In today’s technological world, there is a growing need for greater computing power and intelligent processing abilities. The NVIDIA GH200 with the Grace Hopper Superchip is an AI supercomputer representing a massive step forward in this area. This paper will explore why the GH200 will change what we consider standard for artificial intelligence and machine learning by having never before seen performance levels coupled with efficiency gains. This article breaks down each component, too, from its cutting-edge design down to how they combined GPU and CPU capabilities like never before so that you can understand just how much of an impact it could have on different industries. Join us while we review technical specifications, potential uses, and what might happen next with this game-changing technology!
What is the NVIDIA GH200 and Why is it Revolutionary?
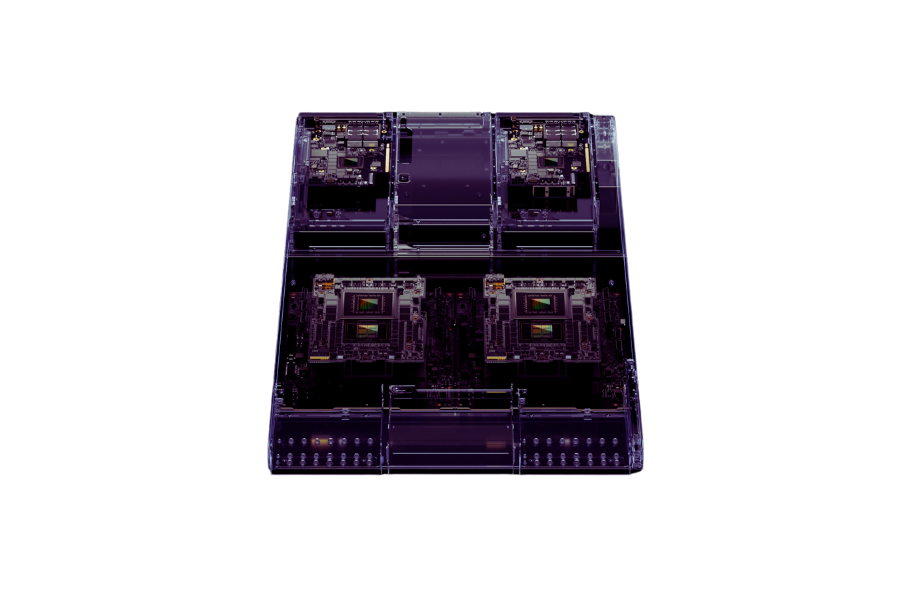
Understanding the GH200 Grace Hopper Superchips
A combined computing solution is the NVIDIA GH200 Grace Hopper Superchip, which integrates the powerful features of NVIDIA Hopper GPU architecture and the performance efficiency of the ARM Neoverse CPU. It has been made possible for this chip to provide unprecedented levels of AI, HPC (High-Performance Computing), and data analytics performance by such incorporation. This GH200 is different from other chips because it can blend GPU and CPU components seamlessly, thereby reducing latency while increasing data throughput so that complex computational tasks are processed more efficiently, leading to new frontiers in AI-driven applications across multiple industries such as scientific research; self-driving machines; and big data handling among others.
Key Features of the NVIDIA GH200
- Merged GPU and CPU Design: The GH200 model combines the NVIDIA Hopper GPU with the ARM Neoverse CPU to create a unifying system that reduces delays and increases the data transfer rate. In addition, NVIDIA has also optimized this platform for different high-performance computing tasks.
- Supercomputer Chip (HPC): This super chip is designed for intensive computational tasks, making it best suited for environments that require high-performance computing capability.
- Better Data Efficiency: The GH200 integrates processing units with memory, therefore improving data transmission speeds and leading to more efficient data processing.
- Scalability: Whether it is scientific research or autonomous systems, the GH200’s design ensures that it can be scaled up or down according to various industry needs.
- Energy Saving: GH200 provides high performance through ARM Neoverse architecture while still being energy efficient, hence important for sustainable computing solutions.
- Supporting AI and Machine Learning: These advanced features in GH200 allow complex artificial intelligence and machine learning models, thus promoting innovation in AI-driven applications.
Grace Hopper™ Architecture Explained
The architecture of Grace Hopper is a revolutionary method for computing systems that combines the power of NVIDIA’s Hopper GPU Architecture with ARM Neoverse CPU Architecture. This union reduces latency in data transfer and increases throughput or the amount of useful work done. The design has fast shared memories, integrates CPU and GPU workflows seamlessly, and employs advanced interconnects, which are necessary for supporting massive data processing requirements.
Some important features of the Grace Hopper architecture are:
- Unified Memory: It allows the CPU and GPU to access a common memory pool, which greatly cuts down on data transfer times, thus making computations more efficient.
