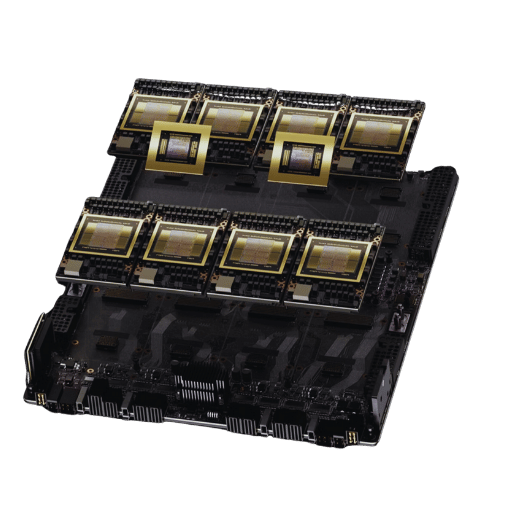
Advanced Cooling Systems in HGX Platforms
In order to handle dense computational workloads, which produce a lot of heat, HGX platforms have advanced cooling systems. Typically, such cooling solutions use liquid cooling technology that is more effective in dissipating heat than traditional air-cooling methods. It consists of cold plates connected to heat exchangers through pumps that efficiently transfer thermal energy away from critical parts to keep them at their optimum operating temperatures. This way, even during tough working situations, the system will continue performing at its peak efficiency, thus making it reliable and less vulnerable to thermal throttling.
HGX H100 vs. DGX H100 Comparison
HGX H100:
- Target Audience: These are large companies that have already advanced their technical capabilities.
- Configuration: Highly customizable configurations suitable for complicated environments.
- Performance: Works best in high-throughput or specialized applications.
- Cooling: Maximum efficiency through advanced liquid cooling systems.
DGX H100:
- Target Audience: These organizations have low technical resources.
- Configuration: It is pre-configured to make deployment faster and easier.
- Performance: Optimized for deep-learning tasks that can be done with different models such as transformers, RNNs, CNNs and etceteras.
- Cooling: Cooling system can be air cooled or basic liquid cooling which can do the job more efficiently.
8x NVIDIA Configurations Unveiled
When we talk about 8x NVIDIA configurations, we mean systems that can hold eight graphics cards. These are made to give the highest possible amount of computing power and efficiency for high-performance computing (HPC), data analytics, and artificial intelligence (AI).
Noteworthy Points:
- Scalability: It is important because it allows massive parallel processing among many GPUs which in turn enables large scale deployment support.
- Performance: This is necessary when dealing with workloads that have a lot of computations by giving them extra speed.
- Flexibility: These can be adjusted so as to fit any given need thus making them applicable in different computing environments where versatility is needed most.
These configurations ensure that all the components work together as one unit so that everything runs smoothly. In other words, they can handle complex data sets quickly while also speeding up calculations by using higher numbers or faster speeds, depending on what needs to be done.
What are AI and Data Center Environments like for NVIDIA HGX Systems?
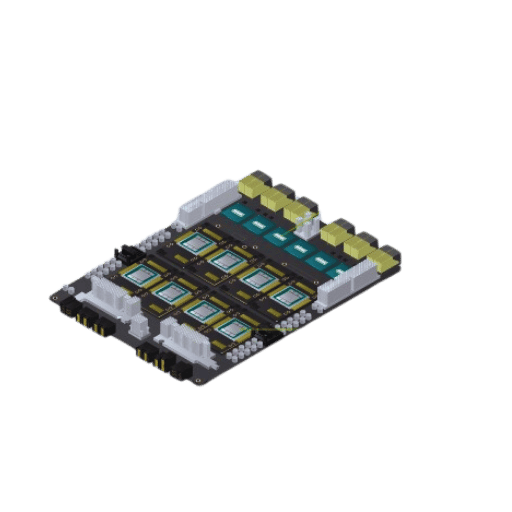
NVIDIA HGX Systems Ecosystem
NVIDIA HGX Systems Ecosystem is great in both AI and data center environments because it’s strong and powerful.
- Performance: It provides unmatched performance for AI model training and inference by giving excellent computing power.
- Scalability: This allows for the scaling up of artificial intelligence operations as well as machine learning with seamless integration into current data centre infrastructures.
