The requirement for high-performing computing solutions is at an all-time high, particularly in artificial intelligence and deep learning. NVIDIA DGX is the number one option for any organization, data scientist, or researcher who wants to maximize computation power and efficiency in this ever-changing world of technology. This article will elaborate more about NVIDIA DGX systems by exploring their capabilities, architecture design, and how they can forever change AI and deep learning workflows. It will also look into their specifications and practical applications, thus giving readers a broader knowledge base on what sets these cutting-edge computers apart from others in the high-performance computing field, according to NVIDIA DGX.
What is an NVIDIA DGX™ System?
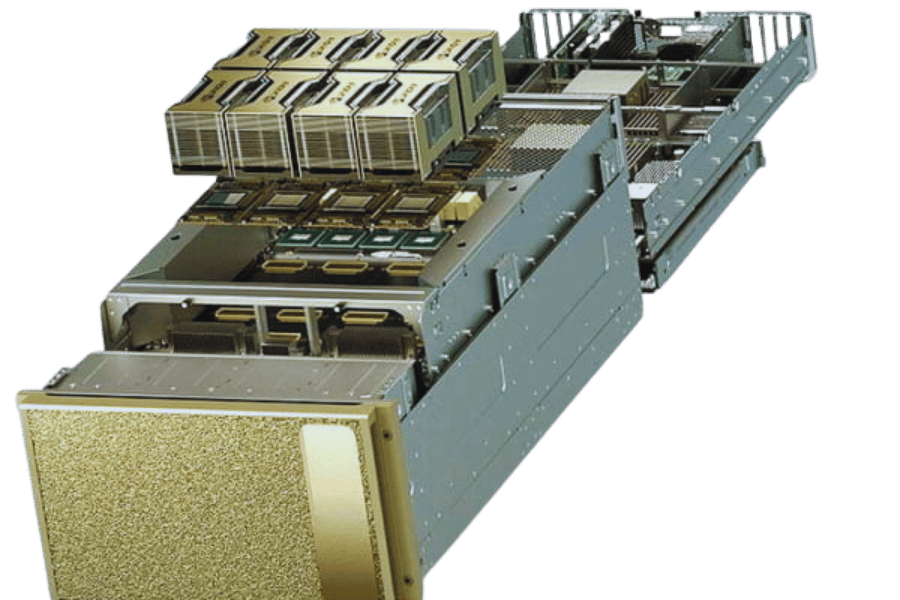
Understanding NVIDIA DGX™
An NVIDIA DGX system is a platform created to accelerate AI and deep learning workloads. This is achieved by embedding advanced software and hardware architectures alongside state-of-the-art GPUs from NVIDIA. They come with an already installed NVIDIA DGX software stack which has optimized versions of various deep learning frameworks for easy deployment and utmost productivity. For example, using such platforms as DGX SuperPOD can cut down model training duration by a great margin, thus allowing companies to discover ideas more quickly and implement creative solutions.
Key Features of NVIDIA DGX™ Systems
DGX™ systems are identified by their advanced features and performance capabilities which make them perfect for AI and deep learning workloads. These comprise:
- NVIDIA Tesla GPUs: NVIDIA Tesla GPUs form the core of all DGX™ systems; they have been designed to carry out parallel processing more efficiently thus providing a computational foundation for complex artificial intelligence tasks that require high-performance computing (HPC) power such as those found in DGX-1 and DGX-2.
- NVLink Interconnect: The NVLink technology by NVIDIA creates connections with bigger bandwidths among GPUs leading to much faster data transfer rates while also ensuring that workload-intensive computations receive the best performance possible.
- DGX™ Software Stack: This software stack comes integrated with optimized versions of well-known deep learning frameworks, tools for monitoring performance during execution as well as support for containerized deployment among others thus enabling seamless integration into existing environments thereby maximizing efficiency gains across different areas.
Together, these features enable businesses to achieve unheard-of levels of performance in their AI projects, cutting down time to find solutions and sparking innovation.
Comparing Different DGX Systems
To ensure the best performance and scalability for AI and deep learning projects, organizations should compare different NVIDIA DGX™ systems in terms of their specific use cases, computational power, and scalability options. The main machines in this series are the DGX Station™ A100, DGX A100, and DGX-2™.