- Advanced Interconnects: This type of technology uses technologies like NVIDIA NVLink, among others, that have very high bandwidths. This enables quick communication between various parts, thereby ensuring the best possible performance for tasks involving large amounts of information.
- Parallel Processing Capabilities: The system’s computational ability is boosted by integrating NVIDIA Grace CPUs with HBM3E memory; this significantly improves its efficiency, too, since it can process many things at once. In addition, this architecture performs exceptionally well in parallel processing, hence being highly suited for AI model training, high-performance computing tasks, and complex simulations in general.
In summary, the Grace Hopper™ Architecture was created to address current needs in computing environments by providing a scalable, efficient, and high-performance foundation for different applications.
How Does the NVIDIA DGX GH200 AI Supercomputer Work?
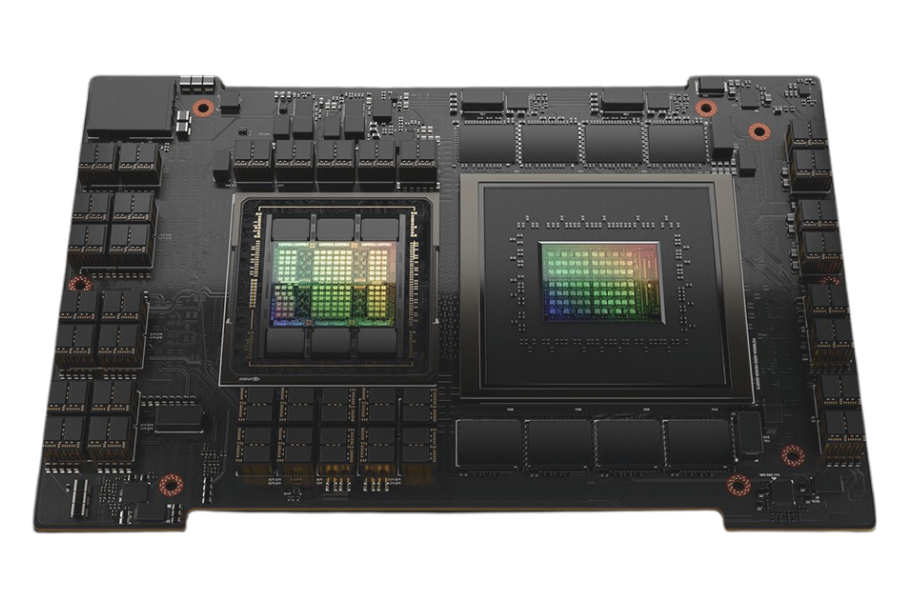
The Role of GPUs and CPUs in the DGX GH200
The AI supercomputer NVIDIA DGX GH200 combines CPUs and GPUs to achieve computing power of never-before-seen sizes. In parallel processing, GPUs or Graphics Processing Units play a fundamental part by dealing with multiple operations at once; this is crucial while training large-scale AI models and performing complex simulations since they are exceptionally good at managing huge amounts of data along with computations running in parallel.
On the other hand, CPUs (central processing units) are responsible for managing general-purpose computing tasks and coordinating activities among different parts within an AI supercomputer. Sequential task computation support for them comes from integrating ARM Neoverse CPUs into DGX GH200 so that they can handle flow control management efficiency systems overall.
ARM Neoverse CPUs integrated into DGX GH200 work together with NVIDIA’s Hopper GPU in such a way that data-intensive AI applications can take advantage of increased bandwidths and reduced latencies, among others, while still enjoying better performance levels than before. This enables scalability and efficiency when dealing with heavy workloads required by demanding artificial intelligence systems, thus making it possible for robust solutions to be provided by DGX GH200 under such circumstances.
NVIDIA NVLink: Enhancing Interconnectivity
NVIDIA NVLink is an interconnect technology with high bandwidth that boosts data exchange between NVIDIA graphic processing units and central processing units. It decreases latency by providing a direct communication path, hence maximizing the rates at which information can be transferred, thus improving the efficiency of any workflow done on platforms such as NVIDIA DGX H100. NVLink technology enhances scalability by allowing multiple GPUs to seamlessly work together and share their resources for handling complicated AI models as well as data-intensive applications. This feature enables such AI supercomputers like DGX GH200 to scale up in performance because they can deliver more than what traditional architectures based on slow speed and inefficient methods of transferring data would have achieved. Within DGX GH200, this interconnect ensures there are no delays in moving data between processors, thereby enabling real-time processing and analysis of large volumes of information.
