Hardware solutions must be robust now that artificial intelligence (AI) technology is advancing quickly. Such solutions should be capable of simultaneously processing complex calculations and large amounts of data. This makes the NVIDIA H200 GPU a game changer in this field as it has been designed for this exact purpose – to handle modern AI workloads effectively. In addition, its state-of-the-art architecture, coupled with unmatched processing power, positions it well for improving performance in machine learning, deep learning, and data analytics, among other applications. The article also discusses technical specifications, features, and real-world use cases for this type of graphics card, thus demonstrating how much more efficient and effective artificial intelligence can become across different industries with NVIDIA H200 GPU.
What is the NVIDIA H200 GPU?
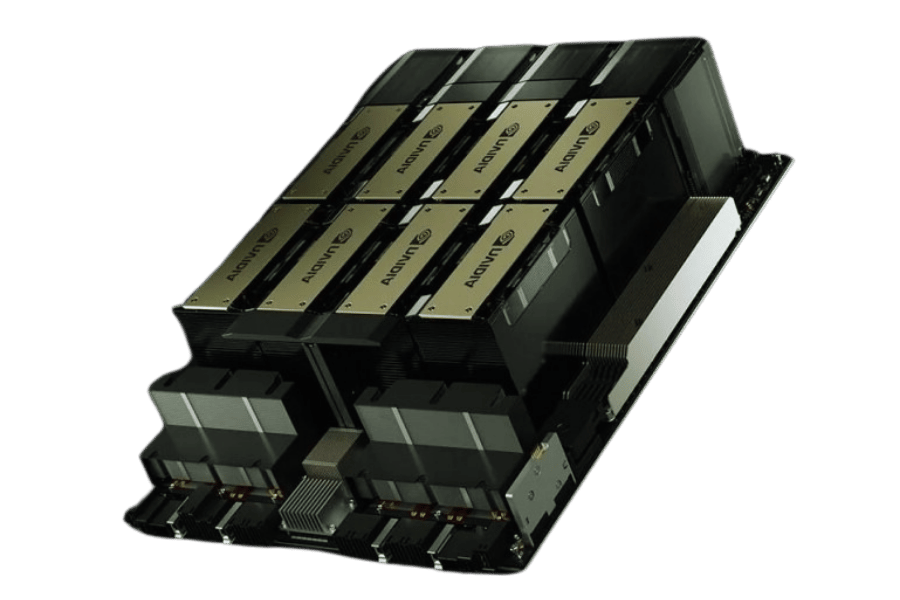
Introduction to NVIDIA’s H200 Series
The highest level of GPU technology for AI and high-performance computing is the NVIDIA H200 series. It is built with a new architecture that makes it more scalable than its predecessors. It can handle bigger workloads at faster speeds and greater precision than H100. It has advanced tensor cores, which accelerate AI model training and inference, thus significantly reducing data-driven time-to-insights. Moreover, these GPUs have been optimized to work seamlessly with other NVIDIA software environments, such as CUDA and TensorRT, to not disrupt existing workflows while providing an upgrade path from H100 SXM. Such integration between hardware and software makes H200 an essential toolset for any company looking forward to leveraging AI advancements in their operations.
How the H200 Compares to the H100 GPU
The H200 GPU is much better than the H100 GPU in many ways. Initially, it has more powerful processing capabilities due to an increased bandwidth of memory and greater data throughput supported by a redesigned architecture. Thus, this leads to higher efficiency in tasks related to training artificial intelligence models than ever before. Also, extra tensor cores are introduced into the H200, which can perform complex computations with improved precision and speed, especially useful in deep learning applications.
Another thing about the H200 is that it has power management systems designed for optimization, hence cutting down energy consumption while still delivering maximum performance even when under heavy loads. Regarding scalability, multi-GPU setups are better supported by the H200, thus allowing organizations to deal with large-scale AI workloads more efficiently. In general terms therefore – these improvements make the new version of GPU (H200) much more effective in addressing present-day challenges faced by Artificial Intelligence as compared to its predecessor (H100), thereby becoming an attractive option for businesses seeking to enhance their AI capabilities.