- Efficiency: High energy efficiency is guaranteed through optimized power usage coupled with advanced cooling mechanisms.
These functionalities together position NVIDIA HGX Systems as an essential tool for improving enterprise-level data analytics as well as Artificial Intelligence research.
Flexibility and Scalability of HGX Platforms
HGX Platforms are very flexible and scalable as they can be deployed in different ways depending on the situation.
Thus, these platforms can adapt to various computing demands.
- Variability: The systems may be adjusted by changing their setups to support many types of artificial intelligence (AI) tasks and data processing needs too.
- Growth: With this infrastructure technology, expansion becomes limitless since it supports improved performance levels as more computation is done.
- Integration: Establishing connection with current infrastructures enables easy scaling up without heavy alterations on operational methods used in a company or an organization.
In summary, HGX Platforms offer powerful solutions applicable in both AI labs and data centers while still remaining efficient.
HGX Systems Cost Considerations
To answer the cost considerations for HGX systems, there are a number of factors that need to be taken into account.
- Initial investment: The initial price of purchasing an HGX system can be high because it has advanced features and a robust design.
- Operating expenses: Continuous running costs include power consumption, cooling needs and regular maintenance.
- Scalability costs: Although being scalable is a good thing but as the system grows larger there will be additional spending on hardware upgrades and infrastructure changes required.
- Return on Investment (ROI): Increase in computing efficiency should be measured against total outlay in terms productivity gains realized from using this kind of system.
- Life-cycle Costs: This involves looking at all costs associated with owning such a system over its lifetime which may involve future upgrades or even decommissioning them where necessary.
In conclusion, undertaking comprehensive analysis on costs would enable individuals make right choices during their investments into HGX systems.
Reference Sources
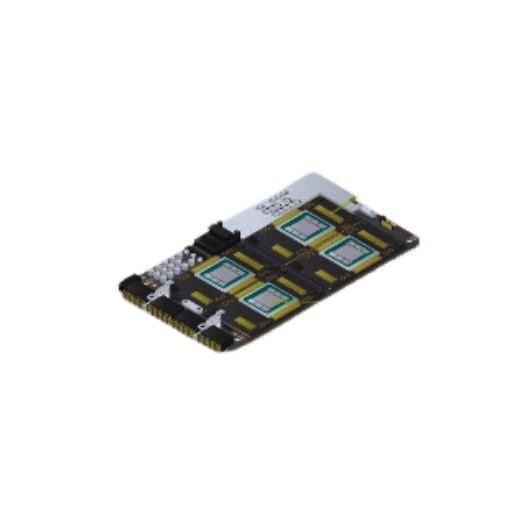
Frequently Asked Questions (FAQs)
Q: In AI applications, what are the main differences between NVIDIA DGX and NVIDIA HGX?
A: When it comes to architecture and use cases, the difference between NVIDIA DGX and NVIDIA HGX platforms is profound. Ready-to-deploy AI and deep learning workflows are supported by DGX systems like DGX A100. On the other hand, modular designs were employed in creating scalable solutions integrated into data centers to provide very strong AI capabilities which is represented by NVIDIA HGX.
Q: How does the DGX A100 compare to the NVIDIA HGX A100 in terms of performance?
A: For immediate AI workloads optimization purposes, this self-contained system called DGX A100 has been created with 8x NVIDIA A100 GPUs in it. Another option is a modular configuration that can be scaled across data centers hence achieving greater aggregate performance due to integration of multiple GPUs and high-speed interconnects known as NVIDIA HGX A100 platform.
Q: What is the purpose of the NVIDIA DGX H100?
A: The latest product in the series of high-performance AI and deep learning applications designed by Nvidia Corporation under its brand Nvidia dgx h100 is here! It inherits all features from previous models but adds some computational power improvements along with efficiency enhancements thanks to new hpc technology nvidia h100 gpus integration.
Q: Can you explain liquid cooling in these systems?
A: Liquid-cooled nvidia hgxa delta uses this method for maintaining optimum temperatures during intense computational tasks thereby increasing performance as well energy savings.
Q: What is the NVIDIA DGX SuperPOD?
A: Multiple dgx systems combined together create an extensive computing environment capable of performing large scale ai and deep learning operations faster known as dgx superpod powered by nvidia nvswitch technology for gpu accelerated computing.
