The Nvidia H200 graphics processing unit (GPU) significantly advances computational performance and technological innovation. This manual examines the features, performance measures, and possible applications of the H200 across different industries. Gaming and virtual reality, artificial intelligence, and scientific computing are some of the areas where this device is expected to perform exceptionally well. By looking at its architectural improvements, power efficiency, and integrated technologies systematically, readers will gain a comprehensive comprehension of what makes the Nvidia H200 different from other products in the market today through this guide. Whether you’re a tech enthusiast or someone who works with computers for a living, even if you just want a better gaming experience — this complete guide has everything that can be said about it: The Nvidia H200 GPU’s astonishing abilities.
What is the Nvidia H200?
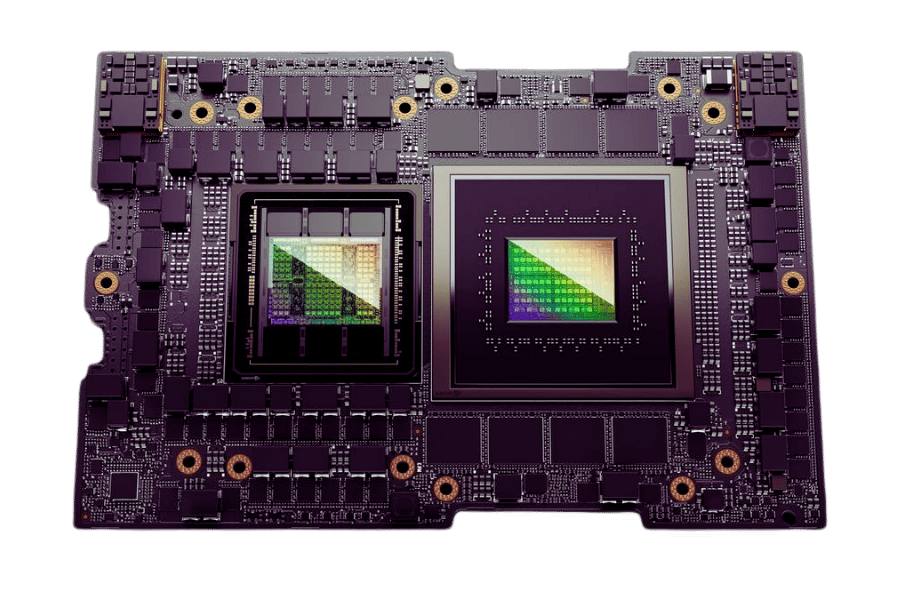
Introduction to Nvidia H200
Nvidia H200 is the latest GPU from Nvidia that has been created with the aim of stretching graphic and computing capabilities. This complex hardware uses advanced architecture developed by Nvidia, which includes real-time ray tracing among other cutting-edge technologies like Tensor Cores for AI acceleration as well as improved memory bandwidth. Designed to be fast while at the same time efficient, this version of H200 can work for many different purposes, including high-end gaming, virtual reality (VR), professional visualization applications such as CAD/CAM systems or simulations for deep learning networks used in natural language processing (NLP) tasks among others – it can also be used by scientists performing various kinds of experiments where large amounts of data need to be processed quickly. By doing all these things simultaneously, this device sets a new bar within the GPU market space where, before, no other card had ever come close in terms of performance plus versatility offered!
Key Features of Nvidia H200
- Real-Time Ray Tracing: The H200 is a real-time application and game that provides the most realistic lighting, shadows, and reflections ever seen using ray tracing technology augmented by its advanced capabilities.
- Tensor Cores: The H200 has a lot of AI power because it is equipped with special Tensor Cores, which enable it to perform computations related to deep learning and other artificial intelligence tasks much faster than any other device can.
- Enhanced Memory Bandwidth: The improved memory bandwidth on this thing ensures that scientific simulations, data analysis, or any other computational workload needing higher speeds get done quickly, making NVIDIA’s new GPU one hell of an accelerator!