- DGX Station™ A100: This is an AI-applied system designed for group work environments that utilizes a maximum of four NVIDIA A100 Tensor Core GPUs. It is most suitable for teams doing AI research and development outside the data center as it balances power with portability.
- DGX A100: This computer is more powerful than the previous one and mostly used in data centers where it can be deployed because it supports up to eight NVIDIA A100 GPUs that are interconnected through NVLink to achieve maximum performance. It has diverse workloads ranging from training large-scale models to complex data analytics, which makes it ideal for enterprise-level applications.
- DGX-2™: Among the three products listed here, this is the strongest system under DGX lineup thanks to its sixteen Nvidia V100 Tensor Core GPUs coupled with NVSwitch technology. The setup maximizes computational resources for intensive artificial intelligence model training while also offering great efficiency per unit of time spent on high-performance computing tasks.
These flagship machines enable companies to choose top-of-the-line hardware solutions that will most effectively meet their needs, thus providing them with the necessary tools for artificial intelligence development at large.
How is NVIDIA DGX™ Used in AI and Deep Learning?
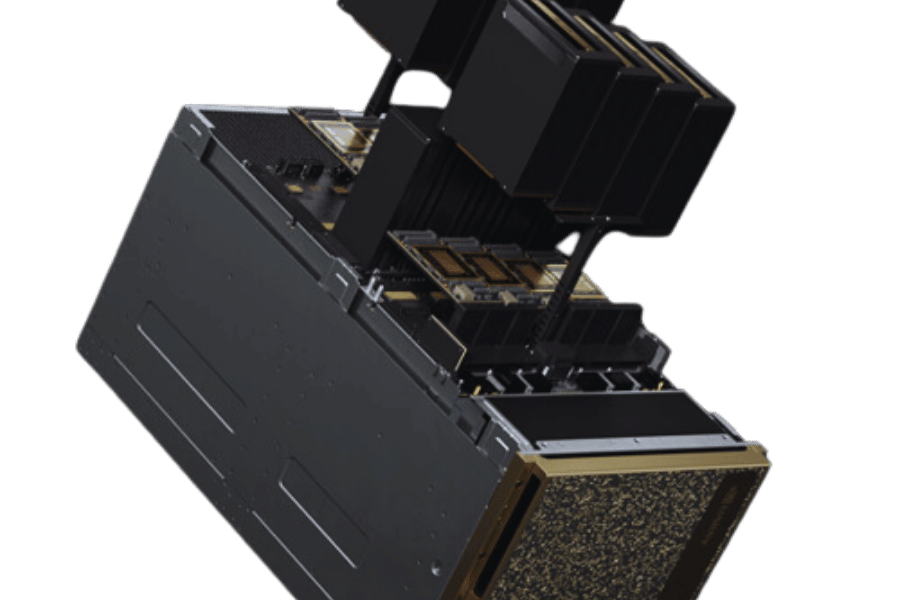
AI Compute with NVIDIA DGX™
NVIDIA DGX™ systems are incredibly important for AI and deep learning frameworks as they provide unmatched computational power and scalability. They can be used in a wide range of applications, including training complex AI models, carrying out advanced data analytics, and developing neural networks and machine learning algorithms. DGX systems are equipped with high-density GPU configurations like NVIDIA A100 and V100 Tensor Core GPUs that enhance processing capabilities to handle large datasets and enable real-time inference. As a result, organizations use NVIDIA DGX™ to foster innovation in autonomous driving, healthcare diagnostics, and financial modeling, among others, while providing cutting-edge solutions to global problems.
Deep Learning Applications on DGX Systems
For a lot of deep learning applications, NVIDIA DGX™ systems are absolutely necessary because they provide enough computing power to handle intense tasks. Here are some main uses:
- Image and Video Processing: Rapid processing and real-time analytics powered by DGX systems that leverage GPUs for object detection, image classification, and video segmentation are essential for media, surveillance, and autonomous vehicles.