Unique Features of H200 Tensor Core GPUs
Artificial intelligence and machine learning find this group of NVIDIA H200 Tensor Core GPUs useful because of its many exclusive characteristics. One such characteristic is the presence of third-generation tensor cores, which enable mixed-precision computing and improve performance and efficiency in deep learning workloads. These tensor cores are capable of executing operations with FP8, FP16, and INT8 data types very efficiently, thus accelerating training and inference while at the same time optimizing memory usage.
Besides, the dynamic scaling of resources is supported by H200 GPUs, which can adjust themselves according to real-time workload demands. This feature allows for higher reliability in data-intensive tasks together with better error correction capabilities. The advanced NVLink architecture integration, on the other hand, establishes better connectivity between GPUs necessary for scaling up AI computations over larger datasets and more complex models, hence making it good for generative AI and HPC, too. All these make the H200 a high performer with great capability that can meet growing needs for enterprise AI systems.
How Does the NVIDIA H200 Support AI and Deep Learning?
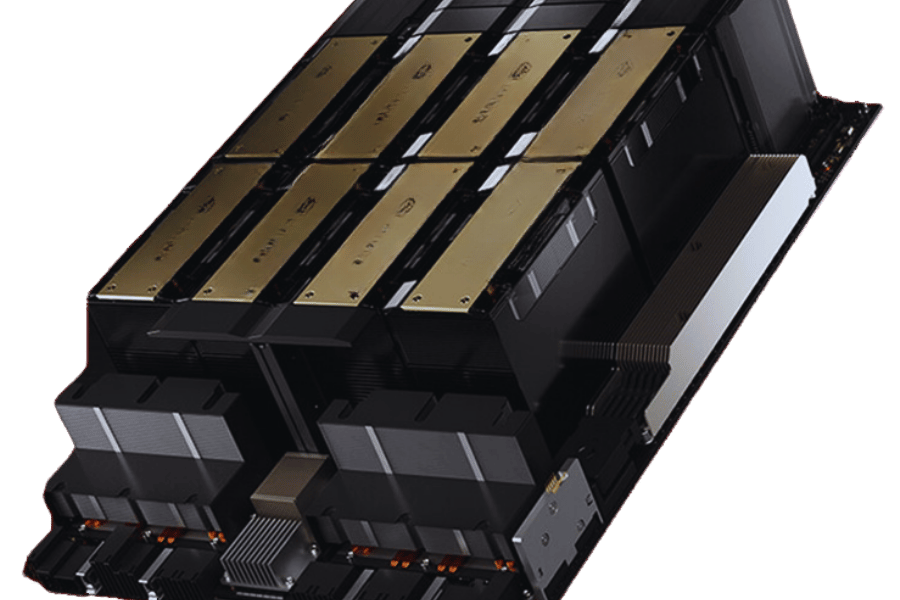
Enhancements for AI Inference
AI inference is improved by the NVIDIA H200 Tensor Core GPUs through a number of changes that improve efficiency and speed. One of these is a reduction in latency during inference tasks through optimized algorithms and hardware acceleration that make real-time processing of AI models possible. It also allows for mixed precision computing, which enables faster inference times without sacrificing accuracy, giving companies an edge when deploying AI applications. In addition to this, there are many software supports available, such as TensorFlow or PyTorch compatibility, so developers have access to all features while using H200 for streamlined AI deployment. The GPU’s architecture is further utilized by integrating specialized libraries for AI inference, thus leading to efficient model optimization. Taken together, these improvements establish H200 as a powerful means for implementing advanced AI-driven applications by any establishment.