- Efficiency plus Speed: What makes H200 different from others is not only its efficiency but also the fact that it is designed for speed. It performs multiple operations within seconds, saving more energy without compromising processing capacity.
- Versatility in Applications: Due to its strong structure, such hardware can be used for many things, like gaming purposes at high-end levels, virtual reality device development, or professional visualization programs, amongst others, while still being able to handle scientific calculations dealing with large sets of information.
This unique combination puts Nvidia’s latest graphics card, the H200, among the top choices when selecting GPUs. They offer unbeatable performance standards alongside their flexibility, which supports various technical and professional needs alike.
Comparing Nvidia H200 with H100
When one compares Nvidia’s H200 and its predecessor, the H100, certain key differences and enhancements can be seen.
- Performance: Compared to the H100 SXM, the H200 has considerably faster processing speed and graphics performance due to more advanced real-time ray tracing and increased memory bandwidths.
- AI Capabilities: The H200 features upgraded Tensor Cores while the H100 has Tensor Cores; this means that it has better deep learning capabilities and AI acceleration.
- Energy Efficiency: Power efficiency is designed into its system architecture so that it provides more watts per performance than any other product of a similar class in its category, such as H100.
- Memory Bandwidths: For high-performance computing (HPC) or advanced simulations where higher data throughput is required, then beyond doubt, there is a need for increased memory bandwidth supported by an upgrading from h100, which only supports low-performance computing (LPC).
- Versatility: Although they are both versatile models, between these two options, next-gen virtual reality will demand much from hardware, hence making the robust nature within the h200 model appropriate alongside professional visualization plus other compute-intensive applications when compared against h100 versions, which were less robust.
To put it briefly, Nvidia’s H200 is much better than the previous generation in terms of performance speed, AI capability improvement rate, energy-saving feature adoption level, and application versatility range, hence making it ideal for use in complex technical or professional setups.
How Does the Nvidia H200 Enhance AI Workloads?
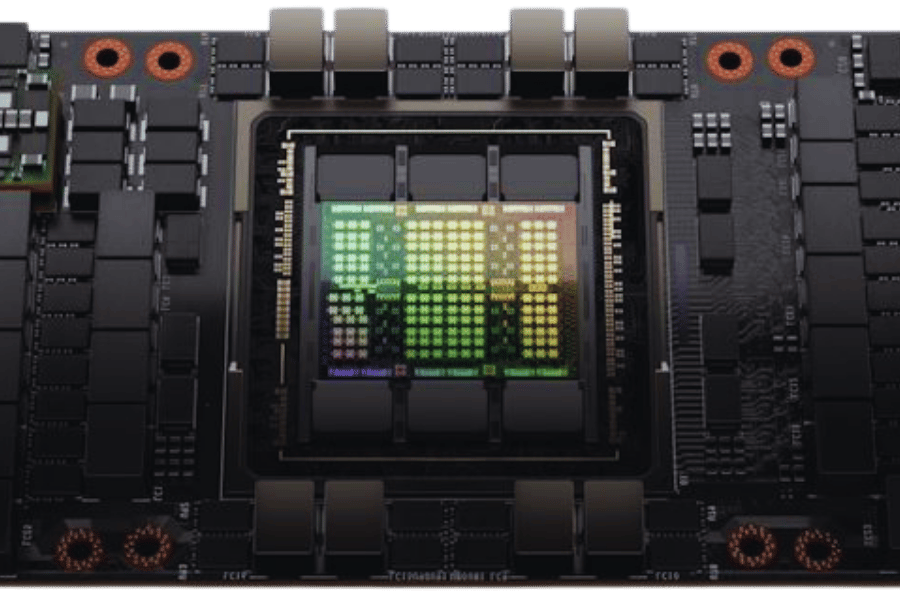
Generative AI Improvements
The H200 chip from Nvidia is a big step forward in generative artificial intelligence, and it does this by improving on last year’s model, the H100. There are three major ways in which it does so:
- Better Tensor Cores: Having updated tensor cores means that matrix operations, which are essential for generative models, can be done more efficiently by the H200 chip. Therefore, training times and inference time will be quicker, too, but what’s even better about them is that they make it possible for AI to create more complicated and higher-quality content.
