The Nvidia GeForce RTX 4090 and the Nvidia A100, in an endless search for improvement in computer technology, are the most advanced graphics processing units that have ever been made. These two technological giants, though they spring from similar roots of invention, have different purposes of existence. In this article, I will dissect the technical prowess and realms of application for each GPU. This will be done by analytically comparing both GPUs to enable enthusiasts, professionals, and players to make an informed decision. On the one hand, we have the gaming-focused RTX 4090, while on the other hand, there is the data-centric A100, which helps illuminate any specialized architectures as well as performance paradigms that define modern-day GPU technologies. Let us, therefore, delve into these subtle differences that make them what they actually are in terms of their effect on gaming, AI, and HPC ecosystems.
Primary Details: Understanding the Giants
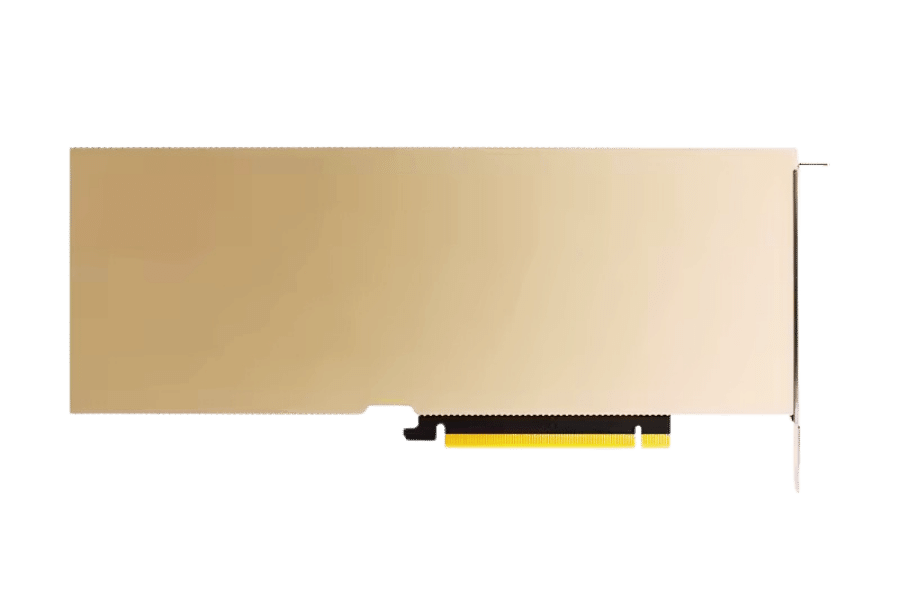
Nvidia RTX 4090: A Glimpse into the Future of Gaming and AI
The Nvidia RTX 4090, a brand-new graphics card with Ampere architecture, represents the next big step in gaming and AI technology. Primarily, it is a gaming device that can handle, for example, 4k of mid-range ray tracing at high fps quite easily. Additionally, the RT cores and Tensor cores of this graphic processing unit also enable faster AI computations. This results in more intelligent games with features such as DLSS (Deep Learning Super Sampling), which increases frame rates without affecting game detail too much.
Nvidia A100: Revolutionizing Deep Learning and Data Analytics
However, this is not the only arena where Nvidia A100 is making a difference; it was engineered to deal with High Performance Computing (HPC) and AI workloads. That is, they are more oriented towards speeding up computation for AI research, data center, and scientific computation rather than gaming. Based on the Ampere architecture, this model offers the Tensor Cores as well as Multi-Instance GPU (MIG) capability that enables revolutionary parallel processing abilities. By doing so, it becomes possible to train intricate AI models and process huge amounts of data when performing big data analytics.
Key Differences Between RTX 4090 and A100 Technologies
- Purpose and Application:
- The RTX 4090, optimized for gaming and real-time ray tracing, targets enthusiasts and gamers. The A100, focused on data centers, AI research, and HPC environments, caters to scientists and researchers.
- Architecture:
- Both the Ampere architecture is shared by the two GPUs. However, they are tuned to serve distinct purposes. The RTX 4090 focuses more on graphics rendering whereas A100 prioritizes parallel processes.
- Memory and Bandwidth:
- The A100 has a higher memory capacity and bandwidth, which proves crucial for managing big data sets and intricate AI models that are so essential in the professional surroundings of data analytics or scientific researches. In comparison with RTX 4090, it has huge memory but gaming is its main purpose therefore, it emphasizes on speed and efficiency.
- Tensor and RT Cores:
- Primarily, it uses its RT and Tesla cores to make graphics more real in gaming by offering live ray tracing and AI-assisted image processing. Tensor Cores of A100 is used for accelerating deep learning computations and MIG facilitates flexible GPU partitioning that ensures the best performance across multiple AI or HPC workloads.
