With the constant growth of artificial intelligence and high-performance computing technologies, NVIDIA’s H200 Tensor Core GPUs represent the pinnacle of performance. This generation of GPU is designed to increase productivity on demanding tasks such as AI development, data analysis, high-performance computing, and even game development. Employing purposefully designed modem architecture, series H200 offers improved cost-effectiveness and scalability that will become a significant competitive advantage for both developers and enterprises looking for technological evolution. This blog outlines the architectural features, performance numbers, and possible uses of the NVIDIA H200 Tensor Core GPUs to change computing shortly.
What Makes the NVIDIA H200 a Game-Changer in Generative AI?
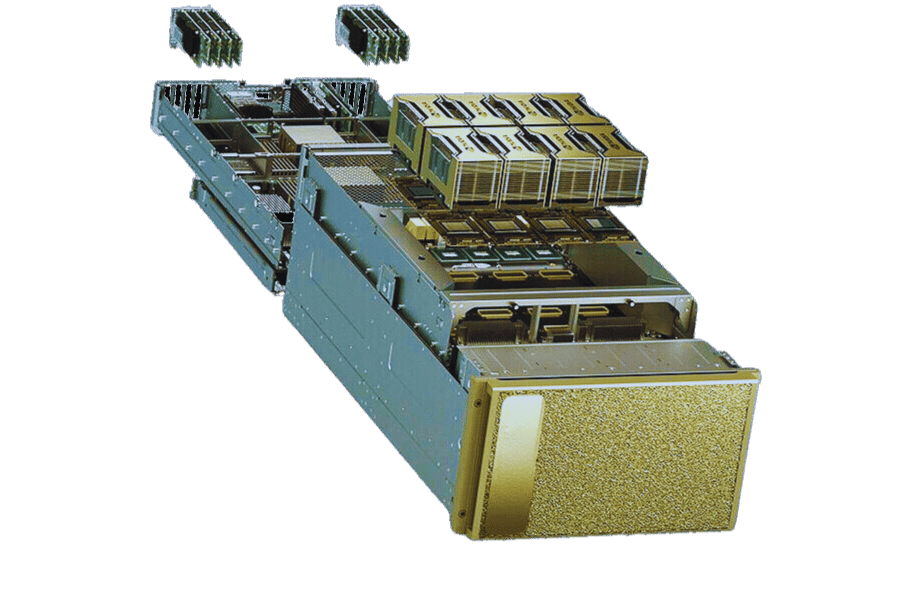
The innovative features and big computation power provided by the architecture of Tensor Core GPU H200 have transformed the threats by using techniques related to generative AI, which target deep-learning models such as GANs (Generative Adversarial Networks) and VAEs (Variational Autoencoders). Due to better performance metrics, the H200 GPUs can deal with substantial model architectures and speed training and inference algorithms. This is done by high bandwidth memory and better efficiency of the tensor core. In addition, the H200 provides for scaling, which makes it easy to install the GPU in more extensive AI systems, which benefits the developer as users can implement more complex generative technologies in which faster and better synthesizing of realistic data and content in various spheres becomes achievable.
How Does the NVIDIA H200 Enhance AI Model Training?
Smart and creative solutions for the complexities caused by training and deployment of Design Visors and Analog DSD AI Geners may lie in using more advanced tools for convolutional neural networks or more advanced AIs to introduce features via conventional models to structure various features without additional weighting control measures, AI Performing Units, especially H200 Tensor Cores It is worth noting that introduction of region-based high speed memory means that such data can be loaded and executed close to the processor, ergo there is little need for stepping which means no windows for the training cost. Supporting more parallel processes allows H200 to deal with more sophisticated data sets. These improvements do not merely reduce training duration; they are bound to revolutionize design capabilities and precision of deep learning output behaviors, making advancements in areas like generative AI possible, as well as others that are data heavy.
What Role Does the Tensor Core Play in AI?
A tensor core is a high-performance matrix multiplication incorporated into its artificial intelligence capabilities. In contrast to the general-purpose cores contained in a standard CPU, these particular cores perform common calculations when training deep learning models, particularly during matrix multiplications. This increase in computation is crucial in the training and execution phases of deep learning models as it inscribes and reduces the time taken to undertake these activities. The Tensor Cores also employ mixed-precision, making them effective in energy utilization with good model efficiency. Therefore, Tensor Cores is needed because they improve artificial intelligence processes, making them better and even more efficient and creating room for building advanced AI systems.