- Natural Language Processing (NLP): DGX systems can train language models on a large scale which is useful for machine translation among other applications like sentiment analysis or conversational AI tools that improve customer service and content recommendation abilities.
- Healthcare and Biomedical Research: Diagnostic tools development speeds up along with personalized medicine or drug discovery thanks to computational capabilities of DGX systems including NVIDIA DGX A100; these also allow faster and more accurate predictions/analyses due to dealing with huge volumes of medical data.
- Financial Services: In finance, people use DGSs mainly for fraud detection but also for algorithmic trading or risk assessment since they can process massive amounts of data while making decisions in real-time is crucial for staying competitive.
- Robotics and Autonomous Systems: Different industries would greatly benefit from higher automation efficiency brought about by operationalizing robots trained through models on DGX systems; this would equally advance navigation across various industries helped by autonomy in functioning
Benefits of NVIDIA DGX™ for AI Infrastructure
The aim of this article is to rewrite the given text in such a way that it becomes very dynamic, extremely perplexing and full of synonyms while retaining its meaning. It should also have a creative, varied sentence structure. The length of your output should be more or less equal to that of the input. Ensure you follow these rules strictly.
- High speed: DGX™ systems use NVIDIA GPUs with high performance which ensures quicker training and inference times for complex AI models. This makes it possible for organizations to work with huge amounts of data sets and perform computations at unprecedented speeds.
- Flexible: NVIDIA DGX™ systems are designed in such a manner that they can scale easily, thus enabling businesses to grow their artificial intelligence capacities with time. This means that companies can create strong and efficient AI infrastructure depending on what they need by clustering several DGX systems together.
- Optimized software stack: DGX™ systems come installed with NVIDIA’s optimized software stack which includes frameworks like TensorFlow, PyTorch among others. This integration allows model developers not to bother about managing infrastructures but rather concentrate on developing models.
- Supports enterprises: NVIDIA supports enterprises in various ways. For instance, technical help is provided on DGX™ systems such as NVIDIA DGX-1 and NVIDIA DGX-2. Regular software updates and performance optimizations are also carried out so that AI infrastructure within an organization is reliable and available.
- Increased productivity: Data scientists and researchers can quickly iterate when using powerful computing environments provided by DGX™ systems, thereby leading to faster deployment across different application areas and accelerating invention around AI solutions.
To sum up, what I can say is that no other foundation can deliver performance combinations like scalability optimization support like those brought about by NVidia dgx systems.
What are the Benefits of NVIDIA DGX™ Systems?
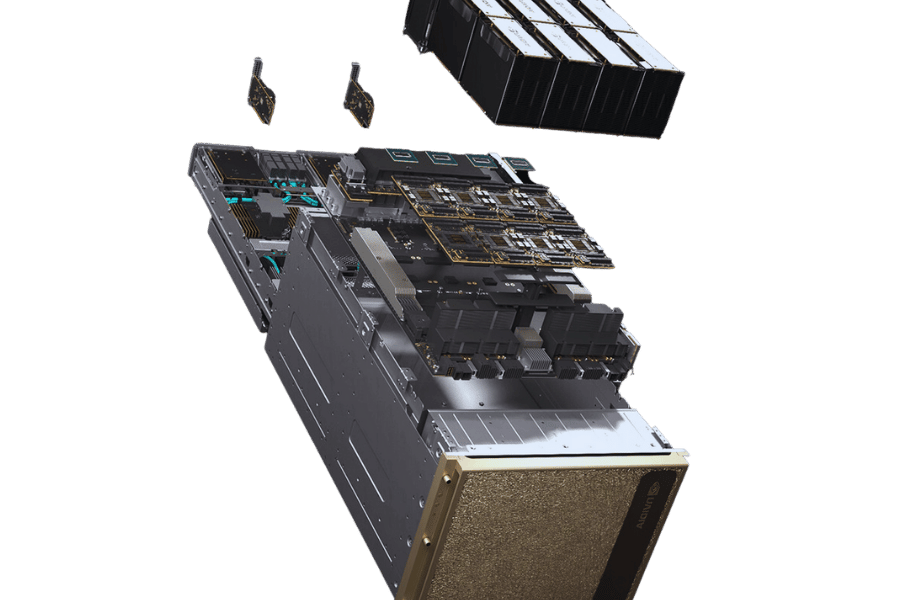
Enhanced Compute Capabilities
NVIDIA DGX systems exploit NVIDIA GPUs to offer unmatched computing power required for AI and deep learning tasks. These are built to handle heavy computation loads with ease, using GPU acceleration that speeds up processes that would otherwise take long hours using conventional CPUs. Every DGX system is packed with numerous powerful GPUs that work together in executing intricate models as well as data analysis at an incredible speed. Such hardware and software optimization has made these systems an essential tool for any organization looking forward to advancing its AI research capabilities. This enables enterprises to achieve quicker realization of ideas and stay ahead in the dynamic world of artificial intelligence.
