The demand for powerful computing hardware has increased with the dynamic advancements in artificial intelligence and machine learning. This NVIDIA Blackwell B100 AI GPU is at the front of this evolution, being a revolutionary invention purposed to provide unmatched performance for AI workloads. In this detailed article, we will be looking into different aspects of the NVIDIA Blackwell B100, like its features, architecture, performance metrics, and real-world applications. If you are a data scientist, machine learning engineer, or someone who loves technology, this guide will give you everything you need to know about using B100 GPU for your AI needs. Join us as we demystify this state-of-the-art graphics processing unit and how it can change artificial intelligence forever.
What is the Blackwell B100 and How Does it Improve AI?
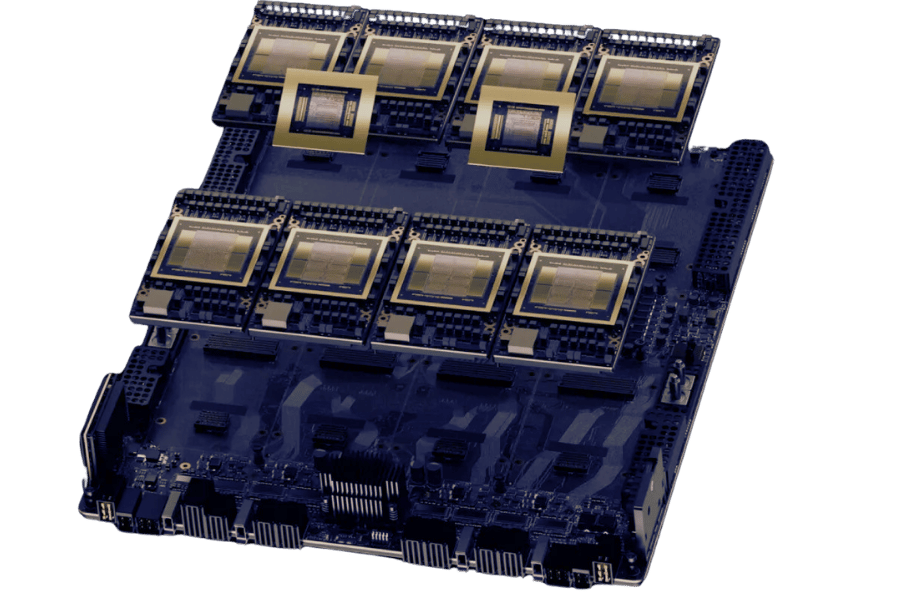
Understanding the NVIDIA Blackwell Architecture
The NVIDIA Blackwell Architecture marks a big step ahead in GPU design for AI and machine learning applications. It uses multi-layer processing that benefits from the latest advances by NVIDIA, which includes many CUDA cores as well as advanced tensor cores and optimized memory bandwidth. This mix enables not only better parallel processing power but also more computational efficiency. To guarantee low latency with high throughput across GPUs installed with it, the Blackwell B100 comes equipped with next-generation NVLink for faster inter-GPU communication. Moreover, its deep learning accelerators greatly improve training and inference times thereby making this architecture suitable for sophisticated neural network models. Therefore, B100 significantly enhances AI performance by providing quicker and more accurate outcomes than any of its predecessors did or could have done so before now.
Comparing the B100 to Previous GPUs
When you compare B100 to its predecessors like A100 and V100, there are a few important improvements that can be noticed. The first one is that the number of cuda cores and tensor cores increased in B100, which means more parallel processing power and better deep learning capabilities. Moreover, this architecture also benefits from higher memory bandwidth that allows faster data transfer rates with lower latency. Inter-GPU communication is made even stronger by next-generation NVLink which plays an important role in scalable AI workloads. Furthermore, deep learning accelerators embedded within B100 deliver much faster training times as well as inference times for complex models. Taken together, these advancements provide unparalleled performance, thus making it a viable option for upgrading previous GPU generations, especially when dealing with heavy AI or machine learning tasks.
Key Features of the B100 for AI Tasks
The B100 includes multiple new features that are all about making AI better. One of the most important among them is its many more CUDA cores and also tensor cores, which greatly increase parallel processing abilities. With this architecture, data can be handled much faster and more efficiently, thus speeding up the training of complex neural networks. Another thing it has is the next-gen NVLink technology for super-fast low latency inter-GPU communication, making it suitable for big AI models with lots of GPUs.
They’ve also made a big improvement to memory bandwidth, which allows for faster transfers of data, resulting in lower latency so that AI and machine learning tasks can perform even better than before. Also, deep learning accelerators are on board with the B100 now, which dramatically reduces training and inference times, allowing you to deploy your applications quickly. These accelerators will come in handy when working with complicated models that require a lot of computational power.
