At a recent seminar, LightCounting projected that the shipment volumes of optical transceiver modules and Optical Circuit Switches (OCS) will see explosive growth over the next five years. This growth may experience occasional slowdowns but is expected to quickly rebound thereafter. Currently, annual shipments of AI cluster optical modules amount to tens of millions, with a projected increase to nearly 100 million units by 2029. OCS shipments, which reached 10,000 units in 2023, are expected to surpass 50,000 units by 2029.
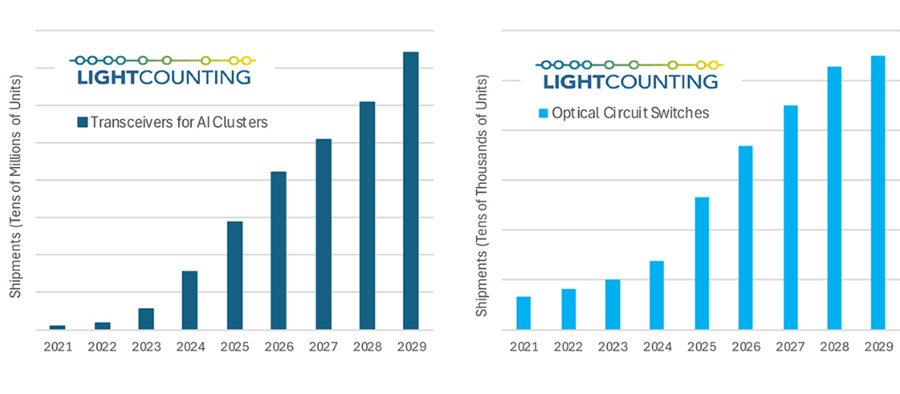
Over a decade ago, Google started using OCS in its computing nodes and AI clusters. The company has recently highlighted the benefits of architectures supporting OCS in several reports. Other major AI cluster providers, including Nvidia and Microsoft, have also begun using OCS, with many additional operators seriously considering the advantages of following suit.
The demand for OCS will undoubtedly be strong, with more complex optical switching applications expected in the future. Packet switching presents challenges due to the lack of practical solutions for optical buffering, but large data flows can be routed optically.
Back in 2007, Google was the first company to use optical transceivers in its data centers. Despite a brief interruption due to the 2008-2009 financial crisis, the company fully resumed the adoption of this technology in 2010. Over the past decade, many other cloud computing companies have followed Google’s lead. Nvidia (Mellanox) preferred Active Optical Cables (AOC) until two years ago but became the largest consumer of 400G/800G transceivers in 2023.
Nvidia now uses optical transceivers for Ethernet and InfiniBand connections between servers and switches. The company announced plans two years ago to use optical devices for NVLink connections and demonstrated this in one of its internally built clusters. The bandwidth required for NVLink connections is nine times that of InfiniBand, making cost and power consumption reductions for optical devices essential for this new application.
As shown in the Figure below, Google’s and Nvidia’s AI cluster architectures are compared. Google’s TPU clusters do not require Ethernet or InfiniBand switches but use OCS. Each TPU can communicate directly with its six nearest neighbors, and OCS can expand and reconfigure these tightly connected networks. In contrast, Nvidia’s design heavily relies on InfiniBand, Ethernet, and NVLink switches, requiring more optical connections than Google’s design.
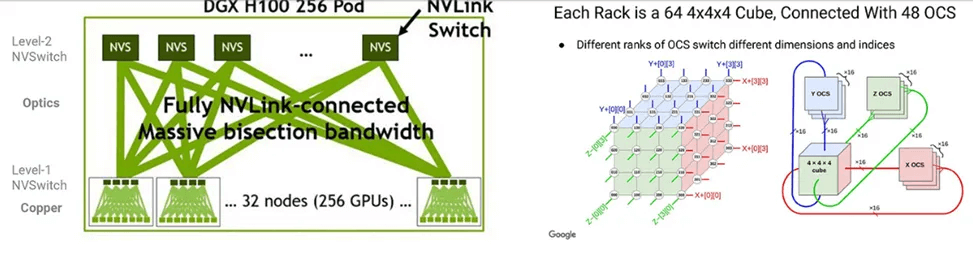
The differences in AI cluster architecture between Google and Nvidia lead to varying priorities for optical interconnects, as shown in the figure. Google utilizes Optical Circuit Switches (OCS) and prioritizes a higher link budget to offset the 1.5 dB optical loss of OCS. In contrast, multi-wavelength FR4/FR8 transceivers increase OCS throughput by four to eight times compared to DR4/DR8 modules.