Streamlined AI Development
NVIDIA DGX™ systems are great for AI development because of their many tools and software. For instance, NVIDIA AI software stack is one such tool that comes pre-installed on these computers; it includes frameworks necessary for deep learning and other artificial intelligence workflows like cuDNN, TensorRT™ among others which utilize NVIDIA CUDA®. Researchers usually spend a lot of time setting up models. Still, with DGX™’s integrated approach, this process can be skipped altogether, thus allowing them to concentrate more on training models for optimal performance. Furthermore, DGX™ supports container-based workflow through NGC by NVIDIA – a catalog containing GPU-optimized containers designed to simplify deployment while improving portability at the same time. This environment also makes artificial intelligence development less complex than before hence more productive and innovative across different projects in AI.
Scalability for Enterprise AI Solutions
NVIDIA DGX™ systems are scalable and suitable for business artificial intelligence solutions. These systems allow for easy expansion from setups consisting of single-nodes to those that involve multi-node clusters thus enabling firms to cope with increased workloads without compromising on performance. For example, DGX™ SuperPOD™ can combine many DGX™ systems into one powerful AI supercomputer. This step-based method guarantees effective infrastructure scaling as data volume and computational requirements increase. In addition, strong interconnect technologies like NVIDIA NVLink are used, which aids in fast communication between GPUs, hence preventing any scaling bottlenecks. Whether it is utilized for large-scale environments’ research, development, or AI deployment purposes, NVIDIA DGX™ systems have the needed power and flexibility to handle enterprise-level demands.
How do you deploy and manage a DGX system?
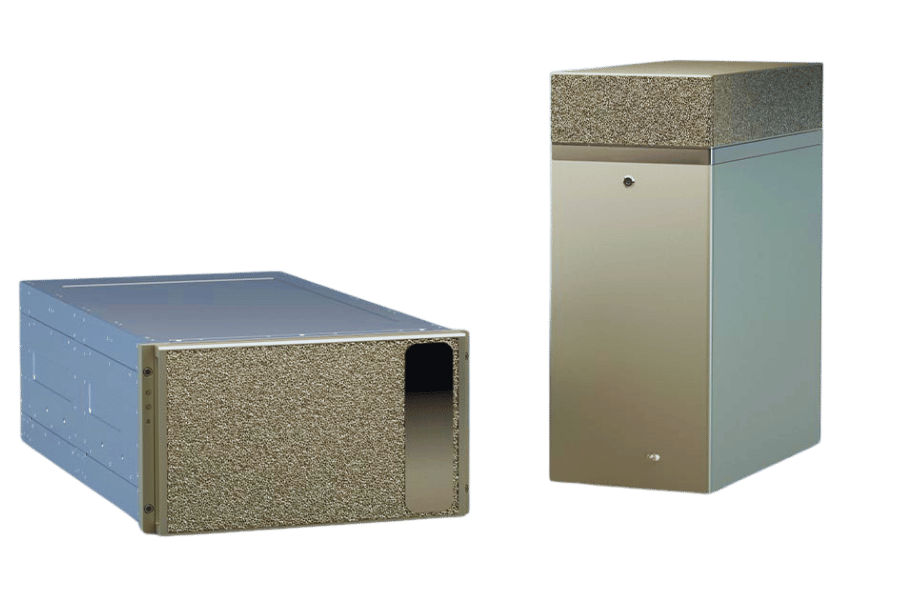
Setting Up Your DGX Station
Certain fundamental steps need to be taken to ensure that DGX Station is working as it should. Firstly, one should put the DGX station in a well-ventilated and stable place so that it can cool down properly without gathering dust. Supply the power and connect the station to an uninterruptible power supply (UPS) to avoid losing data during blackouts. After that, connect your DGX station with other devices using high-speed Ethernet for quick communication, as well as facilitating fast data transfer through a network.