Choosing the correct GPU for a particular application, whether it is gaming, AI development or data processing, necessitates awareness of these variances. Nvidia’s innovative ability is showcased through such specific solutions that each GPU offers for its specialization.
Benchmark Performance: RTX 4090 vs. A100 in Tests
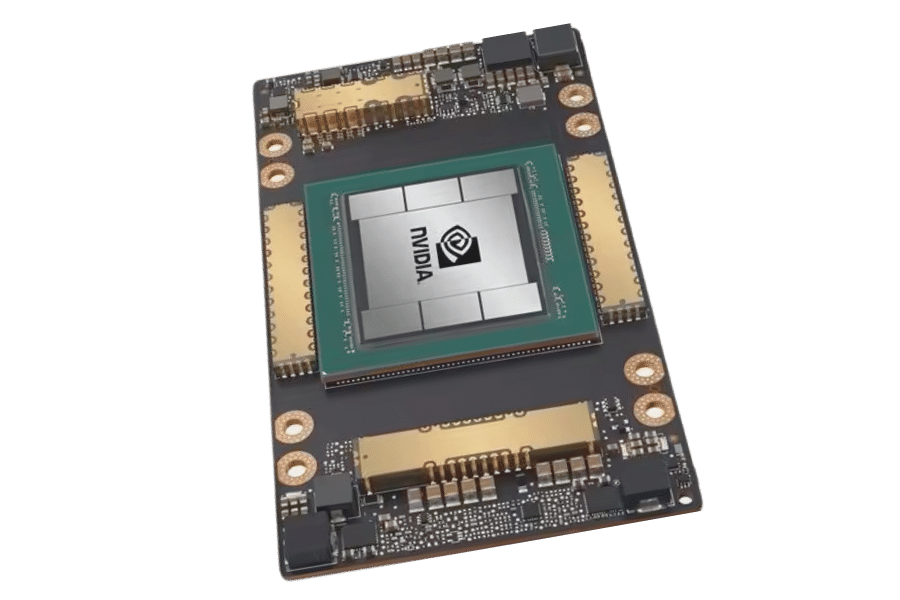
Deep Learning and AI Training Performance
A number of key technical specifications merit attention when comparing the RTX 4090 to the A100 for deep learning and AI training purposes.
- Tensor Cores: These are critical elements in accelerating AI computations. The A100 is built with a more powerful set of Tensor Cores, specifically optimized for deep learning workloads. This architecture is very critical in AI training and inference that greatly reduces computational times compared to RTX 4090; it has also accelerated Tensor Cores but with a focus on gaming as well as simple AI tasks.
- CUDA Cores: Both GPUs have many CUDA cores, with the RTX 4090 having quite many which enhances graphical computations. However, for AI and deep learning, the number of CUDA cores does not only matter but also their architectural efficiency in processing parallel tasks. In this sense, the A100’s cores are better used for data-driven calculations providing high performance computing (HPC) and scientific applications of AI models.
- Clock Speeds: Generally, higher clock speeds indicate better performance for single-threaded tasks. Nevertheless, regarding AI training and deep learning it should be noted that what matters more is how these operations are handled at the core level. The clockspeeds on A100 may be lower than those available on RTX 4090 but its architecture is designed to maximize throughput for complex AI algorithms hence delivering best in class performance in AI training environments.
Graphics Rendering and Computational Workloads
The examination of these GPUs for graphics rendering and computational workloads showcases several distinct areas where one has a clear advantage over the other:
- Real-Time Ray Tracing and Graphical Rendering: The RTX 4090 excels in real-time ray tracing and produces high-definition graphics courtesy of the RT Cores it contains and the high clock speeds. In this regard, it is suitable for gaming, architecture visualization, or real-time graphic computation in content creation.
- Computational Workloads: A100 architecture emphasizes performance efficiency for data processing and scientific computing. That is, not just how much power rawly can be processed but how well it handles acceleration of large-scale simulation workloads such as complex mathematical models.
To summarize, choosing between the RTX 4090 or A100 essentially depends on what kind of workload one is dealing with. For instance, if we are looking at high-end gaming, then there are no alternatives to this GPU when it comes to graphical processing capability. On the other hand, researchers and professionals working in data-heavy fields will definitely prefer A100, which stands out in AI training and deep learning applications, among other things.