Accelerating Deep Learning and AI Workloads
In order to speed up deep learning and AI workloads, DGX GH200 uses advanced hardware and optimized software, including NVIDIA Grace Hopper Superchip. The NVIDIA Hopper GPU integration gives unmatched computational power, hence enabling faster training times and better inference rates on complex models. Additionally, this comes with high-speed storage coupled along side single memory that ensures quick data retrieval as well as processing speeds. Another thing is that the utilization of NVIDIA CUDA, together with cuDNN libraries, simplifies the development process, thus giving developers efficient tools for implementing and deploying AI applications. All these advancements make it possible for DGX GH200 to deliver higher performance levels, thereby meeting the general demands posed by modern AI workloads.
Why Choose NVIDIA GH200 for Your AI Workloads?
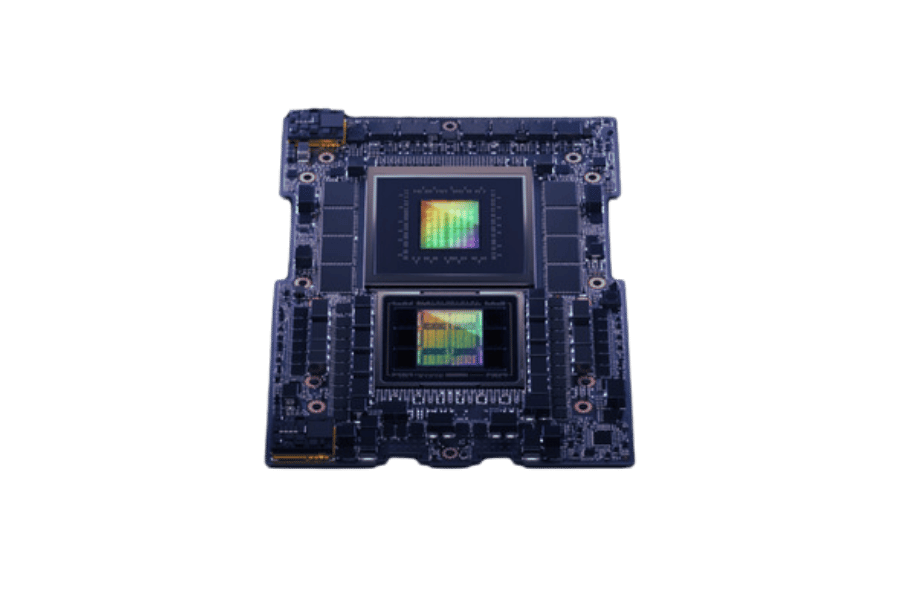
Advantages of High Bandwidth Memory (HBM3E)
The NVIDIA Grace Hopper Superchip platform is an effective way to take advantage of these benefits. That being said, there are many reasons why HBM3E should be used in high-performance AI supercomputers such as the DGX GH200. Some of the most notable ones include its compact design, which reduces the distance data needs to travel, thus preventing any potential bottlenecks from occurring; it acts as a critical component for faster model training and more efficient inference processes that are necessary for dealing with large amounts of information at once; and lastly but not least importantly, this technology offers exceptional energy efficiency which plays a significant role in managing thermal & power budgets of advanced AI systems so they can perform optimally without overheating or using too much electricity.
Leveraging NVIDIA Grace Hopper Superchips
The NVIDIA Grace Hopper Superchips are a novel advancement in AI and high-performance computing. These Superchips combine the power of the Hopper GPU architecture from NVIDIA with the abilities of an advanced Grace CPU, creating a single system that shines in both computation-heavy and memory-heavy workloads. The parallelism of Hopper GPUS and higher bandwidth memory subsystems found in Grace CPUs accelerate AI model training time while allowing for real-time inferences hence making use of NVIDIA Grace Hopper Superchip technology possible. This blend also supports heterogeneous computing, i.e., seamlessly managing different types of computational tasks on one infrastructure. In addition, sustainable performance is guaranteed by the energy-efficient design of these chips, which conforms to more eco-friendly IT solutions due to the increased need for them resource-wise as well as other aspects. Through such means, enterprises can greatly improve their AI capabilities, achieving better results faster at lower costs per operation.
Maximizing Performance for Generative AI
To maximize generative AI performance, deploy state-of-the-art hardware and software optimization techniques that enable vital model training and inference. Some of the top methods are as follows:
- Use Specialized Hardware: Use advanced hardware like NVIDIA Grace Hopper Superchips, which have high-performance GPUs coupled with effective memory systems capable of delivering the required processing power for generative AI tasks. This integration is useful in handling compute-intensive workloads that require more memory simultaneously.
- Implement Parallel Processing: Exploit parallel processing using GPUs to reduce training times during complex execution of generative models. Mixed-precision training, among other optimization techniques, can achieve these computational efficiencies without compromising accuracy.