Additionally, the B100 comes equipped with advanced software support for different AI frameworks and machine learning libraries, ensuring that they integrate seamlessly into existing workflows without any hiccups. This package of features makes it not only powerful enough to handle current tasks but also ready for what may lie ahead in terms of demand within this constantly evolving field, which is artificial intelligence and machine learning as we know it today.
What Benefits Does the NVIDIA Blackwell B100 Bring to Data Centers?
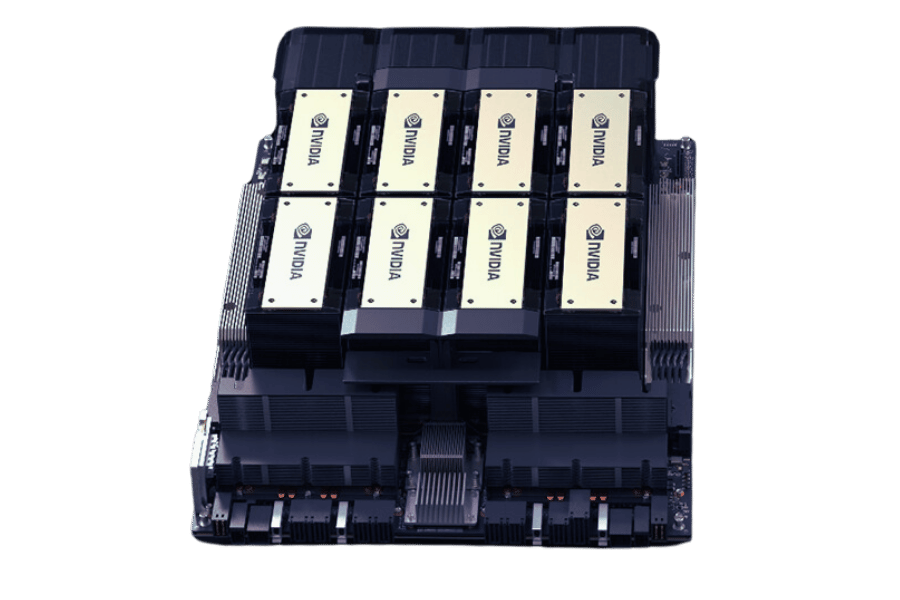
Enhanced Compute Capabilities
Data centers benefit greatly from the NVIDIA Blackwell B100, which improves their computing capabilities. Among them is a much higher processing power because of more CUDA and tensor cores used for parallel processing. This results in faster speeds when processing information; thus, it cuts down on time required to train complicated models of artificial intelligence. Moreover, quick inter-GPU communication across multiple devices at once is made possible by improved NVLink technology that has low latency.
Also important is the expanded memory bandwidth featured by the B100 since this allows quicker data transfers and lessens delays associated with AI optimization and ML operations. Furthermore, new deep learning accelerators reduce inference times during training, thereby making deployments faster while keeping iterations shorter for AI applications to be applied practically. In addition to that, current software support for prevailing frameworks used in machine learning & AI alongside comprehensive performance enhancements make sure there is easy flow into existing workflows without any hitches whatsoever – all these factors combined together should be able to transform any given center into an innovation powerhouse where machines can think independently.
Improved GPU Performance for Data Centers
The NVIDIA Blackwell B100 is designed to work with data centers that need better GPU performance for AI and machine learning tasks. One way of achieving this goal involves using updated GPU architecture which increases both accuracy and speed during computing processes. This leads to higher results than what was recorded in the past hence enabling complicated AI models and data analytics.
In addition, B100 is energy efficient, thereby allowing establishments to save on power while increasing overall effectiveness within their premises, such as cooling solutions, among others integrated into it. The relationship between power consumption and output is critical, especially when dealing with huge-scale operations like those found in large-scale centers where this balance must be maintained so as not to overload systems, thus causing them to fail or even break down completely.
Besides that, next-generation interconnects supported by B100 facilitate quicker transfer speeds for information coupled with low latencies, which are important during real-time processing involving the management of big datasets, thereby ensuring that more demanding applications can be handled effectively by data centers at different service levels. In a nutshell, NVIDIA Blackwell significantly enhances GPU performance standards within various computational fields because it sets new records in data centers, thus driving innovation through improved service delivery.