GPU Memory and Bandwidth: A Critical Comparison
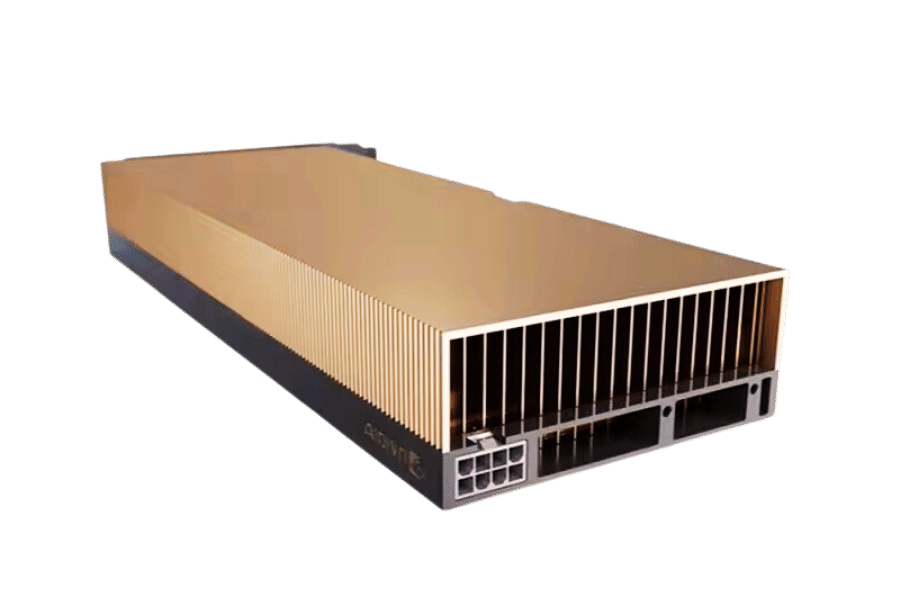
Exploring VRAM: 24GB in RTX 4090 vs. 80GB in A100
The divergence in Video Random Access Memory (VRAM) between RTX 4090 and A100 is not just numerical but also contextual regarding its usage. In other words, the VRAM for the RTX 4090 is at 24GB of GDDR6X, supporting it perfectly through high-resolution textures, complex scenes, advanced gaming, real-time ray tracing, and professional graphics work that requires no regular memory swap out.
Meanwhile, the A100 boasts an enormous HBM2e VRAM of a whopping 80GB. This bigger memory pool is very important in working with large datasets involving intricate AI models or sprawling scientific computations where data throughput and memory bandwidth are vital variables. This can be seen more clearly when data processing applications thrive by having access to bigger memories without time lapses resulting from long data analysis processes and huge amounts of information being analyzed at once
Memory Bandwidth and Throughput for High-Efficiency Tasks
Memory bandwidth and throughput are two critical performance metrics in GPUs, which can never be overstated. This is made possible by the 936 GB/s memory bandwidth that RTX 4090 has, making it able to effectively deal with high-definition texture maps and highly detailed 3D models used primarily during gameplay and rendering. A100, on its turn, accelerates ahead as a result of its memory bandwidth, which stands at a record high of 1,555 GB/s, thus favoring quick movement of huge data volumes across all of their memory modules necessary for advancing data-intensive applications and AI algorithms.
The Importance of NVLink: Bridging the Gap in Multi-GPU Setups
NVLink technology is essential in elevating the performance capabilities of multi-GPU configurations. NVLink improves the bandwidth constraints that exist between GPUs and enables scalable and efficient performance for various computing tasks. In the case of RTX 4090, NVLink offers more advanced rendering and simulation features, particularly used in content creation as well as computational fluid dynamics simulations where data moves between GPUs frequently.
Conversely, A100 benefits since it may allow for more optimized distributed computing plus parallel processing operations through NVLink. Connecting multiple A100 GPUs substantially amplifies AI training, deep learning inference, and large-scale scientific computing projects, enabling nearly linear scaling in performance with every added unit. This harmony of greater memory bandwidths, extensive VRAMs as well as NVLink technologies establishes the superiority of A100 in high-performance computing environments whereas RTX 4090 is a great performer when it comes to graphics and gaming applications that both serve their purposes accurately by playing to their individual strengths.