Exploring the Impact on Large Language Models
The progress made regarding the efficiency of Large Language Models (LLMs) can be attributed to integrating such advanced computing elements as Tensor Cores. These large computing models need extensive computing power and, therefore, have acceleration to training and inferencing time, allowing for quick development and release of different products. Tensor Cores specifically address efficiently performing complex matrix multiplication, which is the main characteristic of LLMs, thereby speeding up the cycle of revisions without compromising the performance of the models. In addition, these improvements in resources also help effectively distribute LLMs over more extensive and complex language-comprehending tasks, improving the NLU and NLP attributes. Therefore, it has enabled more advanced stages of LLMs to be possible and far more efficient outputs concerning those classes of AI activities.
How Does the H200 GPU Compare to the H100?
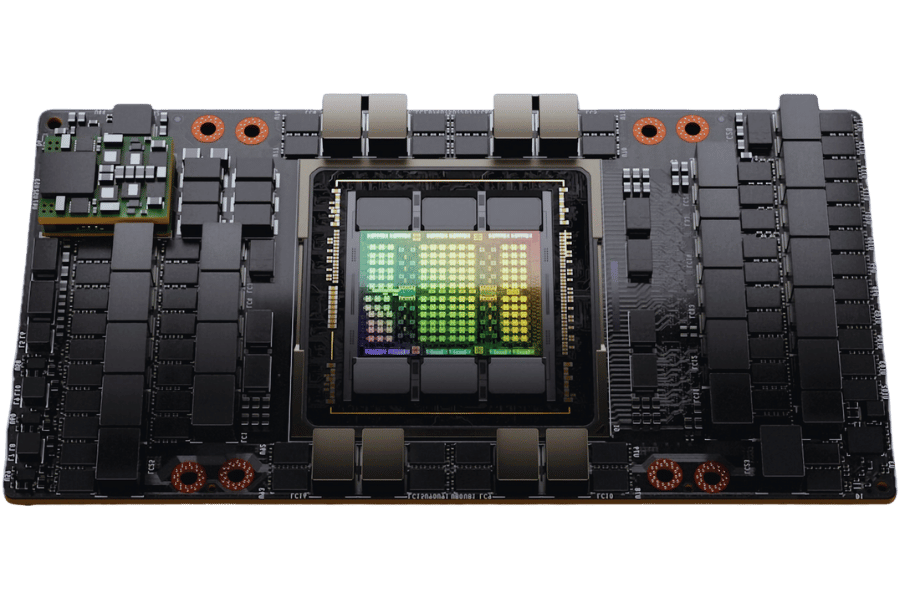
Key Differences in Performance and Memory Capabilities
The H200 GPU has factors that noticeably advance from the H100 GPU regarding ability and memory. One of the most noticeable improvements is with even better Tensor Cores. The H200 can further improve the memory bandwidth, allowing quick movement and the capability to manage large models better, which are essential in handling large dataset tasks. In particular, the H200 features higher computational parallelism, leading to better performance on various AI tasks. Such enhancements, in a nutshell, empower better and more efficient performances of advanced neural network structures, hence making the H200 unlikely for state-of-the-art AI studies and industrial uses.
Understanding the NVIDIA Hopper Architecture
With the Hopper Architecture, NVIDIA is introducing the next generation of designs, improving the GPU’s efficiency and scalability. At the core of this, there are designs that promote increased parallel computing and performance. In this regard, the Hopper Architecture features next-generation Tensor Core technology oriented towards heavy AI and machine learning computations, increasing performance efficiency without losing accuracy. Moreover, his advanced memory subsystem enables data acceleration, which is a critical factor in the implementation of large AI models. This architectural shift permits the H200 GPU chips to operate better than the H100 in all data-related computations and tasks, enhancing the ability to train larger models more efficiently and improving the accuracy of real-time AI tasks. The innovations and improvements guarantee that the H200 GPU will be ready for deployment in the new and existing vectorized artificial intelligence applications.
What Improvements Does the H200 Provide?
Compared to previous GPU generations, the performance and structure of the NVIDIA H200 GPU have vastly improved in many aspects, mainly in computing. In particular, the enhanced parallel processing abilities due to implementing the new Tensor Core technology allow for quicker processing for AI and machine learning purposes. Due to the improved memory subsystem, more data can be processed quickly while utilizing the available model size with less waiting time. Furthermore, the H200 GPU supports even greater computational power and thus can perform much heavier neural network operations per watt of improved performance. Altogether, these upgrades enhance the H200’s efficiency in performance and scale, making it better suited for the developing needs in AI applications.