Boosting Generative AI and LLMS Performance
NVIDIA H200 Tensor Core GPUs have been built specifically to increase the performance of Generative AI models and Large Language Models (LLMs). These improved model training are enabled by the advancements in memory bandwidths and efficient tensor operation handling provided by H200, which can support more complex architectures with larger datasets. The ability of the architecture to achieve high throughout is what makes it so effective at speeding up training cycles for generative models, which in turn helps achieve quick iterations and refines them into good ones. Additionally, transformer-based model optimizations that work as foundations for most LLMs also make data propagate through the model faster, hence reducing training time and resource consumption. Enterprises should, therefore, take advantage of these features if they want to realize the full potentialities behind generative AI technologies in their various applications for innovation and efficiency gains.
Tensor Core Innovations in the H200
NVIDIA H200 Tensor Core GPUs bring in many new things, making it much more powerful for AI workloads. One of the most prominent advancements is better mixed-precision support that enables training and inference to be done with different precision modes depending on the specific needs of each operation, thus saving time as well as optimizing memory utilization, especially when dealing with big models. Moreover, this system has been equipped with improved sparsity features, which allow it to skip zero values within sparse matrices during computation, leading to faster neural networks without compromising accuracy. Additionally, support has been added at the hardware level for advanced neural network architectures like recurrent nets and convolutions so that complex tasks can be executed efficiently by the H200 without sacrificing speed. These are just but some of the other breakthroughs in this area, hence making it an ideal device for anyone who wants top-notch performance while working on AI projects.
What Are the Benchmark Performance and Workload Capabilities of the H200?
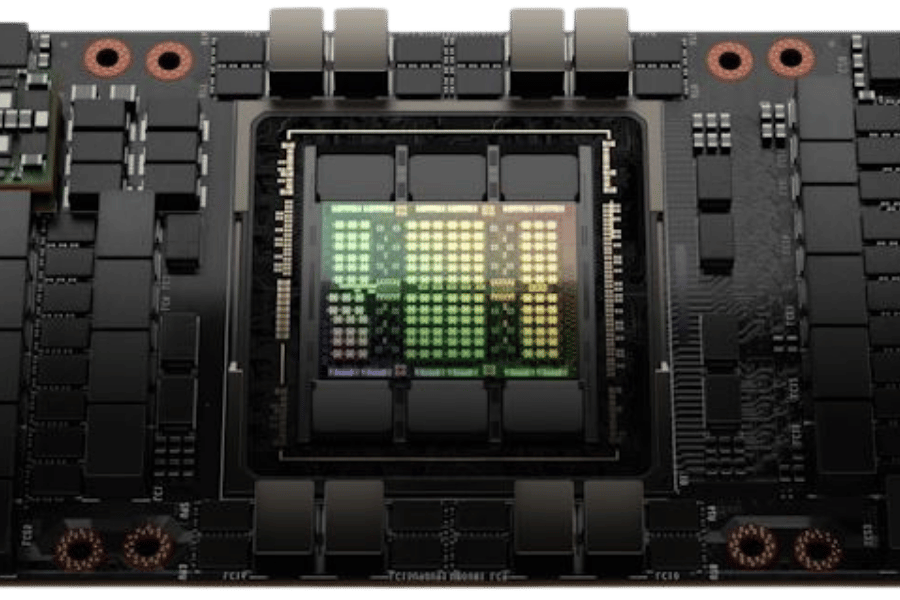
Performance Metrics and Benchmarks of the NVIDIA H200
The NVIDIA H200 Tensor Core GPUs are built to have better performance numbers across various AI workloads. The H200 has been shown to beat previous-generation GPUs by a large margin in benchmark tests, especially when it comes to deep learning and large-scale training tasks. Throughput is also expected to be improved based on performance benchmarks from leading sources, with some applications having up to 50% faster training times than their predecessors.