Once you are done with the physical setup, turn on the DGX Station and follow the prompts displayed on its screen until you access an operating system. You must install up-to-date NVIDIA GPU drivers along with the CUDA Toolkit since they are vital for enabling GPU-accelerated applications to run. NVIDIA’s deep learning software stack may be used for configuring software environments whereby one can utilize Docker containers pre-installed from the NVIDIA NGC catalog, thus simplifying the deployment process of TensorFlow, among other deep learning frameworks like PyTorch.
For proper management and monitoring purposes, one can use the Nvidia-semi command line interface, which is part of the NVIDIA System Management Interface package, so as to keep track of their GPUs’ performance; this also includes usage percentages relative temperature values recorded by those graphic cards while being operated at any given time or period. Moreover, setting secure protocols like SSH enables remote access into DGX Station from different machines, hence allowing users to manage their AI workloads flexibly wherever they are located around the world. By following these instructions, you will have set yourself up for success when trying out various capabilities supported by your DGX Station efficiently.
Managing DGX Systems in Data Centers
There are many steps to manage DGX systems in data centers for optimal performance and smooth operations. First, ensure that you place them correctly in a rack for efficient cooling; leave enough space around each unit for air circulation. Power management is critical, so connect these systems to redundant power supplies and keep tabs on power consumption to prevent overloading. Networking should be configured with high-speed, low-latency connections – preferably InfiniBand or any other similar technology that allows fast exchange of data between machines. Regular software updates, including the most recent drivers and firmware versions, need to be installed so that they remain compatible with one another and work efficiently too. Use centralized management tools such as Kubernetes or NVIDIA GPU Cloud (NGC) for orchestration at scale, among others. Robust monitoring can be done by tracking GPU health through NVIDIA Nvidia-semi and DCGM, which also give performance metrics plus point out possible problems. Finally, secure access protocols, together with regular backups, will protect AI works while facilitating smooth operation within the context of a data center setup.
Software and Tools for DGX Management
To manage DGX systems well, there must be a combination of particular software and tools whose aim is to increase efficiency and simplify operations. An example of such software is NVIDIA AI Enterprise. Below are some vital examples of software and tools:
- NVIDIA GPU Cloud (NGC): This is an all-inclusive package that contains GPU-optimized AI, data analytics, and HPC, among other types of software used for applications’ deployment as well as management in most cases employed by DGX SuperPOD deployments.
- Kubernetes is an open-source platform designed for automation purposes during application container scaling, deployment, and operation across clusters of hosts. It offers strong solutions used in AI workload orchestration, often deployed with DGX SuperPOD.
- NVIDIA Nvidia-semi: A command-line utility that provides monitoring capabilities plus management functions meant for NVIDIA GPU devices. It allows tracking performance metrics and GPU health on a real-time basis.
- NVIDIA Data Center GPU Manager (DCGM): This tool monitors and manages GPU resources within data center environments, thereby giving insights into issues related to utilization levels or any potential problem that may arise concerning the health status of different GPUs.
By implementing these tools, administrators can ensure effective resource use, keep the system healthy, and achieve smooth orchestration of AI workloads.
Which NVIDIA DGX™ System is Right for You?