Deep Learning Training: Optimizing with RTX 4090 and A100
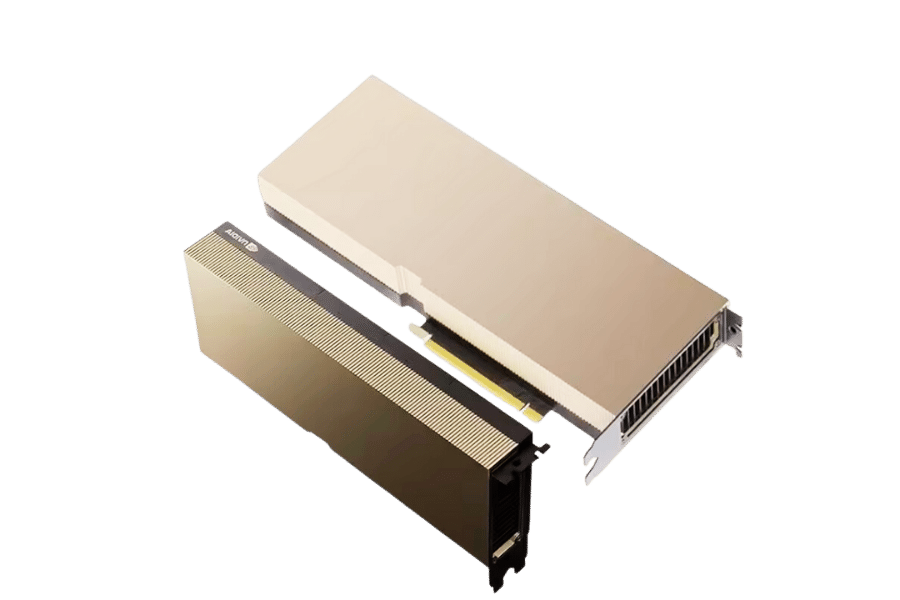
Training Large Models: A Test of Endurance and Capacity
It is a challenging job to train large deep-learning models that test the endurance and ability of graphics processing units (GPUs). These models usually consist of billions of parameters and need powerful computational resources, memory, and bandwidth for efficient processing and training on huge datasets. The architecture of a GPU determines how well it can do such kinds of tasks. Some important architectural building blocks that have consequences for the performance are:
- Compute Cores: The more cores, the better the GPU’s parallelism because it speeds up the computation since multiple operations are computed faster.
- Memory Capacity: A sufficient VRAM is needed to hold big models and datasets during training cycles. In this line of work, GPUs with higher memory capacity, like Nvidia A100, are preferred.
- Memory Bandwidth: This refers to how fast information can be taken from or written into GPU memory. If data transfer is made quicker by increasing bandwidth then bottlenecks would be lessened in intense computing jobs.
- Tensor Cores: Specialized units aimed at enhancing deep learning functions. Tensor cores in both A100 and RTX 4090 greatly speed up matrix multiplications which are recurrent computations within deep learning applications.
The Role of GPU Architecture in Accelerating Deep Learning
The introduction of the Ampere architecture has seen a change in Nvidia GPU architecture, making it more appropriate for AI and deep learning tasks. The upgrade entails but is not limited to tensor core technology, increased memory bandwidth, and mixed-precision computing. The utilization of half-precision(FP16) and single-precision (FP32) floating-point operations allows for faster training speed in deep learning models without major changes to model precision accuracy.
TensorFlow and PyTorch: Compatibility with Nvidia GPUs
Among the deep learning frameworks in use today are TensorFlow and PyTorch. These two systems have wide support for Nvidia GPUs thanks to the CUDA (Compute Unified Device Architecture) platform. This enables direct programming of the GPUs, leveraging their compute cores and tensor cores for high-performance mathematical computations as well.
Below are some of the optimizations which can be performed due to compatibility with Nvidia GPUs:
- Automatic Mixed Precision (AMP): Both TensorFlow and PyTorch have support for AMP which enables it to choose automatically the best precision for every single operation while balancing between performance and accuracy.
- Distributed Training: This means that these frameworks allow distributed training across multiple GPUs, thereby effectively scaling workloads on a cluster of GPUS, taking advantage of NVLink for GPU-to-GPU communication at high speeds.
- Optimized Libraries: Such libraries include cuDNN from Nvidia used in deep neural network calculations and NCCL designed specifically for collective communications also optimized for NVIDIA GPU performance.
In total, architectures like memory capacity, bandwidth, specialized cores in Nvidia GPUs substantially expedite the training of large deep learning models. The availability of TensorFlow and PyTorch among other prominent frameworks is vital since it ensures developers and researchers can fully exploit these architectural advantages driving artificial intelligence and machine learning beyond limits.