- More Memory Bandwidth: This new chip has much higher memory bandwidths, allowing larger data sets and, therefore, bigger models to be run on them. So now, these chips can handle complex artificial intelligence systems using huge amounts of information. This is especially good when making high-resolution images or language models with lots of training material needed.
- Real-Time Ray Tracing Improvements: The inclusion of enhanced real-time ray tracing into the H200 greatly improves rendering fidelity within produced visuals. Things like more realistic simulations are now achievable, while at the same time, visual quality during tasks such as creating generated designs is taken advantage of using ideas brought about by previous generation tensor core GPUs like those found on board an H100 unit from Nvidia.
With all these features combined, one might say that this device represents a great leap forward for generative AIs – enabling developers to create highly sophisticated systems faster than ever before!
Deep Learning Capabilities
The Nvidia H200 boosts deep learning capabilities in various ways:
- Hardware Architecture That Can Be Scaled: The H200 has a scalable architecture that can accommodate multiple GPUs, which enables the concurrent processing of large batches and models. This is necessary for training bigger neural networks more effectively.
- Software Ecosystem Optimization: Nvidia offers a range of software tools and libraries optimized for the H200, such as CUDA and cuDNN. These are designed to fully take advantage of GPU memory capacity while improving performance and streamlining workflow during deep learning project development.
- Increased Data Throughput: The H200 can handle vast volumes of data faster because it has higher data throughput. This eliminates data processing stage bottlenecks, thus reducing the time taken for training and enhancing accuracy in model predictions.
- Accelerated Mixed-Precision Training: The H200 uses both half-precision (16-bit) and single-precision (32-bit) calculations for mixed-precision training. This method speeds up the training process without sacrificing accuracy, thereby saving resources.
These features establish the Nvidia H200 as an important tool for driving deep learning research forward, quickening iteration cycles, and improving model performance across different fields.
Inference Performance and Benefits
The H200 from Nvidia delivers the best inference performance using several benefits:
- Small Latency: This version minimizes latency, which enables quicker response time needed in real-time operations like autonomous driving or financial trading.
- High Throughput: With its enhanced throughput capabilities, the H200 can process many requests for inference simultaneously, making it suitable for deployment at places that require heavy lifting, such as data centers and cloud services. It is equipped with GPU memory enhancements over models released in 2024.
- Power Efficiency: The H200’s architecture design optimizes power consumption during inference operations, reducing operation costs without compromising performance levels.
- Integration Flexibility: The H200 supports popular deep learning frameworks and pre-trained models, making integration into existing workflows seamless and thereby fast-tracking AI solution implementation across different sectors.
These advantages highlight how well Nvidia’s H200 enhances inference tasks, adding value both in research and enterprise-based AI applications.
What Are the Specifications of Nvidia H200 Tensor Core GPUs?