In addition, the GPU’s ability to process complex computations efficiently can be seen in the way it handles large datasets while using less memory due to its enhanced mixed-precision capabilities. What sets this product apart is its exceptional scalability, which enables support for many concurrent workloads without any drop in performance levels, therefore making H200 an ideal choice for enterprise customers who require high-performance AI solutions. These facts can be supported by referring back to comprehensive benchmark data collected by well-known tech review websites that focus more on these figures as against those recorded during tests carried out with other models, such as H100, which were not so effective at enhancing AI capabilities efficiently according to technology review sites that can verify this information.
Handling HPC Workloads with the H200 GPU
The NVIDIA H200 Tensor Core GPU is built for high-performance computing (HPC), which usually involves heavy computations and large-scale data processing. Its architecture allows it to execute parallel workloads more efficiently, thus reducing the time needed to solve complex simulations or analyses.
Among the reasons it is effective in HPC are improved memory bandwidth and dynamic resource allocation based on workload needs. This improves performance-to-power balance, which is very important in big data centers. Additionally, it has advanced computational capabilities like double-precision floating-point operations, which are useful for scientific computations needing high accuracy.
One great thing about this product is its ability to support multi-GPU configurations, which allows scaling seamlessly across multiple nodes within a cluster environment, thereby increasing computing power. Throughput will be higher than ever before for those who use multiples of these devices in their work, while operational costs related to inefficient computing practices will also go down drastically. Therefore, such an invention acts as a game-changer for organizations involved in cutting-edge research activities as well as those carrying out heavy computational tasks.
Comparison to H100 Performance Metrics
Comparing the NVIDIA H200 GPU to its predecessor, H100, reveals several key metrics of performance. These include a better floating point with double precision calculations showing up to 30% improvement as indicated by benchmarks which is necessary for scientific simulations that require high accuracy; memory bandwidth has also been enhanced so that larger datasets can now be supported and data transferred more efficiently – this becomes vital for today’s workloads in HPC.
Additionally, the resource allocation feature of H200 is dynamic, thus outperforming H100 because it allows for better management of workloads, thereby resulting in quicker processing times. Also, in terms of multi-GPU scalability, H200 supersedes H100 such that organizations can easily scale their computing power without any hitches. Therefore, not only does it increase efficiency, but it also provides support for cutting-edge research applications that demand intensive computation capabilities as well. This change represents a significant step forward in GPU architecture development for institutions seeking to explore new frontiers within high-performance computing (HPC).
What is the Memory Capacity and Bandwidth of the NVIDIA H200?
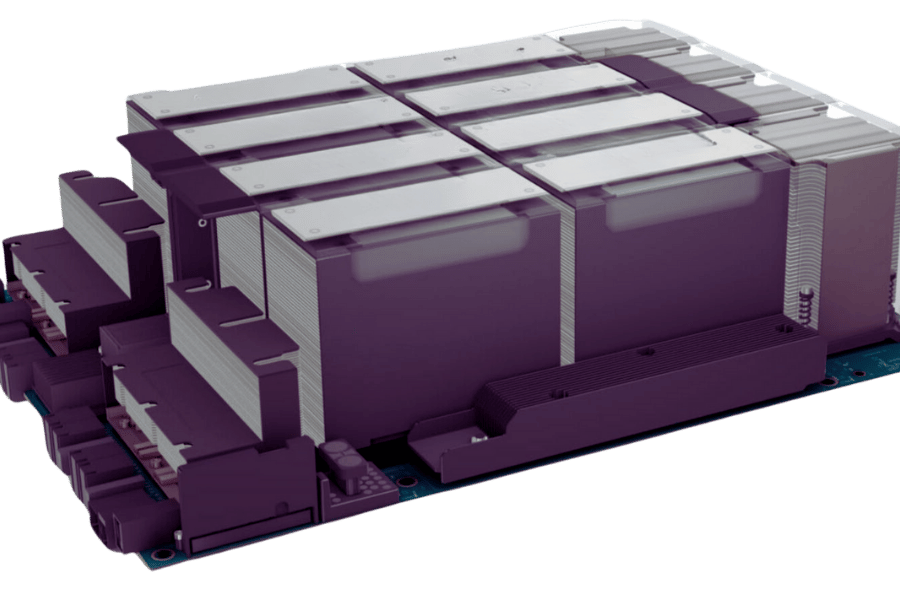
Details on HBM3E Memory Integration
HBM3E (High Bandwidth Memory 3 Enhanced) is integrated into the NVIDIA H200 GPU, which boasts of memory capacity and bandwidth optimized beyond those of its predecessors. Normally rated at 64 GB per GPU, the memory capacity offered by HBM3E is massive; hence, it is most suitable for use in demanding systems that involve a lot of data manipulation. This development results in memory bandwidths that exceed 2.5 TB/s; such speeds enable fast transfer rates necessary for high-performance computing environments.