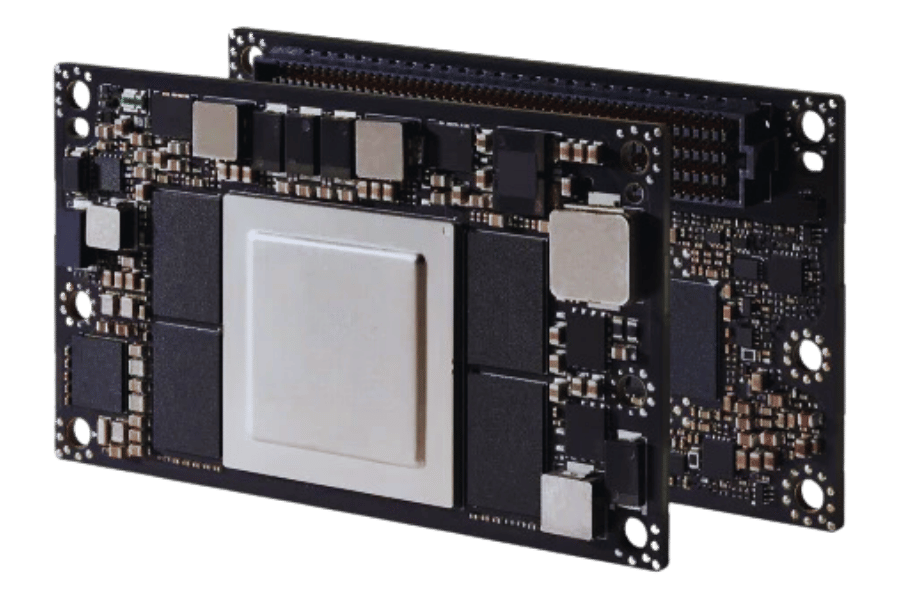
Comparing DGX A100 vs. DGX Station A100
After looking into DGX A100 and DGX Station A100, I have found that both are high-performing but differ in the user’s case. DGX A100 is a system meant for data centers with scalability like no other and flexibility as its core feature. It can handle up to eight A100 Tensor Core GPUs,, making it perfect for complicated AI workloads needing huge amounts of computing power alongside parallel processing abilities in HPC.
On the other hand, DGX Station A100 was created with small office environments in mind, thus making this option more accessible to research teams and smaller workgroups. It has four A100 GPUs and acts as a ‘workgroup server’, meaning it can deliver data center performance without required dedicated IT infrastructure. Also, whisper-quiet operation comes standard on every DGX Station A100 model, so even plugging directly into any regular power outlet will be enough for them to run – such convenience may prove invaluable, especially where space resources are limited within an office setting.
Ultimately, whether one goes for DGX or Station depends entirely upon their needs: either go all out on scalability and deploy in data centers using DGXs; alternatively, opt for near-silent office friendly powerful computing capability with stations.
Choosing Between DGX Workstation and DGX Server
While selecting a DGX Server and a DGX Workstation, it’s necessary to think about the intended use as well as where it will be used. Basically, individual scientists or small teams who need strong AI and machine learning development abilities should go for DGX Workstation. It is packed with large computation resources that can fit into desktops or small offices to ensure silence and ease of use without much IT infrastructure.
On the other hand, DGX Server is designed for use in enterprise data centers on a larger scale deployment. With increased redundancy, higher scalability support as well as advanced management capabilities; this hardware becomes perfect for processing huge amounts of data when dealing with massive AI workloads. This means that DGX Servers can be easily integrated into existing environments of any data center, hence ensuring continuous operation coupled with maintenance supported by powerful performance delivered through such infrastructures.
In conclusion, if you want a collaborative workspace with high-performance requirements but limited space and facilities, then go with a DGX workstation while considering your options carefully; but if you are looking at large-scale enterprise-level AI applications that require robustness in terms of scalability and infrastructure, then a DGX server would be more suitable for you.
Evaluating DGX Systems for Specific AI Use Cases
Consider various factors while evaluating DGX systems for particular AI use cases to ensure optimal performance and resource usage. For example, both DGX Workstations and DGX Servers come with cutting-edge GPUs that can speed up data-intensive artificial intelligence applications such as natural language processing (NLP) or deep learning model training. However, this will depend on the size and scope of your project.