- Optimize Model Architecture: Better results can be obtained by reducing parameters through streamlining model architectures, while pruning or quantization techniques may also be employed where necessary without degrading quality. Advanced neural network frameworks allow for such optimizations, thus enabling real-time deployment on NVIDIA DGX H100 platforms.
With these guidelines, businesses will be able to realize better-performing systems in terms of speed and quality thanks to faster iterations brought about by more refined outputs generated from competing against each other in an increasingly aggressive market environment utilizing creative AI.
How is NVIDIA GH200 Different from NVIDIA H100 and A100?
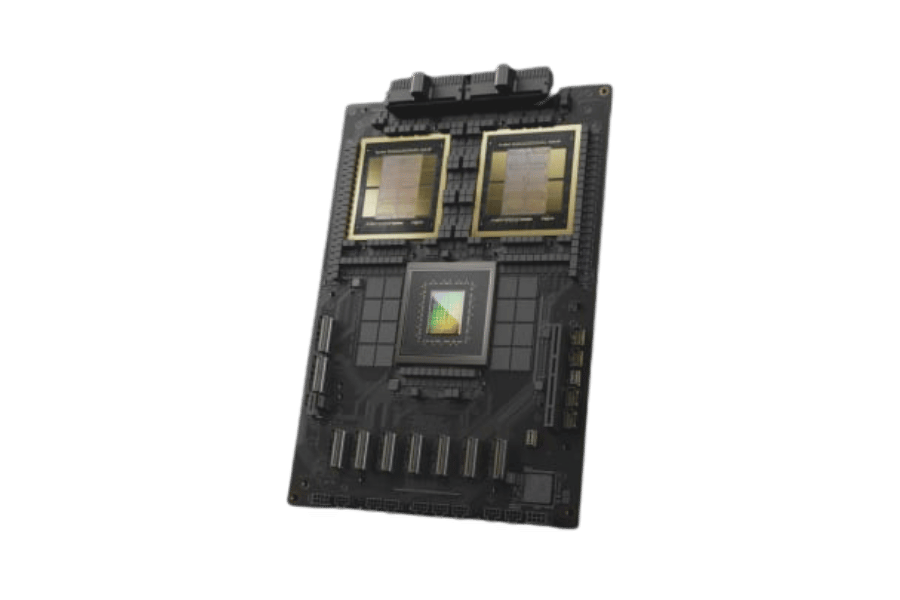
Comparative Analysis with NVIDIA H100
The NVIDIA GH200 and H100 differ greatly in their architecture and performance capabilities. For example, the GH200 uses NVIDIA Grace CPUs which are designed with higher performance in mind. On the other hand, built on Grace Hopper architecture by Nvidia, this chip combines high-performance GPUs and an advanced memory subsystem for better handling of large-scale generative AI workloads. More memory bandwidth, as well as the capacity of storage within the GH200, leads to increased speed and efficiency during data-intensive operations such as training or inferring from generative models.
In comparison to its counterpart based on Hopper Architecture–H100, although it is optimized for various types of accelerated computing workloads including but not limited to Artificial Intelligence (AI) tasks and High-Performance Computing(HPC), it is a lack of integrated memory systems as found in the GH200 model. However, what differentiates between them mostly lies in their memory organization units, whereby much more improvements can be witnessed in terms of parallelism processing capabilities within GH 1000 than any other device currently available today.
While both these designs represent cutting-edge technology advancements within this field, there still exist certain unique features only exhibited by GH200, like hopper GPU combined with grace CPU, which makes it a complete system best suited towards addressing challenges posed by generative AI programs. In particular, this implies that whenever an application needs high levels of computation power coupled with efficient data management strategies then selecting gh 200 will never disappoint.
Performance Differences between GH200 and A100
According to this statement, disparities in performance between NVIDIA GH200 and A100 are mainly caused by architecture and memory capabilities. Grace Hopper is the most recent architecture used in GH200, which has significantly increased computer power and improved memory bandwidth compared to A100, which uses Ampere architecture. This means that the integrated memory subsystem of GH200 is more beneficial for AI systems and other data-intensive applications since it provides higher throughput with greater efficiency.
On the contrary, although A100, based on Ampere architecture, offers excellent performance across various artificial intelligence (AI) and high-performance computing (HPC) applications, it lacks some specialized enhancements found in GH200. Additionally, there are several precision modes in A100 that can be scaled according to different workloads, but its level of memory integration and parallel processing capability is not similar to that of GH200.