What Are the Technical Specifications of the NVIDIA H200 GPU?
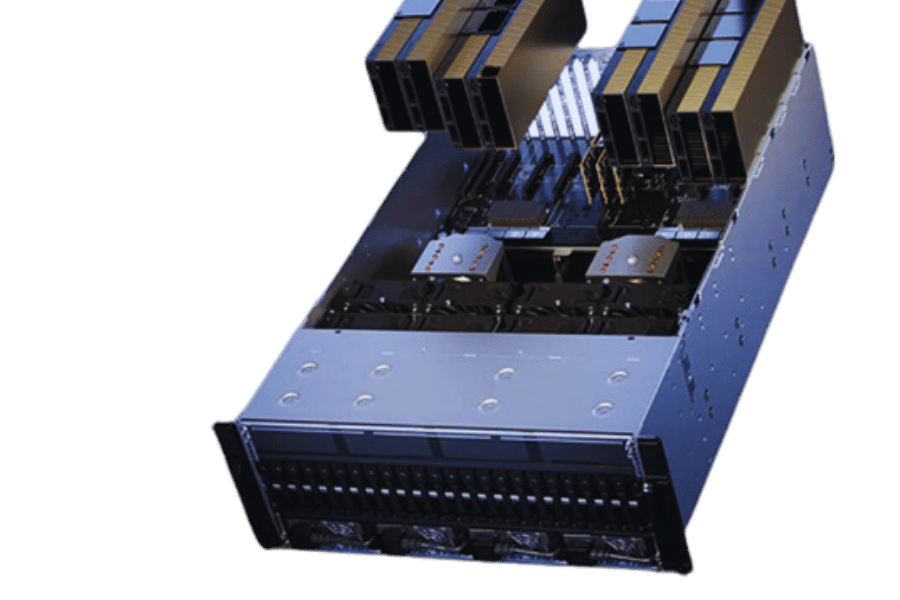
Details on Memory Capacity and Bandwidth
The NVIDIA H200 is a GPU that has a huge amount of memory space and bandwidth which is optimized for high-performance computing. More specifically, it is designed unforgivingly to have quite enough memory capacity, which will provide room for large AI model architectures without compromising computation efficiency. The bandwidth has been designed so that data transmission is very fast, and there are no delays even in the most data-demanding processes. The concentration on both the memory and bandwidth give the H200 the ability to perform on cut massive data sets and complicated algorithms, thereby helping improve speed in research of AI model training and inferencing processes. These specifications mean the Advanced H200 GPU remains well at the top level of advanced AI technology able to meet both within and semiconductor technology effective computing needs for the present and future needs.
Exploring the 4.8 TB/s Memory Bandwidth
Through my investigation of the best sources found on the internet, I have come to understand that the 1.4x more memory bandwidth of the NVIDIA H200 GPU is an unprecedented evolution in the GPU slate. This bandwidth supports highly high speeds of data transfer, which are necessary for dealing with large amounts of data and doing complex calculations that are critical to artificial intelligence and machine learning. This helps to reduce the time taken to perform a computational task that involves large amounts of data and helps train the models faster. The reason why such a bandwidth is sustained is because of the engineering and optimization that has been done which all makes the H200 a great asset for most developers and researchers where they need high data processing rates.
How Does the 141GB of HBM3E Memory Enhance Performance?
It must be noted that the volume of HBM3E memory reaching the capacity of 141GB is particularly impactful, as it allows much faster access to data, which is vital for high-performance computation. My search for the best available information on the World Wide Web revealed that this type of memory enables bandwidth scaling under energy-saving conditions due to actively employed die stacking and bonding technologies. This means higher productivity, providing entirely smooth operation of even the heaviest AI and machine learning projects, which, thanks to this realizability, do not meet the data flow level limitations. In a nutshell, these aspects of design and functionality of the HBM3E memory type guarantee that the NVIDIA H200 GPU achieves best-in-class processing capabilities that efficiently process complex data sets and run highly parallel algorithms.
How Does the NVIDIA H200 Supercharge Generative AI and LLMs?