Additionally, the design behind HBM3E comes with wider memory interfaces and uses advanced stacking techniques, thereby allowing better heat dissipation and saving power consumed during operation. With this storage technology put into practice, the overall performance of the H200 is greatly improved, especially when dealing with large datasets, machine learning models, or complex simulations that heavily rely on quick access to memory resources. This means that through this integration, the H200 can handle any future computational task required by cutting-edge research or industrial applications in various fields.
Memory Capacity and Bandwidth Specifications
NVIDIA H200 GPU is designed to offer excellent memory capacity and bandwidth that will enhance performance when used in demanding computing environments. Here are the main specifications:
- Memory Capacity: The H200 can accommodate memory of up to 64 GB HBM3E per GPU, thus enabling it to effectively work with large data sets and handle complex calculations.
- Memory Bandwidth: With over 2.5 TB/s of bandwidth, it has a faster rate for transferring information, which is essential for such applications as machine learning, AI, or large-scale simulations.
- Architecture benefits: This architecture uses advanced stacking technologies and wider memory interfaces to improve thermal control, energy usage efficiency, and overall computing power.
These characteristics make NVIDIA H200 one of the best choices for professionals and organizations aiming at pushing forward research in high-performance computing.
How Does the NVIDIA HGX H200 Enhance Compute Power?
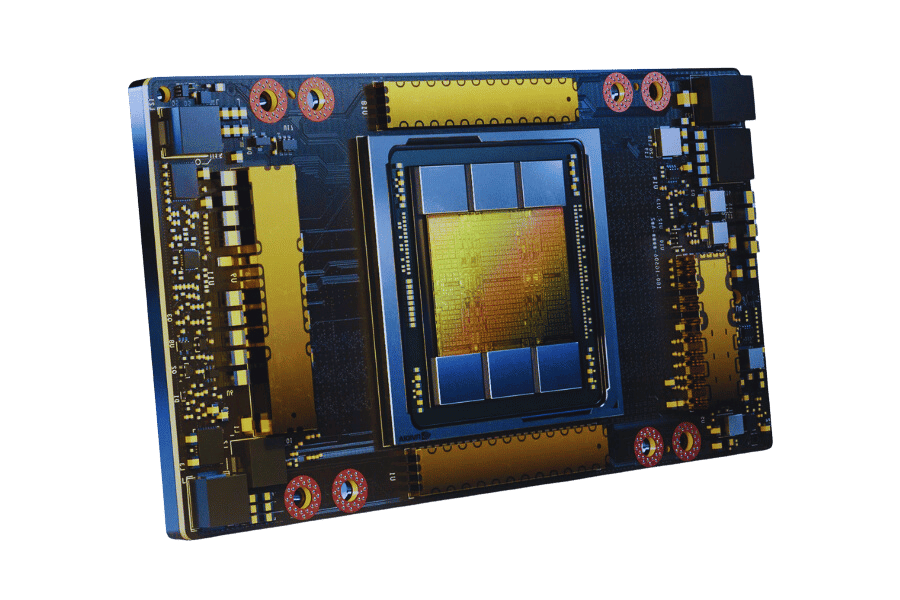
Benefits of NVIDIA HGX H200 Systems
In comparison to H100, NVIDIA HGX H200 systems have several advantages that significantly improve their computing power in demanding applications. Some of these benefits are:
- Optimized for AI and HPC: HGX H200 is designed specifically for artificial intelligence (AI) and high-performance computing (HPC) workloads. In this architecture, parallel processing is handled efficiently so that large volumes of data can be quickly analyzed and models trained.