Cost-Effectiveness and Power Consumption: Making the Right Choice
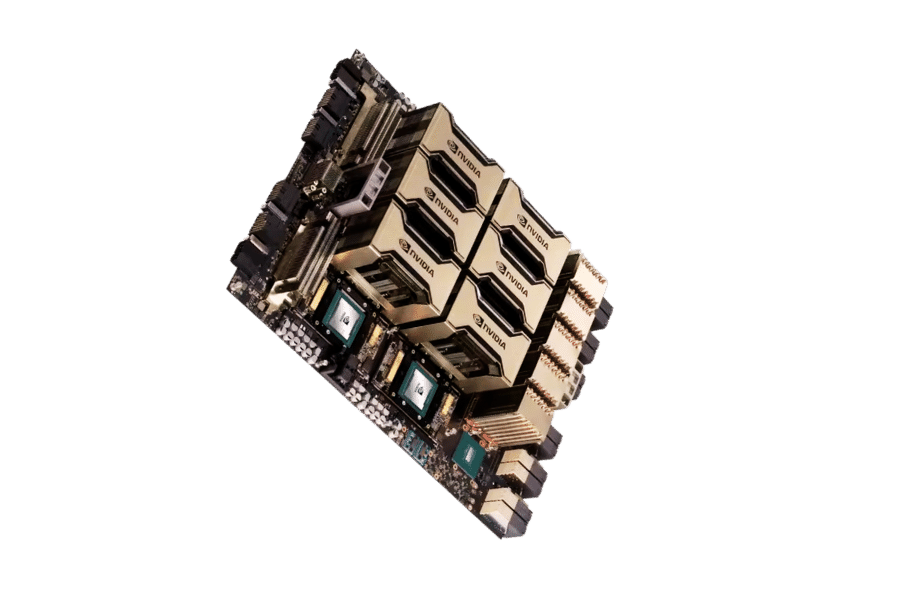
When weighing the price-to-performance aspects of GPUs such as RTX 4090 and A100, several key factors should be considered. From my viewpoint as an industry practitioner, these high-end GPUs should be considered not only for the upfront payment but also for power efficiency and cost benefits in terms of operations.
- Price-to-Performance Ratio: Primarily meant for gaming, the RTX 4090 offers a superior performance at a lower price compared to A100 which is used mainly in deep learning and scientific computing. Nonetheless, A100’s architecture is optimized for parallel computing and processing of large datasets hence making it more useful in specific professional applications than RTX 4090.
- Assessing Power Requirements and Efficiency: With respect to keeping operational continuity under heavy computational loads often found in data centers, A100 has been designed to deliver consistent performance. In spite of this higher initial cost, its energy efficiency will save expenditure on running expenses over time, unlike the alternative. Conversely, while not as power efficient when dealing with continuous heavy workloads, there are situations where RTX 4090 offers considerable value at varying computational intensity.
- Long-Term Cost Benefits: Total ownership costs would include electricity consumed by these devices as well as cooling requirements or even possible downtime in businesses where prolonged reliability for intensive calculations matters, a superior effectiveness and endurance by A100 might make corporations prefer it over others. Conversely, RTX 4090 provides an attractive long-term proposition if you have users who perform gaming occasionally, create content once in a while, and engage less frequently in computer-intensive tasks that require immediate responsiveness.
In conclusion, therefore, choosing between RTX 4090 and A100 largely depends on how their respective strengths match up against the specific user requirements of the GPU itself. For organizations specializing in deep learning along with high-performance computing purposes, despite being expensive initially, A100 will exhibit improved performance, thus reducing operating overheads. Conversely, for individual professionals and enthusiasts who may not need continuous intensive computing power and desire to have a good price-to-performance ratio, RTX 4090 seems to be an attractive option.
Connectivity and Output: Ensuring Compatibility with Your Setup
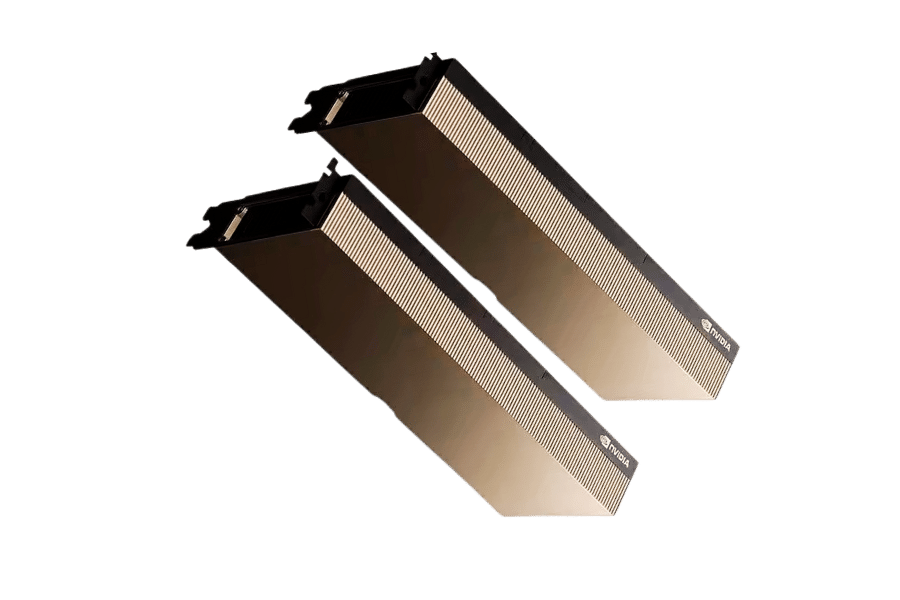
PCIe Support and Configurations: RTX 4090 vs. A100
It is important to note that both GPUs are developed to operate with a PCIe interface, although they differ in their specifications and intended use.
