The rapid advancement of artificial intelligence (AI) technology is revolutionizing the cloud computing and IT industries. Since the launch of Chat GPT in November 2022, the AI field has experienced an investment boom, attracting significant attention. Major cloud service providers have introduced new products and services to meet the growing demand for AI, while many large enterprises are actively exploring AI use cases such as generative AI (GenAI) to enhance operational efficiency and return on investment.
However, the rapid development of AI presents higher demands on the infrastructure of cloud service providers and enterprise data centers. Data, as the critical “fuel” for AI development, must be collected, protected, and transmitted efficiently. Organizations exploring new AI applications must address these challenges. To support the massive data and computational resources required by AI, we need to build more efficient and reliable network infrastructures.
In this context, Ethernet technology, with its mature and widespread ecosystem, is becoming a crucial support for AI network infrastructure. Ethernet shows strong potential to meet the high demands of AI and provide a unified platform, which significantly impacts the economic viability of AI. It can achieve consistent operational models across various networks and clouds, avoiding the high costs associated with maintaining multiple infrastructures.
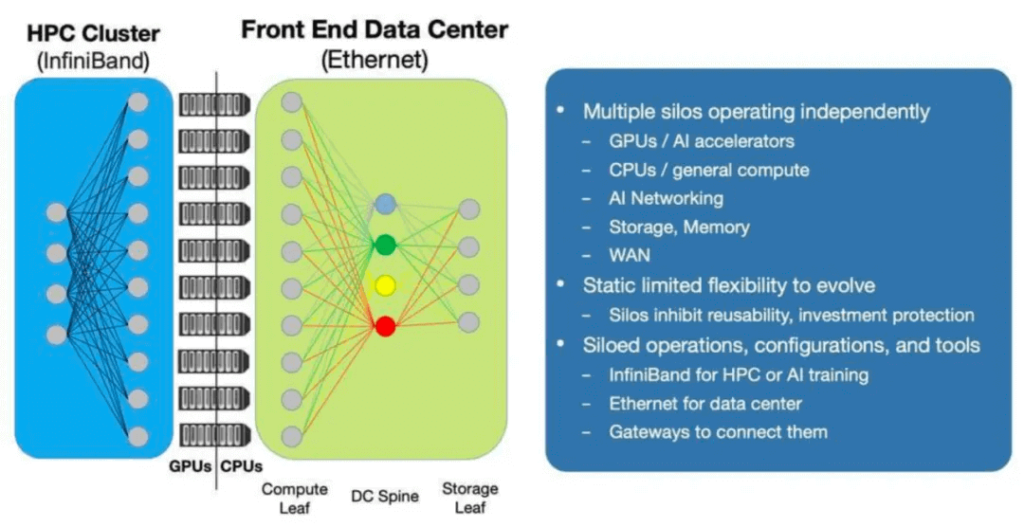
Key Requirements for AI Network Development
- Speed: The rapid growth of AI services drives the need for higher speeds in data centers and edge networks, pushing networks towards new generations like 400 Gbit/s and even 800 Gbit/s.
- Privacy and Security: Networks must efficiently handle data while ensuring high-end encryption and security in multi-tenant environments to protect data privacy.
- Edge Inference: As enterprises deploy large language models (LLMs) or small language models (SLMs) and hybrid private AI clouds, the front-end deployment of inference capabilities will become a focal point.
- Short Job Completion Time (JCT) and Low Latency: Optimizing networks to provide lossless transmission, ensuring efficient bandwidth utilization through congestion management and load balancing, is key to achieving rapid JCT.
- Flexible Clusters: In AI data centers, processor clusters can be configured into various topologies. Optimizing performance requires avoiding oversubscription between layers or regions to reduce JCT.
- Multi-Tenant Support: For security reasons, AI networks need to separate data flows.
- Standardized Architecture: AI networks typically consist of back-end infrastructure (training) and front-end (inference). The generality of Ethernet allows for technical reuse between back-end and front-end clusters.