- Scalability: Using the architecture of HGX H200, organizations may increase performance step by step while scaling their computational capacities. This feature suits cloud service providers and enterprises seeking resource optimization well.
- Enhanced thermal efficiency: The incorporation of state-of-the-art thermal management techniques into the design of HGX H200 guarantees that even under maximum loads, the system will keep working at optimal temperatures. This characteristic leads to a longer life span as well as higher reliability of hardware, which eventually cuts down on operational costs, especially when coupled with the latest NVIDIA GPUs.
These advantages, therefore, make organizations in fields such as deep learning, data analytics, and scientific simulations, as well as any other field where research boundaries need to be stretched, realize that they cannot do without NVidia’s HGX H200 systems.
AI Workloads Acceleration with HGX H200
The ideal GPU for artificial intelligence development is the NVIDIA HGX H200 since it speeds up AI workloads through its advanced architecture as well as processing power. Firstly, it carries out tensor operations effectively during deep learning training by use of tensor core technology thus accelerating the speed of training. Furthermore, this optimization works best when handling large-scale data because it enables quick analysis of huge datasets, which is necessary for such AI applications as computer vision and natural language processing, where the HGX H200 comes in handy. In addition to that, fast interconnects are used so that complex calculations can be made without much delay while transferring data between nodes, which is also done very quickly thanks to their high speed. These functionalities provide the joint ability that allows scientists to come up with new ideas quickly and shorten AI solutions’ time spent on development by developers, thereby heightening companywide system performance in enterprise settings ultimately.
Reference Sources
Frequently Asked Questions (FAQs)
Q: How would you describe the NVIDIA H200 GPU?
A: NVIDIA’s current edition of GPUs is the NVIDIA H200 GPU, which has been designed to accelerate AI work and enhance generative AI and High-Performance Computing (HPC) efficiency. Comparatively, it has more capabilities than the previous model, the NVIDIA H100 GPUs.
Q: What is the discrepancy between the NVIDIA H200 and H100?
A: Better tensor core GPUs, increased memory bandwidth, and enhanced support for large language models are among numerous significant enhancements made in NVIDIA H200 over its predecessor, NVIDIA H100. Additionally, it demonstrates superior performance, such as 1.4 times higher speed than that achieved using an H100.
Q: Why is it said that the NVIDIA H200 is the first-ever GPU with HBM3E?
A: The primary specification differentiating this new product from others may be summarized by saying —that NVIDIA’s latest release includes up to 141GB of fast ‘high-bandwidth memory,’ also known as ‘HBM3E’. This dramatically speeds up Artificial Intelligence working processes while improving overall system effectiveness!
Q: What are some areas where one can use an Nvidia h200?
A: They are perfect for applications such as generative AI, high-performance computing (HPC), large language models, and scientific computations, which all have an upper hand against even tensor core GPU Nvidia h100. These advanced features also make them widely adopted by cloud providers and data centers alike.
Q: Tell me about the benefits offered by the Nvidia h200 Tensor Core GPU.
A: Fourth-generation tensor cores, built on Nvidia hopper architecture, allow AI models to run faster on Nvidia h200 Tensor Core GPU than any other device used before or after it. This gives better energy utilization coupled with speed, hence making them ideal for heavier works, especially when integrated together with Nvidia h100 Tensor Core GPUs during processing complex loads.