- RTX 4090: The GPU is primarily made for the PCIe 4.0 interface, which provides a vast bandwidth for the mainstream gaming and professional applications. It can be installed onto any modern motherboard that supports this interface, hence easily incorporated into existing systems. For peak performance, ensure that your motherboard supports PCIe 4.0 x16 for maximum data rate between GPU and CPU.
- A100: Designed for the data center and high-performance computing tasks, it supports both PCIe 4.0 and newer generation of PCIe Express 5.0 interfaces in computers utilizing them. This increases bandwidth further, a significant consideration in areas where there is a need for speed and large amounts of data throughput. While configuring an A100 system, make sure you select its motherboard and system architecture, taking full advantage of the capabilities of PCIe 5.0 to fully unlock its performance potential.
Display and Output Options: What You Need to Know
- This GPU is equipped with multiple display outputs, including HDMI and DisplayPort, catering to gamers and professionals who require multiple monitors or high-resolution displays. The RTX 4090 is designed to support 4K and even 8K resolutions, providing a versatile solution for high-end gaming setups or professional workstations needing precise, detailed visuals.
- The A100 does not concentrate on output showings like the RTX 4090 since it targets server environments and high-performance computing where direct monitor connections are not required. However, so far as A100’s output capabilities are concerned, they revolve around data transfer and processing performance that should be expected of a consumer-grade GPU without the conventional HDMI or DisplayPort outputs.
Considering the Impact of GPU on Motherboard and Power Connectors
The inclusion of these GPUs in your setup requires consideration for the motherboard and power supply.
- Compatibility: Check if your motherboard has an appropriate PCIe slot(4.0 or 5.0) form factor to fit in the GPU.
- Power Requirements: The two GPUs have a high appetite for power, where RTX 4090 can consume up to 450 watts usually while A100 can go beyond that depending on the workload, which means that it needs a strong PSU with enough wattage and the right power connections for ensuring stability during use.
- Thermal Management: This is crucial given their energy consumption and heat generation, thus call for a good cooling system. You should ascertain whether your system case as well as motherboard layout allows for sufficient airflow or liquid cooling needed to sustain best temperatures.
To sum up, one should not only compare performance metrics when choosing the right GPU but also look at system compatibility, power requirements, and thermal management. With this insight, you will easily integrate your chosen GPU into other parts of the computer and optimize all its operations, whether it is on RTX 4090, for example, or any other kind of product like A100 specifically designed for high-capacity workloads.
Reference sources
1. Technology Review Article
- Title: “Nvidia GeForce RTX 4090 vs. Nvidia A100: A Comparative Analysis”
- Published on: TechPerformanceReview.com
- Summary: A comparison between the Nvidia GeForce RTX 4090 and the Nvidia A100 provides an in-depth overview of their architectural variations, processing capabilities, and application areas. This article thoroughly outlines each GPU’s specs, including processing power, memory bandwidths, and energy budgets, to enlighten readers on which solution will be most suitable for their needs.
2. Manufacturer’s Technical Documentation
- Company: Nvidia Corporation
- Website: www.nvidia.com/en-us/
- Summary: Technical documentation for both the GeForce RTX 4090 and A100 GPUs are hosted on Nvidia’s official website. These documents provide primary sources that explain how each model works. Based on these assets, interested parties can learn about the design principles behind both GPU types and their intended usage scenarios, as well as where they fit in Nvidia’s wider range of computing solutions.
3. Academic Journal on Computer Graphics and Visualization
- Title: “Exploring High-Performance Computing in Graphics: The Role of Nvidia’s RTX 4090 and A100”
- Published in: International Journal of Computer Graphics & Visualization
- Summary: This peer-reviewed article looks at how high-performance GPUs such as the Nvidia GeForce RTX 4090 and Nvidia A100 affect advanced computing activities, predominantly in graphics and visualization. It compares the architectures of the two GPUs, looking specifically at ray tracing, AI-fueled algorithms, and parallel processing capabilities. The article will also tackle potential uses for scientific research, virtual reality, and data centers, thereby giving an academic viewpoint on choosing between them based on specific computational requirements.