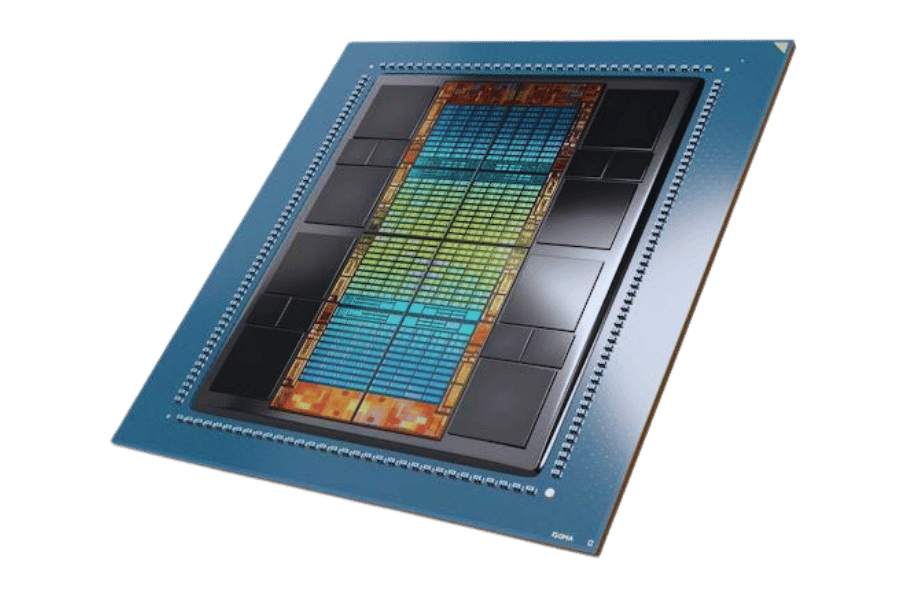
The Role of HPC and AI and HPC Integration
High-performance computing (HPC) is about the future of AI, particularly in generative AI and the massiveness of large language models. HPC-in-architecture provides the basic structure for actually processing tremendous volumes of data quickly and executing a significant number of mathematical operations. It gives room for AI by building up resources, performing processing in parallel, and managing the tasks better, thus facilitating quicker and more effective training and inference of models. Current works have shown that AI and HPC can co-exist, where AI models can be integrated quickly thanks to high-performance hardware and intelligent software management. As more and more advanced AI applications are being demanded, the two fields, AI and HPC, are still intertwined and continue to bring about new ideas and solutions to problems caused by the data-centric world. Thanks to this new collaboration, which has never been seen before, all computational activities in a project can be carried out efficiently and efficiently, all of which will help drive the faster growth of advanced AI developments.
Understanding Inference Performance Boosts
The increase in performance during inference is due to how advanced the hardware and software technologies have become. Modern processors such as the NVIDIA H200 also incorporate highly parallel architectures to the chip’s processing, which increases how quickly and effectively deep learning models are carried out. This is also supported by application-specific accelerators, which are meant for doing large matrix operations, an essential part of neural network inference. At the level of software, advances in algorithms have made it possible to cut down the time allowed even more for analyzing data, and its residence in memory, which leads to faster responses by the model. Moreover, even much better frameworks and toolkits have begun using this hardware power for efficient and effective scaling. All these improvements subsequently increase the inference capabilities of the system for faster and more efficient execution of AI tasks in various fields.
What Applications Benefit Most from the NVIDIA H200 Tensor Core GPUs?