To sum up, while each GPU performs well within its domain; it’s clear from this passage that what sets apart GH200 from others is its advanced architectural design that makes these cards best suited for generative AI loads requiring massive memory handling abilities coupled with computational might.
Use Cases for GH200 vs. H100 vs. A100
GH200:
The GH200 is great for generative AI workloads that take up a lot of memory and processing power. It is designed to handle deep learning training, large language models, and complex simulations. Very few applications can beat the GH200’s wide memory bandwidth and integrated memory subsystem when it comes to working with massive data sets; this allows for quicker data manipulation and optimal model training.
H100:
Utilizing Hopper architecture, the H100 was created as a tool for high-performance computing (HPC), AI inference, and deep learning. It works well in situations where there is a need for significant amounts of computation alongside low latency, like real-time analytics on scientific research or autonomous systems. Its ability to provide high throughput at data centers while still maintaining fast inferencing capabilities makes it an excellent choice across multiple different types of AI applications.
A100:
Built around Ampere architecture, the A100 can be used in many different kinds of artificial intelligence (AI) workloads as well as high-performance computing (HPC). Amongst mainstream machine learning, traditional HPC workloads and data analytics would benefit from their use alone or combined with other hardware such as CPUs or GPUs. A100 supports multiple precision modes, which means that functions like training small-medium neural networks may run faster on this chip compared to others; additionally, inference performance scales better when more diverse computing tasks are performed simultaneously using all available resources within one system. Although lacking specialized enhancements found within GH200 units – A100s remain solid performers across general AI & HPC domains.
What Are the Potential Applications of NVIDIA DGX GH200?
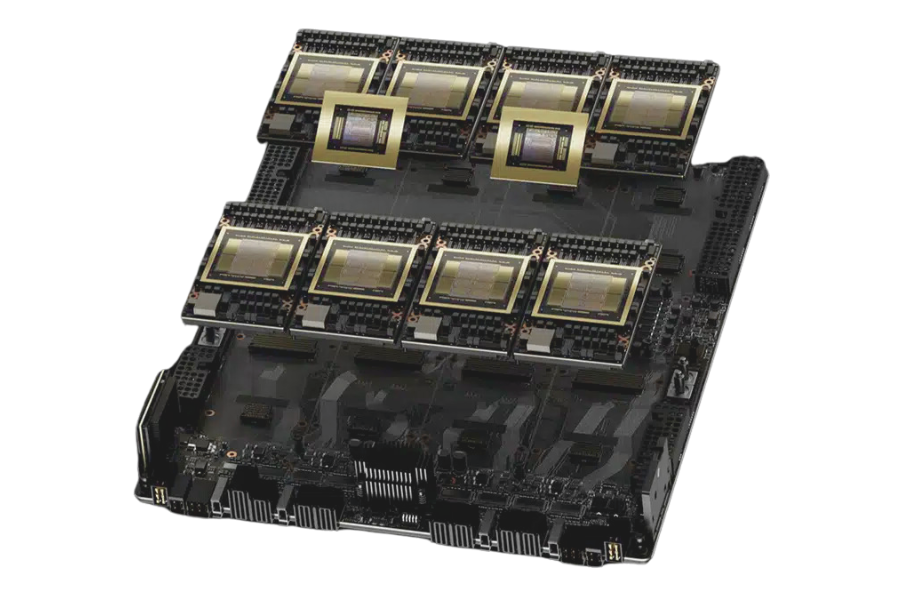
Revolutionizing Data Centers with GH200
The DGX GH200 of NVIDIA changes the game for data centers through its unmatched performance and scalability when handling AI workloads. It helps them process huge datasets faster than before, which is helpful with tasks such as training deep learning models, running large-scale simulations, or real-time information processing. This is especially important in sectors like health care, finance, and autonomous systems, where vast amounts of data must be processed quickly and accurately.
Among the many benefits offered by GH200 is integration with NVIDIA’s Grace Hopper Superchip, which provides extraordinary memory bandwidth and computing power. With this feature incorporated into their infrastructure, organizations can run complex AI models more efficiently while also creating higher-level AI applications. Also, GH200 has an architecture that allows for great scalability, so resources can be added as required without disrupting operations.
What’s more, GH200 can handle an array of different works, from scientific research up to AI-powered applications, making it one versatile component within today’s data centers. Besides lower operational costs due to improved performance and efficiency, there are also other long-term savings since these things will continue evolving, thus future-proofing against changing technology needs but still always ensuring high throughput capacity.
AI Supercomputers in HPC and AI Models
Supercomputers of artificial intelligence are leading the field of high-performance computing and AI models that drive innovation across various domains. These capabilities combine AI with HPC in systems such as the NVIDIA DGX GH200 to solve difficult computational problems thereby enabling scientific breakthroughs and industrial applications.