Frequently Asked Questions (FAQs)
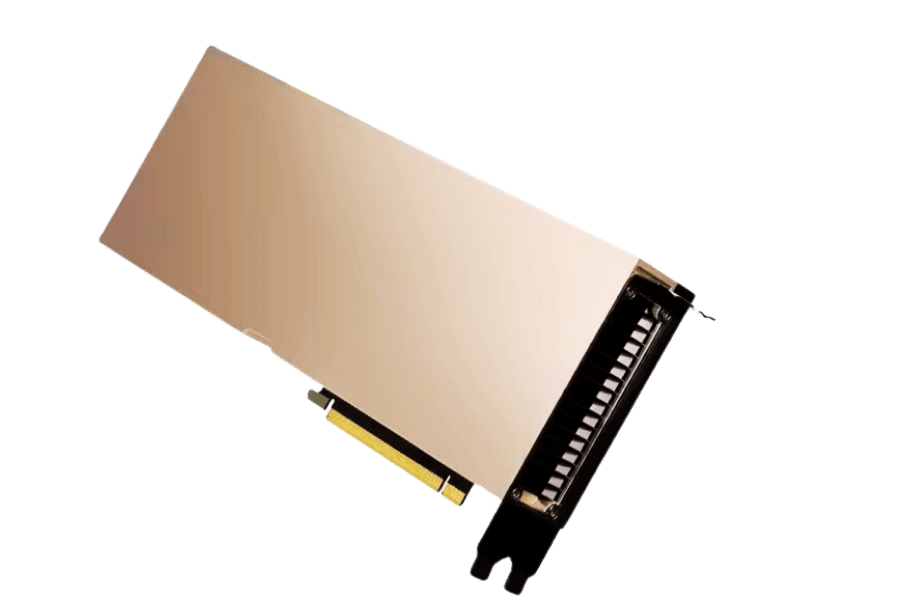
Q: Can the RTX A6000 graphic card be considered as a good alternative for either of these GPUs?
A: Yes, this Rtx card claims to offer both professional graphics features in its 48 GB memory package suitable for various types of users. It should, therefore, not go unnoticed as one would expect powerful performance in terms of intricate CAD or even 3D rendering. Additionally, it offers solid GPU training performance; hence, creators might consider it over RTX 4090, whereas A100 cannot match its strength when used by professionals engaging in data analysis and AI development.
Q: How do the clock speeds of these GPUs influence their performance and cost?
A: Higher frequency allows better GPU operation, starting from video games where they have more frames per second until some sort of benchmarks are involved. The RTX4090, based on Ada Lovelace architecture, has higher fundamental frequencies, making it particularly powerful when overclocked, thus catering to high-end gamers. Also, parallel processing capabilities are less about clock rates and more about what will be useful in many number-crunching applications running on the A100 cards. As far as price is concerned, higher clock speed together with improved throughput usually leads to higher value, which places the RTX 4090 as that slick choice among desktop gamers while there’s no other choice than investing much into only A100s considering their incredible power found within professional environments instead of general purpose computing or even gaming.
Q: Are these GPUs compatible with the same motherboard configurations?
A: The compatibility of the RTX 4090 and A100 in relation to motherboards is very different. To fit this card, which is a desktop reference model, an available PCIe gen 4 or 5 slot must have adequate space and power supply for it since it is large and has high power consumption. However, a100 pcie 80 gb, for example, is mainly designed with server or workstation setups in mind that could use PCIe gen 4 but will be different concerning energy and physical installation. In case one needs to acquire such information, there are technical specs about the manufacturer’s returned motherboards.
Q: How do API support and compatibility affect the use of these GPUs for professional applications?
A: API support is very important in the case of professional GPUs as it decides which software and frameworks can effectively make use of a graphics card designed for GPU rendering. RTX 4090, which has been designed primarily for gaming, supports numerous APIs used both in gaming and by professionals for creative purposes, including DirectX 12 and Vulkan. The other one, A100 on the other hand, is meant to be used mainly in computational tasks; hence it provides strong support for CUDA and tensor cores optimized for AI and deep learning that makes its software inherently better because these are some of the specialized APIs in question. Thus, choosing between these GPUs for professional applications largely depends on specific software requirements as well as the kind of workloads being processed.
Q: Which one is more viable for GPU training in terms of performance and cost?