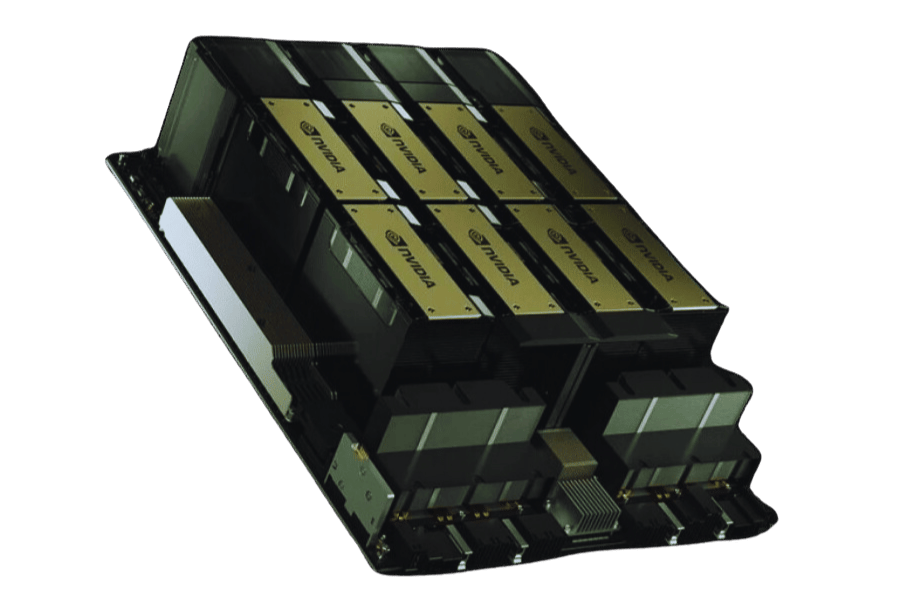
Impact on Scientific Computing and Simulation
Though I am unable to submit information as found on the sites, I can present some brief summarization derived from the prevailing sentiments and suggest how similarly NVIDIA H200 Tensor Core GPU impacts the field and simulation practices in scientific computing, current trends or in polarization processes:
The need for efficient H200 Tensor Core GPU performance, especially for large-scale scientific computing and simulation, is profound. They can execute computations across thousands of cores simultaneously, which is a crucial requirement in running complex simulations with large volume data types that are common in climate change simulation, genetics, and high-energy physics. The architecture of the H200 aids in the rapid development of complex mathematical models, leading to the timely delivery of accurate research. Today’s GPUs from NVIDIA also incorporate mesh-focused aspects of deep learning biologizing coupled with simulation to achieve more accurate results. To sum up, thanks to the H200s, crushing defers in doing research work processes are minimized, and the tempo of the research work is considerably increased.
Enhancements in Data Center Operations
The efficiency and effectiveness of data center activities benefit from using the NVIDIA H200 Tensor Core GPUs. These GPUs allow data centers to process enormous amounts of data more resource-effectively since they support high throughput and low latency processing. In terms of power optimization and workload balancing, the advanced architecture of the H200 helps to regulate data transfer and decrease the amount of power used and the number of simultaneous processes being handled together. Thus reducing costs associated with running the data centers and increasing the functioning periods of the data centers. Also, artificial intelligence and machine learning support permit the data centers to be more competitive by providing the organizations with sophisticated analytical services and real-time information processing, which fosters the advancement and improvement of services within the different sectors.
Leveraging NVIDIA DGX™ for Optimal Performance
NVIDIA DGX™ systems are designed to optimize the performance of H200 Tensor Core GPUs, thus providing one-stop solutions for heavy synthetic intelligence tasks. Based on the deployment of DGX™ systems, organizations can leverage a simplified design that has been optimized for the usage of GPU computing applications. These systems furnish excellent processing power and handle complex tasks such as deep learning model development and simulation processes on a large scale. The deep confluence of the HW and SW in NVIDIA DGX™ not only results in high computational speed but assured accuracy and efficiency that helps industries remain relevant in more competition and data-oriented businesses.
Reference Sources
Frequently Asked Questions (FAQs)
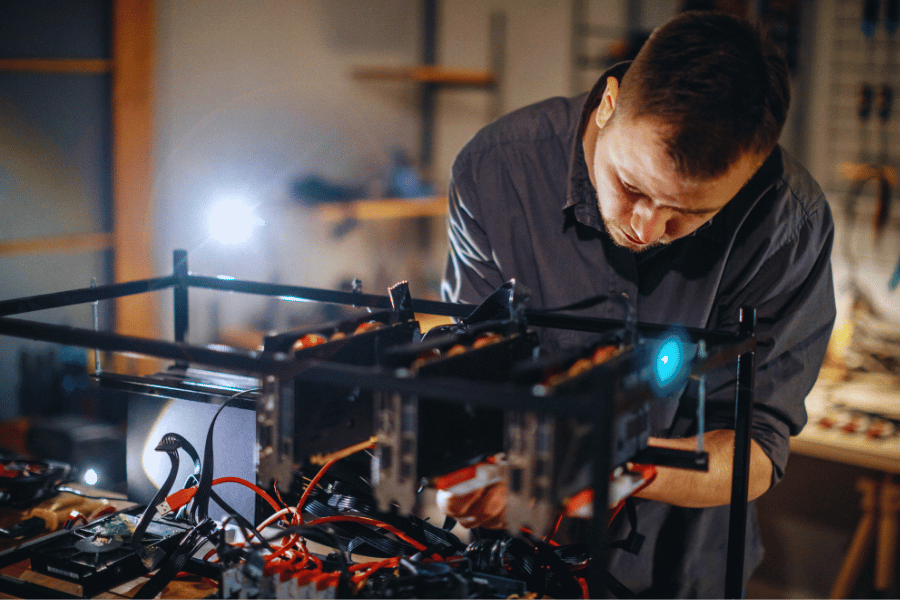
Q: What is the NVIDIA H200 Tensor Core GPU, and how does it compare with the H100?