The compact size together with reduced noise production makes DGX Workstation ideal for individual researchers or small teams collaborating on projects with limited resources such as prototyping stages through model development up until initial deployment phase where only few computation powers are required in comparison to large scale AI applications involving massive amounts of data processing coupled with high throughput needs which calls for scalability they offer as well as robust infrastructure provided by enhanced management tools within DGX server making it more suitable than any other option available when dealing with enterprise level artificial intelligence systems running continuously at data centers requiring seamless integration into established IT ecosystems supported by NVIDIA AI Enterprise but not only should you pick between these two models based on what your organization does need for its operational environment and infrastructural capabilities to deliver against specific demands of different Artificial Intelligence use cases while considering computational requirements vis-a-vis overhead costs involved?
Reference Sources
Frequently Asked Questions (FAQs)
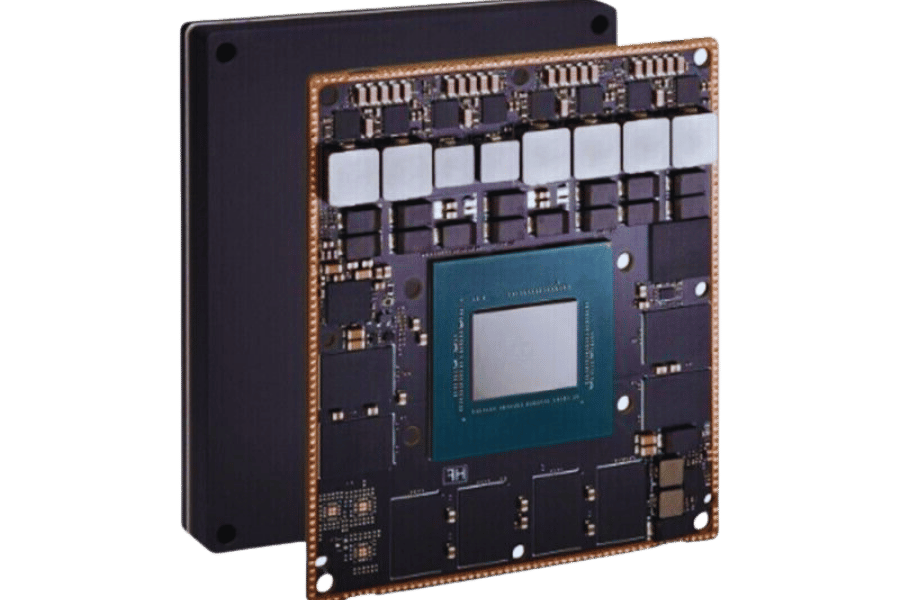
Q: What are NVIDIA DGX™ Systems?
A: The NVIDIA DGX™ are specially designed deep learning systems that provide the computing power needed for creating and deploying artificial intelligence (AI) and deep learning models. These systems use high-performance NVIDIA GPUs and advanced software stacks optimized by NVIDIA to handle complicated calculations.
Q: How are NVIDIA DGX Systems different from other solutions?
A: NVIDIA DGX Systems combine the most powerful GPUs, including Tensor Core technology, with their hardware and the NVIDIA GPU Cloud (NGC) software stack. This combination ensures record-breaking performance, scalability, and efficiency when handling AI and deep learning tasks, allowing data scientists or researchers to construct better AI models faster.
Q: Could you explain about the NVIDIA DGX H100?
A: The Nvidia dgx h100 is a model inside the Dgx family created for optimized execution of deep learning workloads within an AI environment. It is also part of enterprise solutions. It consists of multiple Nvidia GPUs, a high-speed interconnect like a link from Nvidia, and robust memory bandwidth, thus making it appropriate for heavy machine learning and analytics applications.
Q: What is the NVIDIA DGX Station A100 used for?
A: Data scientists, researchers, and engineers use a workstation-class system called Nvidia DGX Station a100 to develop & test AI models. This form factor brings Nvidia’s ai technology closer than ever before, allowing high-performance computing at desk level
Q: How do NVIDIA GPUs enhance the performance of DGX Systems?
A: The latest tesla Tesla-based Nvidia GPUs equipped with tensor cores have an enormous parallel processing power required for deep learning and AI workloads done by DGX-2 servers — this is one more reason why those machines need them so much! Their ability to handle huge computations alongside complex neural networks speeds up model training and accelerates inference processes, too
Q: What does the NVIDIA GPU Cloud (NGC) do in DGX Systems?