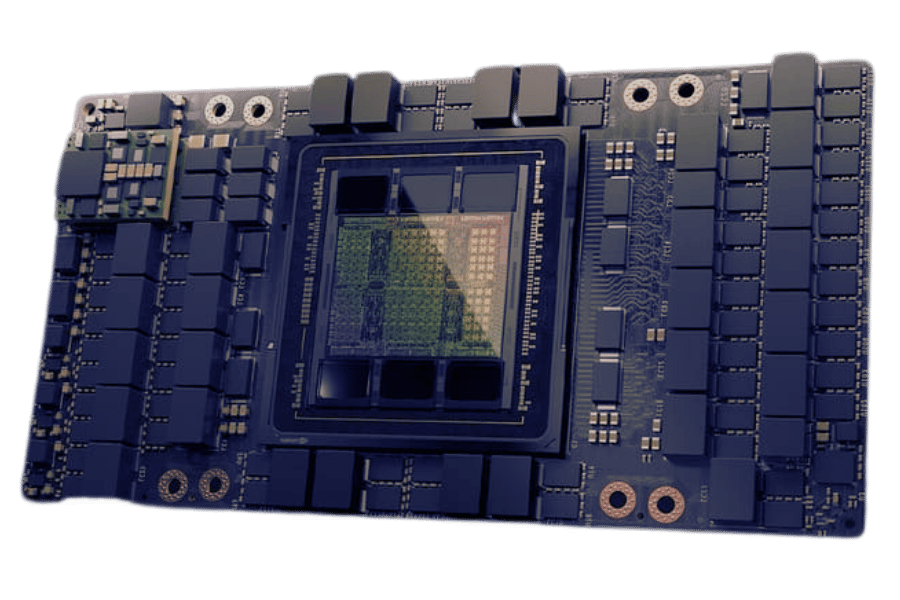
Detailed Specs of H200 Tensor Core GPUs
The H200 Tensor Core GPUs of Nvidia were created with state-of-the-art technology meant to give the best performance in AI and deep learning tasks. Here are some of the most important features:
- Architecture: The H200 is based on Nvidia’s Ampere architecture that has the latest GPU design improvements for strong and efficient AI model processing.
- Tensor Cores: This product has been equipped with third-generation tensor cores; they greatly enhance mixed-precision computing performance and offer twice as much speed as their predecessors.
- CUDA Cores: Each H200 contains more than 7k CUDA cores, thereby ensuring superb computational power for both single-precision and double-precision tasks.
- Memory: It boasts 80GB of high-speed HBM2e memory per GPU, which provides enough bandwidth and capacity for large-scale AI models and datasets.
- NVLink: With NVLink support, there will be higher bandwidth interconnectivity between GPUs, leading to easy multi-GPU configuration and better scalability.
- Peak Performance: For deep learning inference & training, this card delivers up to 500TFLOPS peak fp16, making it one of the most powerful GPUs available today.
- Power Consumption: Power consumption has been optimized so that it operates within a 700W envelope thus balancing between performance and energy use effectively.
These specifications make Nvidia’s H200 tensor core GPUs suitable choices for deploying advanced AI solutions across various sectors, such as data centers or self-driving cars.
Benchmarking the H200
It is important to compare the performance of the Nvidia H200 Tensor Core GPUs with industry standards. Among the benchmarks used to evaluate this graphics card are MLPerf, SPEC, and internal Nvidia performance tests.
- MLPerf Benchmarks: According to MLPerf findings, the H200 is among the fastest devices for AI training and inference workloads. This means that it exhibited great efficiency combined with high speed during tasks such as natural language processing, image classification, or object detection.
- SPEC Benchmarks: The H200’s capabilities for double-precision floating point operations were proven by SPEC GPU computations, where it outperformed other similar products in terms of the computational power needed for this kind of hardware when dealing with scientific calculations or large-scale simulations.
- Internal Nvidia Testing: Single-node and multi-node configurations showed excellent results during both types of benchmarking carried out by NVIDIA internally on their equipment; even multi-GPU setups achieved near-linear scaling due to NVLink supporting high bandwidth low latency communication, which is also enhanced by HGX H100 platforms.
These tests have confirmed that the H200 can be a powerful device for deep learning and AI applications, thus providing an opportunity for companies interested in improving their computational abilities.
AI and HPC Workloads with H200
The most demanding AI and HPC workloads are taken care of by the Nvidia H200 Tensor Core GPU. A notable feature of the H200 is that it enables mixed-precision computing; this means that both FP16 and FP32 operations can be executed seamlessly, leading to shorter training times and reduced computational costs. Furthermore, with NVSwitch and NVLink integrated into it, there is an exceptional interconnect bandwidth that allows efficient scaling across many GPU systems, which is very important in tasks with a lot of data like climate modeling and genomics, among others.