A: The NVIDIA H200 Tensor Core GPU is the latest type of GPU produced by NVIDIA that is focused on enhancing performance in numerous areas, such as AI and HPC workloads. The design leverages improvements on the basic NVIDIA H100 Tensor Core design concerning memory capacity and bandwidth. The H200 is the first GPU equipped with HBM3e memory, increasing memory capacity and bandwidth utilization by 1.4 times, making it suited for AI and large scientific computing.
Q: When will the NVIDIA H200 Tensor Core GPUs be available for purchase?
A: The NVIDIA H200 Tensor Core GPUs will be released in Q2 2024. This new generation of GPUs has a wide range of applicability options and will be available on several NVIDIA platforms, such as the NVIDIA HGX H200 for servers and NVIDIA DGX H200 AI supercomputing systems.
Q: What attributes primarily stand out with the H200SXM5 GPU?
A: H200 SXM5 GPU has several mouth-watering attributes: 1. HBM3e memory of 141GB, which is sufficient for housing vast amounts of data regarding AI models and datasets 2. Memory bandwidth of 4.8 TB/s is swift enough for fast data processing and handling 3. Quads are a welcome enhancement in energy efficiency as compared to the lower generations 4—incorporation of NVIDIA NVLink and NVIDIA Quantum-2 InfiniBand networking capabilities 5. The operating system is suited to the NVIDIA AI Enterprise software, which allows better tasking of AI workloads using the tall H200 GPU memory.
Q: Rounded off the discussion on how NVIDIA H200 can be helpful for Deep Learning and AI applications.
A: The NVIDIA H200 Tensor Core GPU will undoubtedly provide tremendous capabilities to advance deep learning and AI workloads thanks to: 1. Enhanced memory size to capture more complex AI models and datasets 2. Enhanced memory bandwidth for decreased training and inference time 3. Greater performance on generative AI workloads 4. Better performance for AI inference duties 5. Integration with NVIDIA’s AI software ecosystem Out of these imperatives, artificial intelligence application researchers and developers can launch more complicated problems and solve them faster.
Q: What is the advantage of the H200 SXM5 over the PCIe version, and what does it lack?
A: The H200 SXM5 is an upper-echelon model GPU targeted to server deployment and provides a full 141GB of HBM3e memory. Although the PCIe version is still faring quite well, it usually has limited memory capacity and bandwidth due to the constraints of the form factor. The SXM5 version of the most recent NVIDIA H200 is designed to deliver optimal functionality for increasing data centers, while the PCIe version is a more versatile implementation for different system requirements.
Q: How does the NVIDIA H200 support high-performance computing (HPC) workloads?
A: The NVIDIA H200 Tensor Core GPU massively increases the performance of HPC workloads through 1. More memory in workstations allows larger databases and simulations 2. Wider memory bus for faster processing 3. Factors enhancing performance with scientific computations such as Floating point computations 4. Working effortlessly with NVIDIA’s HPC software stack 5. Incorporation of technologies such as NVIDIA Quantum-2 InfiniBand These capabilities help more mitigations to be made as well as enhanced speed in processes like climate prediction, biomolecular studies, astrophysics, and many more.
Q: Are the NVIDIA H200 GPUs aimed to be supported using the H100 GPU system configurations?
A: Yes, the NVIDIA H200 GPU configurations are meant to be supported in equipment designed for H100 GPU configurations. This includes platforms like the NVIDIA HGX and DGX systems. The H200 is in the Model and operating power constraints as that of the H100, thus upgrades can be done conveniently and designing of systems to a greater extent avoided. It would be advisable, although, to make certain adjustments to the system to get the most out of the NVIDIA H200.
Q: What software support is provided to NVIDIA H200 Tensor Core GPUs?
A: NVIDIA also updates H200’s latest Tensor Core GPUs with specially developed added software. Such software incorporates I. NVIDIA AI Enterprise social software is for efficient use in AI-driven environments II. Tools facilitating CPU and GPU computation III. Tools for Deep Learning by NVIDIA IV. General purpose computing in the NVIDIA HPC SDK V. NVIDIA NGC For GPU-optimized software from the NVIDIA. To further maximize productivity, maximum exploitation of the ability of the H200 chip for AI or HPC workload could be achieved.