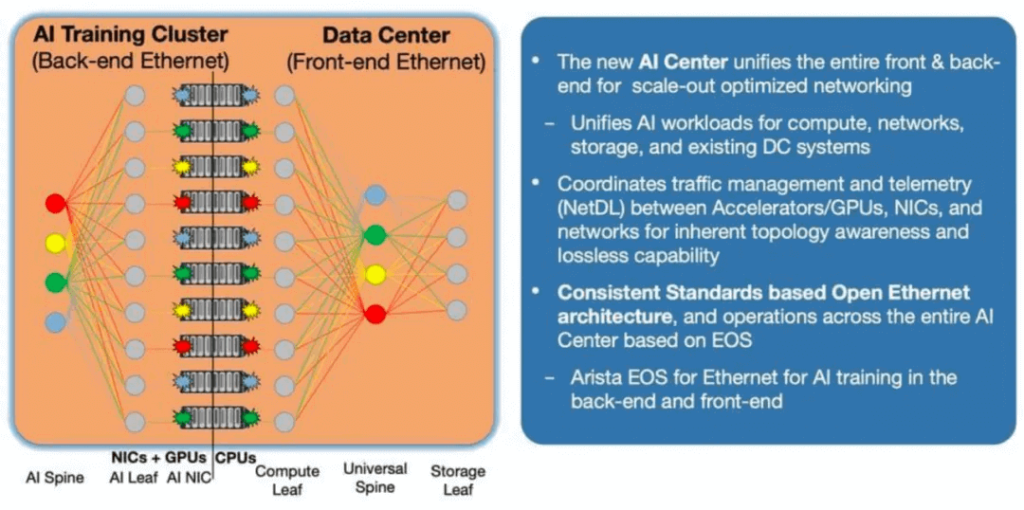
Continuous Innovation in Ethernet Technology
Ethernet technology is continuously innovating and developing to meet the higher demands on network scale by AI. Some key technological advancements include:
- Packet Spraying: This technology allows each network flow to access all paths to the destination simultaneously. The flexible ordering of packets fully utilizes all Ethernet links with optimal load balancing, enforcing ordering only when bandwidth-intensive operations require it in AI workloads.
- Congestion Management: Ethernet-based congestion control algorithms are crucial for AI workloads. They prevent hotspots and evenly distribute load across multiple paths, ensuring reliable transmission of AI traffic.
Unified and Optimized Enterprise Infrastructure
Enterprises need to deploy unified AI network infrastructure and operational models to reduce the cost of AI services and applications. Adopting standard-based Ethernet as the supporting technology is a core element. It ensures compatibility between front-end and back-end systems, avoiding the standardization process obstacles and economic impacts brought by different architectures. For example, Arista advocates building an “AI Center,” where GPUs are efficiently trained through lossless networks. The trained AI models are connected to AI inference clusters, allowing end users to query these models conveniently.
Market Advantages of Ethernet
Ethernet exhibits strong competitiveness in AI deployment due to its openness, flexibility, and adaptability. Its performance surpasses InfiniBand, and with the enhancements from the Ultra Ethernet Consortium (UEC), its advantages will further expand. Moreover, Ethernet is more cost-effective, has a broader and more open ecosystem, providing generality, unified operations, and skill sets for both back-end and front-end clusters, as well as platform reuse opportunities between clusters. As AI use cases and services continue to expand, the opportunities for Ethernet infrastructure will significantly increase, whether at the core of hyperscale LLMs or at the enterprise edge. AI-ready Ethernet can meet the demand and provide AI inference based on industry-specific private data.
In summary, Ethernet technology plays a critical role in AI network infrastructure. It can meet the multifaceted needs of AI in terms of speed, security, edge inference, and more. Through continuous technological innovation and extensive ecosystem support, Ethernet provides more efficient and cost-effective solutions for enterprises, promoting the widespread application and development of AI.