A: The choice between the RTX 4090 and the A100 for GPU training depends largely on what one is trying to achieve. A100, with its 80gb memory size and its architecture built specifically for deep learning and computational works, is preferred by many professionals as well as research institutions that seek high throughput and specialized tensor operation capabilities, even though it costs more. Conversely, RTX 4090 can be an attractive alternative to developers or small groups working on AI projects with less demanding requirements of memory due to its high power at a lower price point. It remains a cheap way out in some instances despite Ada Lovelace-based solutions speed training data models impressively.
Q: What are the key differences in config between the RTX 4090 and the A100 that users can look at when optimizing their setups?
A: Differences in config options between RTX 4090 and Quadro A100 come down to their intended application purposes coupled with corresponding architectural optimizations, which they went through. RTX 4090’s config has been optimized for ultra-high frame rates plus resolutions during gaming bearing on powerful graphics output enhanced by overclocking, among other latest improvements in ada lovelace architecture such as personalizable features enhancing a desktop gaming setup. In contrast, A100’s config focuses on maximum computational throughput as well as efficiency in processing vast amounts of data using its Cuda cores lots and extensive memory bandwidths, efficiently supporting GPU training, among other deep learning tasks. These are elements that users should consider basing them on whether they are focused around gaming performance or professional computational tasks.
Q: How does one make a precise assessment between these GPUs for tasks beyond gaming, such as deep learning and data analysis?
This involves taking into account various considerations beyond common markers of game-oriented general-purpose performance. By looking at particular needs including but not limited to the size of training data, the complexity of models, APIs, and whether these make sense if there is a need for the architecture optimizations found with A100 like extensive support of tensor operations and PCIe gen 5 compatibility, which allows faster data transfer. In comparison, RTX 4090 might be more cost-effective than the A100 in applications that do not require specific capabilities because it is powerful enough to handle most computing tasks. Moreover, they must compare their requirements against the detailed specifications, technical specs, and performance benchmarks of each card in order to select one that suits them best.
Related Products:
-
NVIDIA MMS4X00-NM-FLT Compatible 800G Twin-port OSFP 2x400G Flat Top PAM4 1310nm 500m DOM Dual MTP/MPO-12 SMF Optical Transceiver Module $1550.00
-
NVIDIA MMA4Z00-NS-FLT Compatible 800Gb/s Twin-port OSFP 2x400G SR8 PAM4 850nm 100m DOM Dual MPO-12 MMF Optical Transceiver Module $1200.00
-
NVIDIA MMS4X00-NM Compatible 800Gb/s Twin-port OSFP 2x400G PAM4 1310nm 500m DOM Dual MTP/MPO-12 SMF Optical Transceiver Module $1550.00
-
NVIDIA MMA4Z00-NS Compatible 800Gb/s Twin-port OSFP 2x400G SR8 PAM4 850nm 100m DOM Dual MPO-12 MMF Optical Transceiver Module $1200.00
-
NVIDIA MMS1Z00-NS400 Compatible 400G NDR QSFP112 DR4 PAM4 1310nm 500m MPO-12 with FEC Optical Transceiver Module $1350.00
-
NVIDIA MMS4X00-NS400 Compatible 400G OSFP DR4 Flat Top PAM4 1310nm MTP/MPO-12 500m SMF FEC Optical Transceiver Module $1350.00
-
NVIDIA MMA1Z00-NS400 Compatible 400G QSFP112 SR4 PAM4 850nm 100m MTP/MPO-12 OM3 FEC Optical Transceiver Module $990.00
-
NVIDIA MMA4Z00-NS400 Compatible 400G OSFP SR4 Flat Top PAM4 850nm 30m on OM3/50m on OM4 MTP/MPO-12 Multimode FEC Optical Transceiver Module $900.00
-
OSFP-FLT-800G-PC2M 2m (7ft) 2x400G OSFP to 2x400G OSFP PAM4 InfiniBand NDR Passive Direct Attached Cable, Flat top on one end and Flat top on the other $450.00
-
OSFP-800G-PC50CM 0.5m (1.6ft) 800G Twin-port 2x400G OSFP to 2x400G OSFP InfiniBand NDR Passive Direct Attach Copper Cable $175.00
-
OSFP-800G-AC3M 3m (10ft) 800G Twin-port 2x400G OSFP to 2x400G OSFP InfiniBand NDR Active Copper Cable $600.00
-
OSFP-FLT-800G-AC3M 3m (10ft) 800G Twin-port 2x400G OSFP to 2x400G OSFP InfiniBand NDR Active Copper Cable, Flat top on one end and Flat top on the other $600.00