In model training and inference speeds, the H200 significantly improves AI workloads. The underlying reason behind this is its Tensor Core architecture, designed specifically for deep learning frameworks such as TensorFlow and PyTorch, resulting in faster performance during neural network training, reinforcement learning, or even hyperparameter optimization. On the other hand, when making inference, low latency, and high throughput execution are supported by H200, making edge computing deployments possible together with real-time decision-making applications, which include models up to 70B parameters.
For heavy computational tasks and complex simulations in HPC workloads, there are few, if any, equal to the capabilities possessed by the H200. Its advanced memory hierarchy, coupled with large memory bandwidth, ensures that big datasets can be handled efficiently, which is necessary for astrophysics simulations, fluid dynamics, and computational chemistry. Besides, the parallel processing abilities of the h200 open new avenues in areas like quantum computing research and pharmaceutical development, where complicated calculations involving vast volumes of data analysis occur frequently.
To sum up this review on the Nvidia H200 Tensor Core GPU, It offers unmatched performance scalability efficiency over its predecessor(s) while still being efficient enough to run on laptops, too!
How Does the Memory Capacity Impact Nvidia H200 Performance?
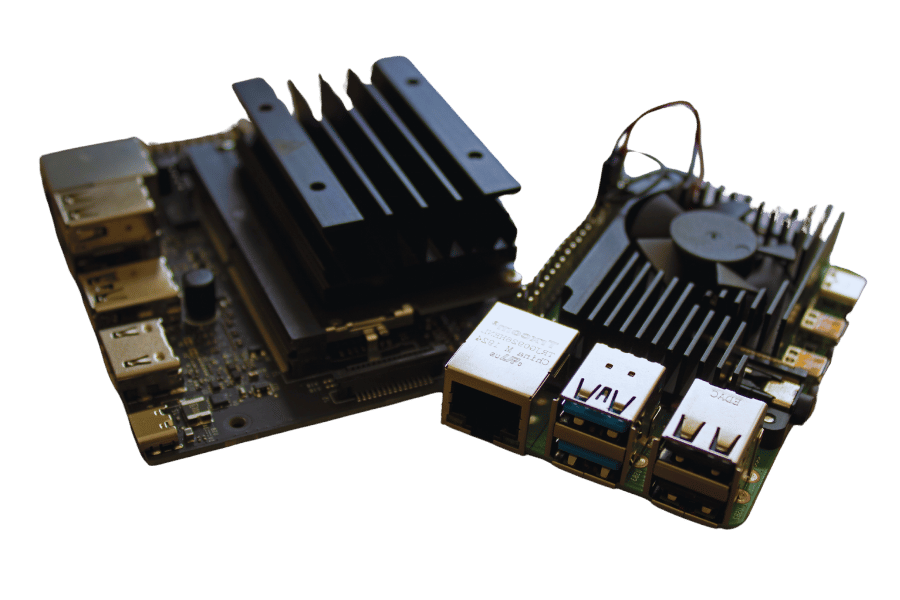
Understanding 141GB HBM3E Memory
The performance of the Nvidia H200 is greatly improved by the 141GB HBM3E memory integrated into it. This is especially so when dealing with large-scale data and computational tasks. High bandwidth Memory Gen 3E, or HBM3E for short, provides much more bandwidth than previous versions, thus delivering speeds necessary for operations requiring a lot of data processing. With bigger storage capacity and higher bandwidth, the GPU can store and work on larger datasets more efficiently, minimizing delays and increasing overall throughput.
In real-world use cases, this enlarged memory capacity enables H200 to maintain peak performance across workloads ranging from AI model training and inference to simulations in high-performance computing (HPC). It should be noted that deep learning heavily relies on manipulating large amounts of data sets, hence benefiting most from this kind of advanced memory architecture while keeping its superiority over Nvidia H100 Tensor Core GPUs in terms of ability to handle complex simulation-involved HPC tasks. This means that the 141GB HBM3E memory allows for better execution of many parallel processes by the H200, leading to quicker computation times and more precise results in various scientific & industrial applications.