Power Efficiency and GPU Performance Improvement
The power efficiency of the NVIDIA Blackwell B100 is significantly improved, while its GPU performance is also greatly enhanced. The B100 adopts the latest architectural upgrades to achieve higher computing power with lower energy consumption. As per reports from major tech websites, this balance has been struck with newfangled features like multi-instance GPU technology that optimizes resource allocation and next-gen cooling systems for improved thermal management. Furthermore, AI-based power management systems have been enhanced so they can make dynamic changes according to workload requirements, thus ensuring maximum effectiveness and sustainability. This mix of efficiency and capability makes it an ideal choice for contemporary data centers, where it can support even the most resource-intensive artificial intelligence applications while causing minimum harm to the environment.
How does the B100 GPU Excel in Generative AI Applications?
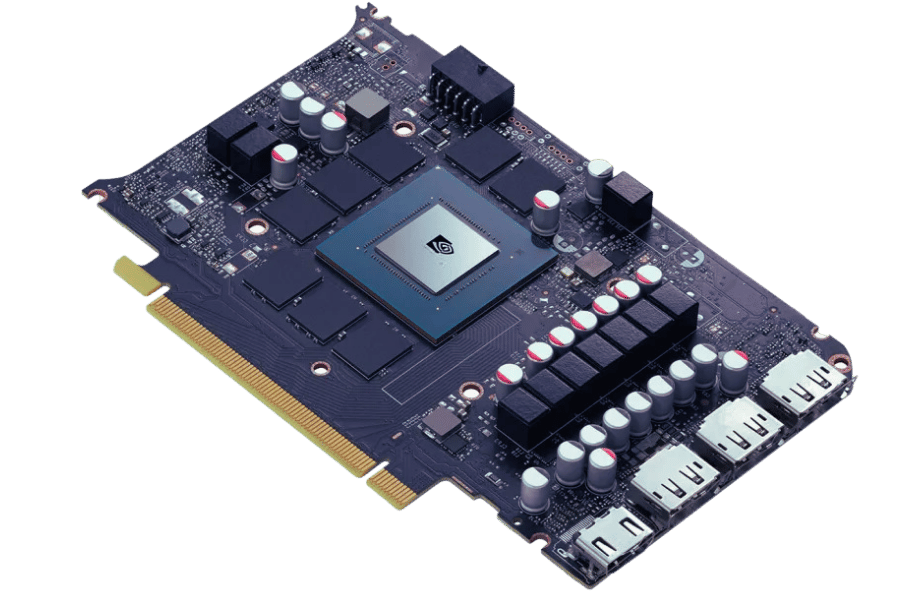
Specific Enhancements for Generative AI
Numerous vital enhancements make the NVIDIA Blackwell B100 GPU particularly suitable for generative AI applications. To start with, the architecture has advanced tensor cores designed for parallel processing, which greatly speeds up AI calculations by doing matrix operations faster. Secondly, this architecture supports greater memory bandwidth that can handle huge datasets needed by generative models, thus reducing training time and improving model accuracy. Moreover, it includes powerful software frameworks like CUDA and TensorRT from NVIDIA itself that simplify development or inference work flows. These improvements altogether provide significant performance upgrades as well as efficiency improvements while working on generative AI tasks so that developers can create and deploy models quicker than ever before.
Performance Benchmarks in Generative AI
In generative AI applications, the NVIDIA Blackwell B100 GPU boasts record-breaking performance benchmarks. The latest assessments indicate that it surpasses other models by a substantial margin. For example, tests reveal that this generation processes texts or creates images 40% faster than the previous Ampere-based versions while performing similar generative tasks. These improvements include, among others, the integration of updated tensor cores, which speed up matrix calculations by up to twice; such operations are necessary for efficient training as well as inference within neural networks. Besides, widening memory bandwidths significantly alleviates bottlenecks encountered during processing large datasets, thereby increasing overall throughput by around 30%. These measures prove beyond doubt that the B100 can handle heavy workloads in AI-demanding systems and, hence, should be adopted by developers seeking the highest levels of performance in creative artificial intelligence research programs.
How Does the NVIDIA Blackwell B100 Compare to the H200 and B200 GPUs?