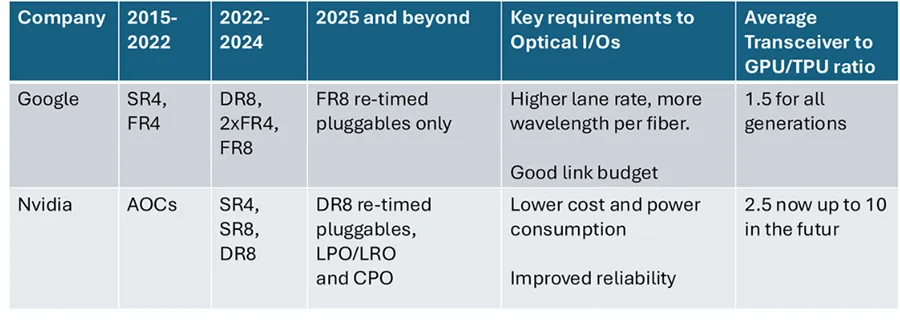
On the other hand, Nvidia prioritizes cost and power reduction to accommodate the large number of transceivers required for its clusters. The company is highly supportive of Linear Drive Pluggable Optics (LPO) and Co-Packaged Optics (CPO). Google is less interested in LPO or CPO, as it continues to use a design averaging only 1.5 transceivers per TPU. In the future, Nvidia might need up to 10 transceivers per GPU to support NVLink over fiber.
LightCounting predicts that Nvidia will deploy LPO and/or CPO in the next 2-3 years, reducing power consumption from 10-15 pJ/bit to 4-7 pJ/bit to achieve NVLink over fiber, as illustrated in Figure. Google has already used optical devices for inter-core interconnect (ICI) between TPUs.
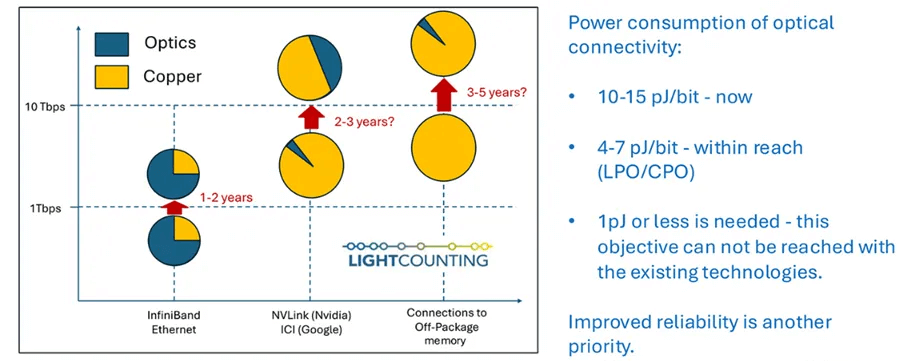
Performance Scaling Challenges
The reliability of all components within AI clusters is critical for scaling these systems. A single GPU or network link failure can reduce the entire cluster’s efficiency by 40%, and mitigating such failures (through software) may take up to 10 minutes. These failures occur every 30-45 minutes on average, worsening in larger clusters with more complex GPUs and optical devices.
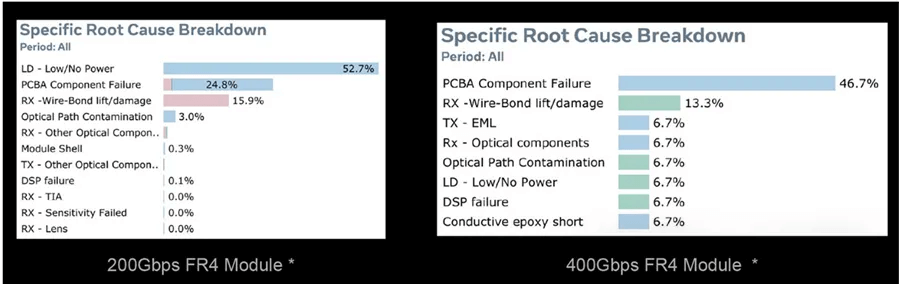
The figure (Source: Meta) shows transceiver failure analysis data for 200G FR4 and 400G FR4 modules. Directly modulated laser degradation is the main source of 200G module failures. Issues with externally modulated lasers used in 400G transceivers are less than general manufacturing problems related to PCB assembly and wire bonding. More integrated wafer-level design and manufacturing are crucial for improving optical device reliability.
y the end of 202X, GPU performance is expected to significantly improve through a combination of CMOS, substrate and packaging methods, chip architectures, and better cooling technologies. Managing the heat dissipation of these ultra-large chip components is among the many challenges, making energy efficiency critical for all technologies used in AI clusters. CMOS is set for deployments moving from 5 nm to 3 nm and 2 nm within the next five years, but optical interconnects are still seeking ways to enhance energy efficiency.
LightCounting expects linear drive optics to be deployed on a large scale within the next five years, whether as pluggable transceivers (LPO) or CPO. The industry will require new materials and equipment to further improve power efficiency. Some new technologies might take up to ten years to become available, but some will be adopted within the next five years. This is an arms race, with customers willing to take on greater risks.