Memory Bandwidth and Capacity
No attribute of the Nvidia H200 Tensor Core GPU is more important in terms of overall performance than its memory bandwidth and capacity. The H200 integrates 141GB HBM3E memory, which gives it one of the most impressive memory bandwidths in the world at 3.2 TB/s. High bandwidth is needed to accelerate applications that depend on memory by enabling very fast data transfer rates between storage units and processing devices.
With its significant bandwidth, this vast amount of memory allows Nvidia’s latest offering to process large datasets efficiently, a necessary requirement for AI training and high-performance computing simulations, among others. This helps keep a lot of information close at hand so that there is little need to swap frequently used data out, thereby lowering latency. Additionally, it supports parallel processing through HBM3E architecture, thereby making computations more efficient while speeding up data manipulation.
In short, the Nvidia H200 has great features like big memory size and fast-speed channels; these two combined together greatly enhance its ability to quickly perform complex mathematical operations necessary for artificial intelligence development or any other demanding calculations typical within this field.
Performance in Data Center Environments
The Nvidia H200 Tensor Core GPU is designed to meet the demands of modern data center environments. The advanced processing power of this product makes it possible to perform multiple diverse tasks efficiently. These functions include artificial intelligence, machine learning, and high-performance computing (HPC) applications. Parallel processing features in data centers are optimized by H200, which ensures that computations are done faster and resources are managed better. In addition, with a memory bandwidth of up to 3.2 TB/s, data can be transferred quickly, thus reducing bottlenecks and enabling quicker execution of complex algorithms. Moreover, the muscular structure of H200 allows for easy scaling up without any impact on performance levels so that more computational abilities may be added when needed without sacrificing speed or reliability. Essentially, if you want your data center operation at peak efficiency, then this is the perfect device for you!
What is the Role of Nvidia DGX in Harnessing the Power of H200?
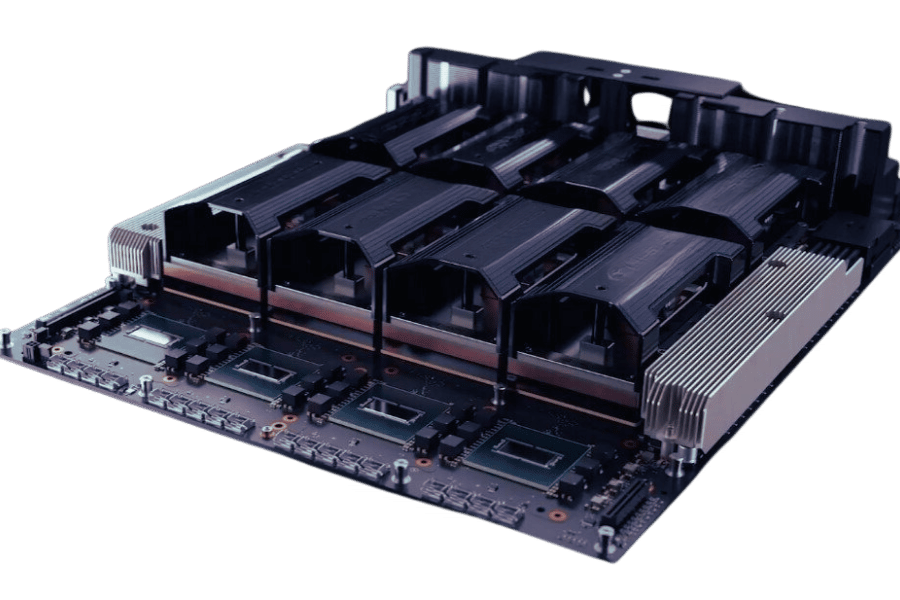
Nvidia DGX H200 Systems
The Nvidia DGX H200 Systems are designed for the H200 Tensor Core GPU. These systems are made to provide extraordinary AI and HPC performance by integrating many H200 GPUs with each other using high-speed NVLink interconnects as the HGX H100 setups work. The DGX H200 system is very scalable and efficient, which lets data centers achieve quicker insights and innovations than ever before possible. The software stack that comes with the system, created by Nvidia and optimized for AI as well as data analytics, among others, ensures this happens in addition to ensuring that organizations can make the best use of what these cards offer them. Such features include DGX Station A100 for smaller scales or DGX SuperPOD when working on larger scale deployments; all these make sure that there is no limit to computational power needed by any user at any level.