AI supercomputers work better with massive datasets because they use state-of-the-art hardware and software architectures for fast, accurate results in AI and deep learning tasks. As a result, researchers can train large models more quickly, shorten development cycles, and get insights faster. In addition, parallel processing is one area where AI supercomputers excel by optimizing simulation performance and large-scale modeling project speed.
Notably, climate modeling would not have reached its current level without integrating AI supercomputers into the HPC environment, according to websites like IBM or Top500.org, which also talk about genomic research, drug discovery, and financial modeling. These machines have immense processing power needed for handling huge volumes of data sets, driving new ideas into algorithms used for artificial intelligence, and fostering future generations of such programs. Thanks to their exceptional computing abilities coupled with increased memory bandwidths, these devices provide strong yet scalable solutions capable of meeting any dynamic requirements posed by HPC along with AI models.
Future Prospects in Accelerated Computing
Continuous architecture, hardware, and software innovation will significantly advance the future of high-speed computing. As indicated by NVIDIA, Intel, Microsoft, and other leading sources, AI integration with HPCs is expected to bring about even more radical changes in different sectors. They also report that GPU progression is not over yet but still continuing to take place which will see complex artificial intelligence models developed alongside simulations due to increased performance levels. Quantum computing, according to Intel, can solve problems that were previously unsolvable while creating new limits for computational power through the use of neuromorphic architectures.
These developments collectively imply that data processing efficiency will be improved and computation time reduced, thus driving inventions within such fields as self-driving car systems, personalized medicine, and climate science mitigation research, among others. Additionally, future outlooks on energy-saving methods should also consider catering to environmentally friendly technologies since this will help meet the ever-increasing demand for sustainable development in different areas related to quickening computations while maintaining balance throughout their growth process according to energy conservation.
How to Implement the GH200 Grace Hopper Superchip Platform?
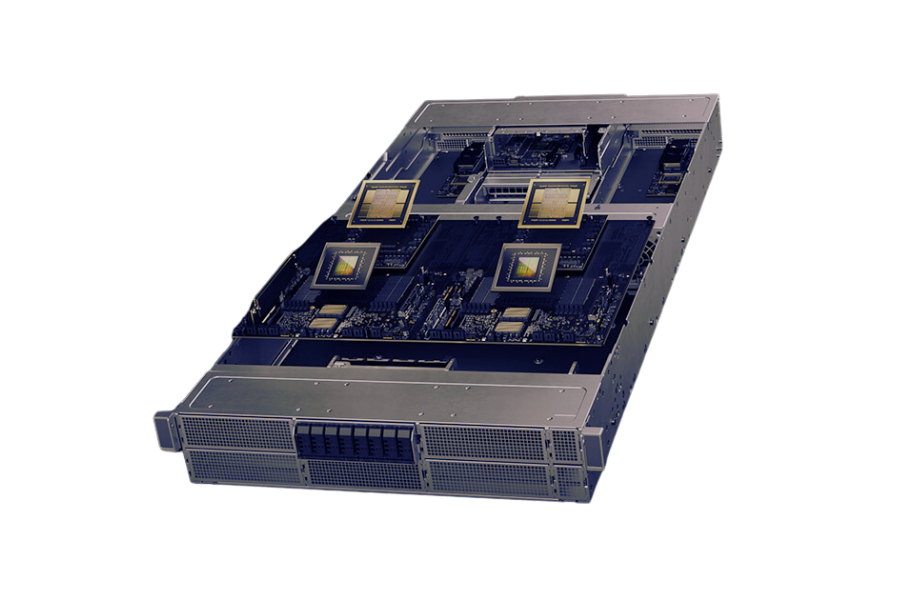
Setting Up NVIDIA GH200 in Your Cluster
Multiple steps are required to set up the NVIDIA GH200 driver in your cluster, beginning with hardware installation and concluding with software configuration and optimization. First, confirm that your cluster hardware meets GH200 specifications and that you have sufficient cooling as well as power supply arrangements. Securely connect the GH200 cards to the correct PCIe slots on your servers.
Afterward, install the necessary software drivers and libraries. Get the latest NVIDIA drivers and CUDA Toolkit from their website; these packages are important for the proper functioning of GH200 while optimizing performance, too. Additionally, ensure that you’re using an OS that supports this platform’s software requirements. If not, any other recent Linux distribution will do since it leverages all features of NVIDIA Grace CPU.
Once you install drivers together with software, configure them to be recognized by your management system so they can be utilized accordingly within the cluster environment. This may require modifications to resource manager settings or even updating scheduler settings so as to allocate GPU resources efficiently. For instance, SLURM or Kubernetes can handle GPU scheduling and allocation.