Q: How does the NVIDIA H200 boost memory bandwidth?
A: It has 141GB of GPU memory and introduces new technologies like HBM3E, drastically improving memory bandwidth. This enables faster data access and better AI or HPC performance on heavy tasks.
Q: What can be expected regarding performance improvements with the NVIDIA H200 GPUs?
A: Users should see up to 1.4 times better performance compared to the prior generation NVIDIA H100 Tensor Core GPUs; this leads to faster workload completion and more efficient processing, particularly when using state-of-the-art NVIDIA GPUs.
Q: When will the NVIDIA H200 GPU become available?
A: According to an announcement by Nvidia, the H200 GPUs will be released in 2024. The exact date may vary depending on location and partnerships with cloud providers.
Q: Which systems will integrate NVIDIA H200 GPUs?
A: Various systems will incorporate these cards, including DGX and HGX H100 models from Nvidia themselves; such combinations aim to improve performance for artificial intelligence (AI) and high-performance computing (HPC) applications.
Q: How does the NVIDIA H200 architecture enable large language models?
A: It is built with large language models in mind, providing better efficiency, superior performance, and memory bandwidth over competing architectures. This makes it a great option for complex AI models and tasks.
Related Products:
-
NVIDIA MMA4Z00-NS400 Compatible 400G OSFP SR4 Flat Top PAM4 850nm 30m on OM3/50m on OM4 MTP/MPO-12 Multimode FEC Optical Transceiver Module $650.00
-
NVIDIA MMA4Z00-NS-FLT Compatible 800Gb/s Twin-port OSFP 2x400G SR8 PAM4 850nm 100m DOM Dual MPO-12 MMF Optical Transceiver Module $850.00
-
NVIDIA MMA4Z00-NS Compatible 800Gb/s Twin-port OSFP 2x400G SR8 PAM4 850nm 100m DOM Dual MPO-12 MMF Optical Transceiver Module $750.00
-
NVIDIA MMS4X00-NM Compatible 800Gb/s Twin-port OSFP 2x400G PAM4 1310nm 500m DOM Dual MTP/MPO-12 SMF Optical Transceiver Module $1100.00
-
NVIDIA MMS4X00-NM-FLT Compatible 800G Twin-port OSFP 2x400G Flat Top PAM4 1310nm 500m DOM Dual MTP/MPO-12 SMF Optical Transceiver Module $1200.00
-
NVIDIA MMS4X00-NS400 Compatible 400G OSFP DR4 Flat Top PAM4 1310nm MTP/MPO-12 500m SMF FEC Optical Transceiver Module $800.00
-
Mellanox MMA1T00-HS Compatible 200G Infiniband HDR QSFP56 SR4 850nm 100m MPO-12 APC OM3/OM4 FEC PAM4 Optical Transceiver Module $200.00
-
NVIDIA MFP7E10-N010 Compatible 10m (33ft) 8 Fibers Low Insertion Loss Female to Female MPO Trunk Cable Polarity B APC to APC LSZH Multimode OM3 50/125 $47.00
-
NVIDIA MCP7Y00-N003-FLT Compatible 3m (10ft) 800G Twin-port OSFP to 2x400G Flat Top OSFP InfiniBand NDR Breakout DAC $275.00
-
NVIDIA MCP7Y70-H002 Compatible 2m (7ft) 400G Twin-port 2x200G OSFP to 4x100G QSFP56 Passive Breakout Direct Attach Copper Cable $155.00
-
NVIDIA MCA4J80-N003-FTF Compatible 3m (10ft) 800G Twin-port 2x400G OSFP to 2x400G OSFP InfiniBand NDR Active Copper Cable, Flat top on one end and Finned top on other $600.00
-
NVIDIA MCP7Y10-N002 Compatible 2m (7ft) 800G InfiniBand NDR Twin-port OSFP to 2x400G QSFP112 Breakout DAC $200.00