DGX H200 for Large Language Models (LLMs)
The reason Large Language Models (LLMs) are considered the most advanced form of artificial intelligence technology is their capacity to comprehend and generate human-like text. The DGX H200 system is designed to implement LLMs in training and deployment operations. In order to process the massive datasets that LLMs need, the DGX H200 has multiple H200 GPUs integrated into it and high-speed NVLink interconnects incorporated within them, thereby providing high throughput as well as low latency. Additionally, Nvidia’s software stack, which includes frameworks such as TensorFlow and PyTorch, has been optimized to take advantage of parallel processing capabilities offered by these types of GPUs, resulting in faster training times for LLMs. Therefore, if you are an organization seeking to build cutting-edge language models quickly, then this would be your best bet!
High-Performance Compute with Nvidia DGX H200
The Nvidia DGX H200 is designed to handle the most computationally demanding tasks across various domains, including scientific research, financial modeling, and engineering simulations. It achieves this using several H200 GPUs connected with NVLink that provide the best interconnect bandwidth and minimal latency. Moreover, their software suite for high-performance computing (HPC) includes CUDA, cuDNN, and NCCL, among others, which are very helpful in achieving breakthroughs faster than ever before possible in such areas. For this reason alone, but also due to its flexible nature and scalability properties, it makes perfect sense why DGX H200 would be recommended for any establishment looking forward to venturing far beyond what has been accomplished through computational sciences.
Reference Sources
Frequently Asked Questions (FAQs)
Q: What is the Nvidia H200 and how does it differ from the Nvidia H100?
A: The Nvidia H200 is an advanced GPU that builds upon the foundation laid by the Nvidia H100. It features improved computational power, memory capacity, and bandwidth. The first GPU with integrated HBM3e memory is called the H200, which has much faster memory speeds than the H100. Increased efficiency in processing large amounts of data for AI-intensive workloads was thoughtfully developed.
Q: What are the key features of the Nvidia H200 Tensor Core GPU?
A: The Nvidia H200 Tensor Core GPU features the latest Nvidia Hopper architecture, 141GB of HBM3e memory, and a TDP of 700W. FP8 precision is supported, improving AI model training and inference efficiency. It’s optimized specifically for large language models (LLMs), scientific computing, and AI workloads.
Q: How does the H200 architecture enhance AI computation and generative AI capabilities?
A: The H200’s architecture builds on top of the NVIDIA hopper, introducing better tensor cores and high-speed memories, which allow more efficient computation when doing things like training inference or generative AI models such as ChatGPTs. What OpenAI did with ChatGPT scaled using increased compute capability, but this required additional storage because there were many more parameters.
Q: What makes the Nvidia H200 suitable for scientific computing and AI inference?
A: FP16 and Fp8 precision are needed for accurate and efficient scientific computations and AI inference. The high amount of memory also helps ensure that large data sets can be processed quickly, so it’s best suited for these tasks.
Q: How does the memory capacity and bandwidth of the Nvidia H200 compare to previous models?
A: Compared to older models such as its predecessor, the Nvidia H100, the Hbm3e-based Nvidia H200 has a memory capacity of 141GB while providing larger bandwidth and faster speeds that enable rapid access to data during computation needed for large-scale AI or scientific tasks.
Q: What is the relevance of HBM3e memory in the H200 GPU?
A: It delivers faster speed and greater bandwidth compared to earlier versions of HBMs. This allows the chip to handle huge AI models and high-performance computing workloads that no other GPU has ever done, making it the first scalable GPU with such capabilities. Hence, this makes it extremely useful in environments that require quick data processing and high memory performance.
Q: What are some advantages of utilizing the Nvidia HGX H200 system?