Q: How does DGX B200 differ from DGX GB200?
A: These are some models of the DGX series with different configurations and performance capabilities for various AI workloads. They vary in performance and application by architectural and GPU configuration differences.
Q: What are the advantages of NVIDIA HGX H100 platform?
A: The NVIDIA HGX H100 platform is equipped with the latest NVIDIA H100 GPUs thus enhancing high-performance computing for AI applications. The capability to scale, modular design as well as advanced interconnects like NVLink and NVSwitch for data centers which maximize their performance.
Q: How does “NVIDIA DGX versus NVIDIA HGX” comparison affect decision-making in AI infrastructure?
A: By comparing NVIDIA DGX against NVIDIA HGX systems, enterprises can make informed choices based on their requirements. While DGX systems are good for ready-to-deploy AI solutions; HGX provides more flexibility and power in large-scale deployments where data center integration is required thus becoming scalable and high-performance oriented.
Q: What is the role of NVIDIA Tesla GPUs in DGX and HGX platforms?
A: In both DGX and HGX platforms, powerful computing capabilities have been delivered by NVIDIA Tesla GPUs such as NVIDIA Tesla P100 for AI as well as deep learning workloads. Modern systems come with more advanced GPUs like A100 plus H100 that provide even higher levels of performance.
Q: Why is “difference between NVIDIA HGX and DGX” important for AI development?
A: Knowledge about the dissimilarity between these two influences infrastructure selection during artificial intelligence development hence being critical. Out-of-the-box solutions suit best on DGX while scalable modular configurations which can be customized extensively for enterprise research or deployment environments form part of what makes up an ideal HGX system.
Related Products:
-
NVIDIA MMA4Z00-NS400 Compatible 400G OSFP SR4 Flat Top PAM4 850nm 30m on OM3/50m on OM4 MTP/MPO-12 Multimode FEC Optical Transceiver Module $650.00
-
NVIDIA MMA4Z00-NS-FLT Compatible 800Gb/s Twin-port OSFP 2x400G SR8 PAM4 850nm 100m DOM Dual MPO-12 MMF Optical Transceiver Module $850.00
-
NVIDIA MMA4Z00-NS Compatible 800Gb/s Twin-port OSFP 2x400G SR8 PAM4 850nm 100m DOM Dual MPO-12 MMF Optical Transceiver Module $750.00
-
NVIDIA MMS4X00-NM Compatible 800Gb/s Twin-port OSFP 2x400G PAM4 1310nm 500m DOM Dual MTP/MPO-12 SMF Optical Transceiver Module $1100.00
-
NVIDIA MMS4X00-NM-FLT Compatible 800G Twin-port OSFP 2x400G Flat Top PAM4 1310nm 500m DOM Dual MTP/MPO-12 SMF Optical Transceiver Module $1200.00
-
NVIDIA MMS4X00-NS400 Compatible 400G OSFP DR4 Flat Top PAM4 1310nm MTP/MPO-12 500m SMF FEC Optical Transceiver Module $800.00
-
Mellanox MMA1T00-HS Compatible 200G Infiniband HDR QSFP56 SR4 850nm 100m MPO-12 APC OM3/OM4 FEC PAM4 Optical Transceiver Module $200.00
-
NVIDIA MFP7E10-N010 Compatible 10m (33ft) 8 Fibers Low Insertion Loss Female to Female MPO Trunk Cable Polarity B APC to APC LSZH Multimode OM3 50/125 $47.00
-
NVIDIA MCP7Y00-N003-FLT Compatible 3m (10ft) 800G Twin-port OSFP to 2x400G Flat Top OSFP InfiniBand NDR Breakout DAC $275.00
-
NVIDIA MCP7Y70-H002 Compatible 2m (7ft) 400G Twin-port 2x200G OSFP to 4x100G QSFP56 Passive Breakout Direct Attach Copper Cable $155.00
-
NVIDIA MCA4J80-N003-FTF Compatible 3m (10ft) 800G Twin-port 2x400G OSFP to 2x400G OSFP InfiniBand NDR Active Copper Cable, Flat top on one end and Finned top on other $600.00
-
NVIDIA MCP7Y10-N002 Compatible 2m (7ft) 800G InfiniBand NDR Twin-port OSFP to 2x400G QSFP112 Breakout DAC $200.00