Finally, fine-tune the system based on workload needs in order to optimize performance levels achieved while using it. Employ various profiling tools like NVIDIA Nsight plus NVML (NVIDIA Management Library), among others provided by NVIDIA, for monitoring performances as well as making necessary adjustments where applicable. Keep firmware versions up-to-date and coupled with regular software package updates for enhanced security stability reasons. In this way one can ensure efficiency together with effectiveness when working within their computational clusters using a comprehensive approach towards setting up a given NVIDIA GH200 device.
Optimizing AI Workloads on DGX GH200
When it comes to the DGX GH200, optimizing AI workloads can be done by following good practices for software setup and hardware configuration, particularly with HBM3E memory. First, verify that your AI framework, like TensorFlow or PyTorch, is completely compatible with CUDA and cuDNN versions on your system. Enabling training in mixed precision can speed up calculations without losing the accuracy of the model.
Furthermore, one should use distributed training techniques which scale training across multiple GPUs effectively using libraries such as Horovod. Optimize memory usage and compute efficiency by using automatic mixed precision (AMP). It is also suggested to make use of NVIDIA’s Deep Learning AMI as well as NGC containers which come pre-configured with latest optimizations for various AI workloads.
Keep an eye on how the system is performing by regular monitoring through Nsight Systems and Nsight Compute – NVIDIA’s profiling tools; this will help you load balance GPU configurations for maximum throughput. By doing these things you will greatly enhance AI workload performance on DGX GH200 in terms of speed and effectivenesss.
Best Practices for Utilizing Grace CPU and Hopper GPU
To maximize the performance of Grace CPUs and Hopper GPUs, it is necessary to adhere to several best practices according to up-to-date recommendations from leading industry sources. First of all, make sure your software stack is optimized for hybrid CPU-GPU workloads. Use NVIDIA’s software development kits (SDKs), such as CUDA and cuDNN, designed specifically for taking advantage of Grace CPUs and Hopper GPUs’ computational capabilities. Also, implement efficient data parallelism techniques together with optimized algorithms for balancing computation load between the two processors.
System architecture should prioritize lowering latency while maximizing bandwidth between a central processing unit (CPU) and graphics processing units (GPUs). This can be achieved by employing high-speed interconnects like NVLink that enable faster data transfer rates, thus reducing occurrence bottlenecks. Moreover, performance parameters may be fine-tuned continuously using profiling tools like NVIDIA Nsight.
Significant gains in performance could be realized when using mixed precision training for artificial intelligence/machine learning tasks alongside frameworks optimized for use on Grace CPUs and Hopper GPUs. This method ensures the best utilization of resources during training by efficiently distributing these tasks using libraries such as Horovod.
Ultimately, keeping pace with recent firmware updates plus drivers provided by NVIDIA is mandatory because they usually come bundled with bug fixes & performance improvements aimed at boosting stability plus efficiency within various computations performed on them. With this set of guidelines, one should be able to tap fully into what Grace CPUs and Hopper GPUs have under their sleeves, thereby experiencing computing nirvana in terms of optimality for both speediness and energy consumption!
Reference Sources
Frequently Asked Questions (FAQs)
Q: What is the NVIDIA GH200 Grace Hopper Superchip?
A: What does this mean for the NVIDIA GH200 Grace Hopper Superchip? Combining GPU and CPU power into one package optimized for fast computing and generative AI workloads, it features a GPU based on Hopper architecture and a powerful CPU joined by high-performance memory which is coherent with both of them through a lot of bandwidth.
Q: How does the GH200 differ from the NVIDIA A100?
A: The NVIDIA A100 was designed mainly for tasks like AI training and inference, but what makes it different from GH200 is that while these things can also be done on it to some extent, it also has other properties. One such property is integration with more advanced HBM3 memory along with GPU as well as CPU cores, which allows us to do more complex computations involving the movement of data between various parts of our system, thereby doubling efficiency in certain cases where workload demands are met accordingly.
Q: What advantages does the DGX H100 system provide?
A: Large language models need a lot of performance, and that’s exactly what they get when run on DGX H100 systems powered by NVIDIA GH200 Grace Hopper Superchips. These machines have high-speed interconnects like NVLink-C2C, among many others, and significant memory bandwidth, so it should come as no surprise that data throughput becomes higher than ever before, thus making everything go faster and smoother, too!
Q: What role does NVIDIA AI Enterprise play in using the GH200?
A: NVIDIA AI Enterprise helps enterprises use accelerated computing tools with maximum GPU memory capabilities. It achieves this by taking advantage of GHCPU and GPUMEMORYSPEED, two features offered by the software suite to ensure efficient resource utilization during accelerated computing applications such as deep learning models utilizing massive amounts of data stored within large datasets.