A: The Nvidia HGX H200 system uses many H200 GPUs together to create a powerful AI platform for scientific computing. With this comes lots of computing power offered by Tensor Core GPUs built into each one, thus allowing large organizations to run complex tasks efficiently on single-node clusters while saving costs associated with scaling out multiple nodes across racks or even buildings.
Q: How does Nvidia’s computational power impact AI model training and inference?
A: Enhanced precision capabilities like FP8 combined with advanced tensor cores greatly accelerate AI model training and inference, but only when supported by hardware such as those found on Nvidia’s newest chip, the H200. This means that developers can build larger deep learning models in less time than ever, thereby accelerating both research development cycles and deployment speed, especially for developers working at edge locations with limited connectivity backhaul capacity.
Q: What new things does Nvidia H200 bring for generative AI and LLMs?
A: The increased computational power, memory capacity, bandwidth, etc., provided by NVIDIA h 200 enable better handling of bigger model datasets, hence allowing more efficient training deployment of complex systems used by enterprises to create advanced artificial intelligence applications.
Q: Why is the Nvidia H200 considered a breakthrough in GPU technology?
A: Nvidia h200’s use of high-level precision computation support through hbm3e memory adoption alongside hopper architecture advancement makes it outstanding among other GPUs. This sets new standards for AI and scientific computing due to the improved performance efficiency and capability offered by these inventions.
Related Products:
-
NVIDIA MMA4Z00-NS400 Compatible 400G OSFP SR4 Flat Top PAM4 850nm 30m on OM3/50m on OM4 MTP/MPO-12 Multimode FEC Optical Transceiver Module $900.00
-
NVIDIA MMA4Z00-NS-FLT Compatible 800Gb/s Twin-port OSFP 2x400G SR8 PAM4 850nm 100m DOM Dual MPO-12 MMF Optical Transceiver Module $1200.00
-
NVIDIA MMA4Z00-NS Compatible 800Gb/s Twin-port OSFP 2x400G SR8 PAM4 850nm 100m DOM Dual MPO-12 MMF Optical Transceiver Module $1200.00
-
NVIDIA MMS4X00-NM Compatible 800Gb/s Twin-port OSFP 2x400G PAM4 1310nm 500m DOM Dual MTP/MPO-12 SMF Optical Transceiver Module $1550.00
-
NVIDIA MMS4X00-NM-FLT Compatible 800G Twin-port OSFP 2x400G Flat Top PAM4 1310nm 500m DOM Dual MTP/MPO-12 SMF Optical Transceiver Module $1550.00
-
NVIDIA MMS4X00-NS400 Compatible 400G OSFP DR4 Flat Top PAM4 1310nm MTP/MPO-12 500m SMF FEC Optical Transceiver Module $1350.00
-
Mellanox MMA1T00-HS Compatible 200G Infiniband HDR QSFP56 SR4 850nm 100m MPO-12 APC OM3/OM4 FEC PAM4 Optical Transceiver Module $300.00
-
NVIDIA MFP7E10-N010 Compatible 10m (33ft) 8 Fibers Low Insertion Loss Female to Female MPO Trunk Cable Polarity B APC to APC LSZH Multimode OM3 50/125 $47.00
-
NVIDIA MCP7Y00-N003-FLT Compatible 3m (10ft) 800G Twin-port OSFP to 2x400G Flat Top OSFP InfiniBand NDR Breakout DAC $495.00
-
NVIDIA MCP7Y70-H002 Compatible 2m (7ft) 400G Twin-port 2x200G OSFP to 4x100G QSFP56 Passive Breakout Direct Attach Copper Cable $250.00
-
NVIDIA MCA4J80-N003-FTF Compatible 3m (10ft) 800G Twin-port 2x400G OSFP to 2x400G OSFP InfiniBand NDR Active Copper Cable, Flat top on one end and Finned top on other $600.00
-
NVIDIA MCP7Y10-N002 Compatible 2m (7ft) 800G InfiniBand NDR Twin-port OSFP to 2x400G QSFP112 Breakout DAC $680.00