A: This cloud platform provides easy access to NVIDIA’s latest tools and resources, including AI frameworks, pre-trained models, and HPC applications optimized for running on NVIDIA DGX systems. In addition to accelerating AI development, NGC also simplifies deployment processes by allowing software packages compatible with DGX hardware to be downloaded directly from its repository.
Q: How do data scientists benefit from using NVIDIA DGX Solutions?
A: Faster model training times, improved inference performance, and better productivity are some of the benefits data scientists get when using NVIDIA DGX solutions. These systems free them from dealing with hardware limitations and configuration issues; hence, they can focus more on developing innovative AI models.
Q: What does the term “NVIDIA DGX SuperPOD™” mean?
A: “NVIDIA DGX SuperPOD™” refers to a scalable AI infrastructure solution designed by Nvidia that combines several DGX systems into one cluster that acts like a high-performance computer. This computing environment supports large-scale AI model training and analytics, making it suitable for organizations or research institutions needing massive computational power.
Q: What role does the NVIDIA Deep Learning Institute play within the overall context of the NVIDIA DGX ecosystem?
A: Users will be able to maximize their potential in utilizing AI projects while using their NVIDIA DGX Systems after getting trained through gaining knowledge at this institute, where developers, data scientists, and researchers are taught how best they can apply deep learning technologies provided by Nvidia corporation effectively during different stages involved in doing artificial intelligence related work such as training models or even creating new ones.
Related Products:
-
NVIDIA MMA4Z00-NS400 Compatible 400G OSFP SR4 Flat Top PAM4 850nm 30m on OM3/50m on OM4 MTP/MPO-12 Multimode FEC Optical Transceiver Module $650.00
-
NVIDIA MMA4Z00-NS-FLT Compatible 800Gb/s Twin-port OSFP 2x400G SR8 PAM4 850nm 100m DOM Dual MPO-12 MMF Optical Transceiver Module $850.00
-
NVIDIA MMA4Z00-NS Compatible 800Gb/s Twin-port OSFP 2x400G SR8 PAM4 850nm 100m DOM Dual MPO-12 MMF Optical Transceiver Module $750.00
-
NVIDIA MMS4X00-NM Compatible 800Gb/s Twin-port OSFP 2x400G PAM4 1310nm 500m DOM Dual MTP/MPO-12 SMF Optical Transceiver Module $1100.00
-
NVIDIA MMS4X00-NM-FLT Compatible 800G Twin-port OSFP 2x400G Flat Top PAM4 1310nm 500m DOM Dual MTP/MPO-12 SMF Optical Transceiver Module $1200.00
-
NVIDIA MMS4X00-NS400 Compatible 400G OSFP DR4 Flat Top PAM4 1310nm MTP/MPO-12 500m SMF FEC Optical Transceiver Module $800.00
-
Mellanox MMA1T00-HS Compatible 200G Infiniband HDR QSFP56 SR4 850nm 100m MPO-12 APC OM3/OM4 FEC PAM4 Optical Transceiver Module $200.00
-
NVIDIA MFP7E10-N010 Compatible 10m (33ft) 8 Fibers Low Insertion Loss Female to Female MPO Trunk Cable Polarity B APC to APC LSZH Multimode OM3 50/125 $47.00
-
NVIDIA MCP7Y00-N003-FLT Compatible 3m (10ft) 800G Twin-port OSFP to 2x400G Flat Top OSFP InfiniBand NDR Breakout DAC $275.00
-
NVIDIA MCP7Y70-H002 Compatible 2m (7ft) 400G Twin-port 2x200G OSFP to 4x100G QSFP56 Passive Breakout Direct Attach Copper Cable $155.00
-
NVIDIA MCA4J80-N003-FTF Compatible 3m (10ft) 800G Twin-port 2x400G OSFP to 2x400G OSFP InfiniBand NDR Active Copper Cable, Flat top on one end and Finned top on other $600.00
-
NVIDIA MCP7Y10-N002 Compatible 2m (7ft) 800G InfiniBand NDR Twin-port OSFP to 2x400G QSFP112 Breakout DAC $200.00