Q: How does HBM3 memory enhance the GH200 Grace Hopper Superchip?
A: For the GH200 Grace Hopper Superchip, HBM3 memory significantly boosts GPU data bandwidth. This allows faster transfer rates and, therefore, improved performance when it comes to tasks that require a lot of memory, such as AI and generative workloads, which usually deal with large datasets.
Q: What is the significance of NVIDIA NVLink-C2C in GH200?
A: The importance of NVIDIA NVLink-C2C in GH200 is that it allows computers to communicate with one another at high speeds. It interconnects CPU and GPU within GH200, providing high bandwidth for efficient data transfer with minimum latency. This connection links the CPU memory space with the GPU memory space thereby establishing coherence between them necessary for seamless operation during complex computational tasks.
Q: How will the GH200 impact the era of accelerated computing?
A: The GH200 chip by Grace Hopper Supercomputing Center (GHSC) is a game-changer for accelerated computing because it brings together CPUs and GPUs under one roof while greatly enhancing their memory and interconnection capacities. This integration is designed to meet rising demands brought about by generative AI workloads alongside large-scale data processing.
Q: What does NVIDIA Base Command do within the ecosystem of GH200?
A: Within this system, NVIDIA Base Command serves as an inclusive platform for managing and organizing artificial intelligence workflows on top of GH200. It ensures easy implementation, tracking, and scaling up or down AI models, thus enabling businesses to make full use of what GH200 has to offer.
Q: How does the new GH200 Grace Hopper Superchip support large language models?
A: LPDDR5X memory, among other things, forms part of its advanced architecture, which enables it to process and train big language models more effectively than any other device available today can ever hope to achieve for such purposes. Besides having ample amounts of memory bandwidth at its disposal, it also performs parallel computations very well, meaning that there isn’t another piece out there better suited to these types of applications than this chip.
Related Products:
-
NVIDIA MMA4Z00-NS400 Compatible 400G OSFP SR4 Flat Top PAM4 850nm 30m on OM3/50m on OM4 MTP/MPO-12 Multimode FEC Optical Transceiver Module $650.00
-
NVIDIA MMA4Z00-NS-FLT Compatible 800Gb/s Twin-port OSFP 2x400G SR8 PAM4 850nm 100m DOM Dual MPO-12 MMF Optical Transceiver Module $850.00
-
NVIDIA MMA4Z00-NS Compatible 800Gb/s Twin-port OSFP 2x400G SR8 PAM4 850nm 100m DOM Dual MPO-12 MMF Optical Transceiver Module $750.00
-
NVIDIA MMS4X00-NM Compatible 800Gb/s Twin-port OSFP 2x400G PAM4 1310nm 500m DOM Dual MTP/MPO-12 SMF Optical Transceiver Module $1100.00
-
NVIDIA MMS4X00-NM-FLT Compatible 800G Twin-port OSFP 2x400G Flat Top PAM4 1310nm 500m DOM Dual MTP/MPO-12 SMF Optical Transceiver Module $1200.00
-
NVIDIA MMS4X00-NS400 Compatible 400G OSFP DR4 Flat Top PAM4 1310nm MTP/MPO-12 500m SMF FEC Optical Transceiver Module $800.00
-
Mellanox MMA1T00-HS Compatible 200G Infiniband HDR QSFP56 SR4 850nm 100m MPO-12 APC OM3/OM4 FEC PAM4 Optical Transceiver Module $200.00
-
NVIDIA MFP7E10-N010 Compatible 10m (33ft) 8 Fibers Low Insertion Loss Female to Female MPO Trunk Cable Polarity B APC to APC LSZH Multimode OM3 50/125 $47.00
-
NVIDIA MCP7Y00-N003-FLT Compatible 3m (10ft) 800G Twin-port OSFP to 2x400G Flat Top OSFP InfiniBand NDR Breakout DAC $275.00
-
NVIDIA MCP7Y70-H002 Compatible 2m (7ft) 400G Twin-port 2x200G OSFP to 4x100G QSFP56 Passive Breakout Direct Attach Copper Cable $155.00
-
NVIDIA MCA4J80-N003-FTF Compatible 3m (10ft) 800G Twin-port 2x400G OSFP to 2x400G OSFP InfiniBand NDR Active Copper Cable, Flat top on one end and Finned top on other $600.00
-
NVIDIA MCP7Y10-N002 Compatible 2m (7ft) 800G InfiniBand NDR Twin-port OSFP to 2x400G QSFP112 Breakout DAC $200.00