Related Products:
-
Arista Networks QDD-400G-SR8 Compatible 400G QSFP-DD SR8 PAM4 850nm 100m MTP/MPO OM3 FEC Optical Transceiver Module $180.00
-
Arista Networks QDD-400G-DR4 Compatible 400G QSFP-DD DR4 PAM4 1310nm 500m MTP/MPO SMF FEC Optical Transceiver Module $450.00
-
Arista QDD-400G-VSR4 Compatible QSFP-DD 400G SR4 PAM4 850nm 100m MTP/MPO-12 OM4 FEC Optical Transceiver Module $600.00
-
Arista Networks QDD-400G-FR4 Compatible 400G QSFP-DD FR4 PAM4 CWDM4 2km LC SMF FEC Optical Transceiver Module $600.00
-
Arista Networks QDD-400G-XDR4 Compatible 400G QSFP-DD XDR4 PAM4 1310nm 2km MTP/MPO-12 SMF FEC Optical Transceiver Module $650.00
-
Arista Networks QDD-400G-LR4 Compatible 400G QSFP-DD LR4 PAM4 CWDM4 10km LC SMF FEC Optical Transceiver Module $650.00
-
Arista QDD-400G-SRBD Compatible 400G QSFP-DD SR4 BiDi PAM4 850nm/910nm 100m/150m OM4/OM5 MMF MPO-12 FEC Optical Transceiver Module $1000.00
-
Arista Networks QDD-400G-PLR4 Compatible 400G QSFP-DD PLR4 PAM4 1310nm 10km MTP/MPO-12 SMF FEC Optical Transceiver Module $1000.00
-
Arista Q112-400G-DR4 Compatible 400G NDR QSFP112 DR4 PAM4 1310nm 500m MPO-12 with FEC Optical Transceiver Module $800.00
-
Arista Q112-400G-SR4 Compatible 400G QSFP112 SR4 PAM4 850nm 100m MTP/MPO-12 OM3 FEC Optical Transceiver Module $650.00
-
Arista OSFP-400G-LR4 Compatible 400G LR4 OSFP PAM4 CWDM4 LC 10km SMF Optical Transceiver Module $1199.00
-
Arista OSFP-400G-XDR4 Compatible 400G OSFP DR4+ 1310nm MPO-12 2km SMF Optical Transceiver Module $879.00
-
Arista Networks OSFP-400G-2FR4 Compatible 2x 200G OSFP FR4 PAM4 2x CWDM4 CS 2km SMF FEC Optical Transceiver Module $3000.00
-
Arista Networks OSFP-400G-FR4 Compatible 400G OSFP FR4 PAM4 CWDM4 2km LC SMF FEC Optical Transceiver Module $900.00
-
Arista Networks OSFP-400G-DR4 Compatible 400G OSFP DR4 PAM4 1310nm MTP/MPO-12 500m SMF FEC Optical Transceiver Module $900.00
-
Arista Networks OSFP-400G-SR8 Compatible 400G OSFP SR8 PAM4 850nm MTP/MPO-16 100m OM3 MMF FEC Optical Transceiver Module $480.00
-
Arista OSFP-800G-2SR4 Compatible OSFP 2x400G SR4 PAM4 850nm 100m DOM Dual MPO-12 MMF Optical Transceiver Module $750.00
-
Arista OSFP-800G-2PLR4 Compatible OSFP 8x100G LR PAM4 1310nm Dual MPO-12 10km SMF Optical Transceiver Module $2200.00
-
Arista OSFP-800G-2XDR4 Compatible OSFP 8x100G FR PAM4 1310nm Dual MPO-12 2km SMF Optical Transceiver Module $1300.00
-
Arista OSFP-800G-2LR4 Compatible OSFP 2x400G LR4 PAM4 CWDM4 Dual duplex LC 10km SMF Optical Transceiver Module $3700.00