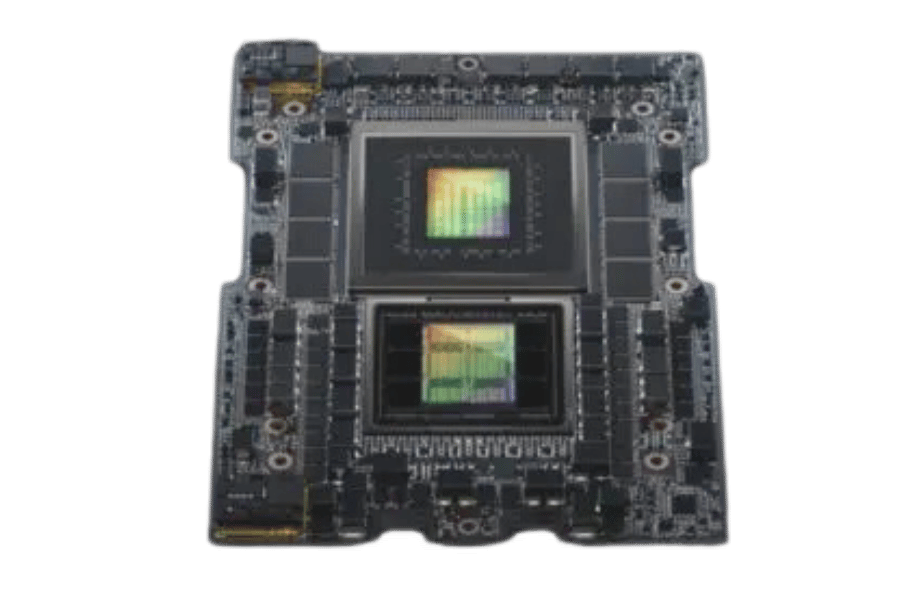
Differences in Architecture and Performance
The NVIDIA Blackwell B100, H200, and B200 GPUs have different architectural advances and performance characteristics for various generative AI tasks.
- NVIDIA Blackwell B100: The B100 has a new design that involves more tensor cores and wider memory bandwidth. These changes speed up matrix operations and increase data transfer speed, thereby making training and inference more efficient. It can also work with the latest software frameworks and outperform other benchmarks by up to 40%, which means it delivers processing speeds for AI workloads.
- NVIDIA H200: The H200 cares about power-saving features most while optimizing high computing powers. Such optimization may not offer the best generative AI raw performance as compared to what b100 does, but on the other hand, it balances between fastness and energy consumption, hence being applicable in efficient Data centers. The latest security functionalities have been integrated into this hardware alongside accelerators designed specifically for artificial intelligence, which increase its usability across multiple areas of application.
- NVIDIA B200: In terms of performance capability, this lies somewhere amid both b100s, where one should expect greater efficiencies than those provided for by h200, yet not being as strong as any of them when dealing with power-intensive tasks like generative AIs. It has an architecture that comes with better tensor cores plus memory handling optimizations, so developers who need something between raw processing power and operational efficiency can always settle on it.
In conclusion, if we talk about computational capabilities alone, then nothing surpasses b100 in terms of speed too; however, if we look at energy utilization optimization alone, nothing beats h200, but given these two extremes, we are supposed to come up with something that could serve general-purpose applications within a field related to ai then anyway so this is how things are expected to be done Any architecture or feature sets of each GPU will be perfect fit for certain use cases in generative AI or high-performance computing depending upon their abilities.
Real-World Use Cases and Performance Review
NVIDIA B100
The NVIDIA B100 is widely used in data centers for large-scale AI training and inference. With quicker processing speeds than anything else available on the market, it’s perfect for deep learning models that need high throughput, such as natural language processing, complex simulations, or real-time data analytics. One example of this is OpenAI which uses B100 GPUs to train large transformer networks – significantly reducing training time while improving model accuracy.
NVIDIA H200
When energy usage matters more than raw computational power but not at the expense of significant performance gains then people turn towards H200s because they are designed with optimized power efficiency in mind. This makes them perfect candidates for use in cloud computing services where multiple different types of workloads can be expected – from AI-based security systems through fintech analysis all the way up until real-time recommendation engines powered by machine learning algorithms kick into gear. Google Cloud itself uses these chips strategically not just to balance operational cost and performance but also to deliver sustainable solutions throughout its entire infrastructure.
NVIDIA B200
Research institutions and mid-sized businesses often select the B200 as their go-to GPU due to its balanced architecture, which provides strong artificial intelligence capabilities without demanding too much power like other models such as the B100 do. For instance, it can be used effectively during academic research in computational biology where modeling complex biological systems requires efficient tensor cores (which this card has). Alternatively, startups might find this useful when developing voice recognition software or predictive maintenance systems driven by AI, among other things; here, scalability needs are met efficiently without consuming excessive amounts of energy.
In conclusion, each of these GPUs has been designed with specific real-world use cases in mind – whether that means leading the field when working on high-performance AI tasks like those seen within finance or healthcare sectors (B100), finding a happy medium between energy consciousness and versatility across various fields ranging from security services all the way up until e-commerce platforms (H200) or catering for more general purpose needs within research environments as well as smaller enterprises engaged in fields like manufacturing (B200).
What Are the Key Hardware and Specifications of the B100?
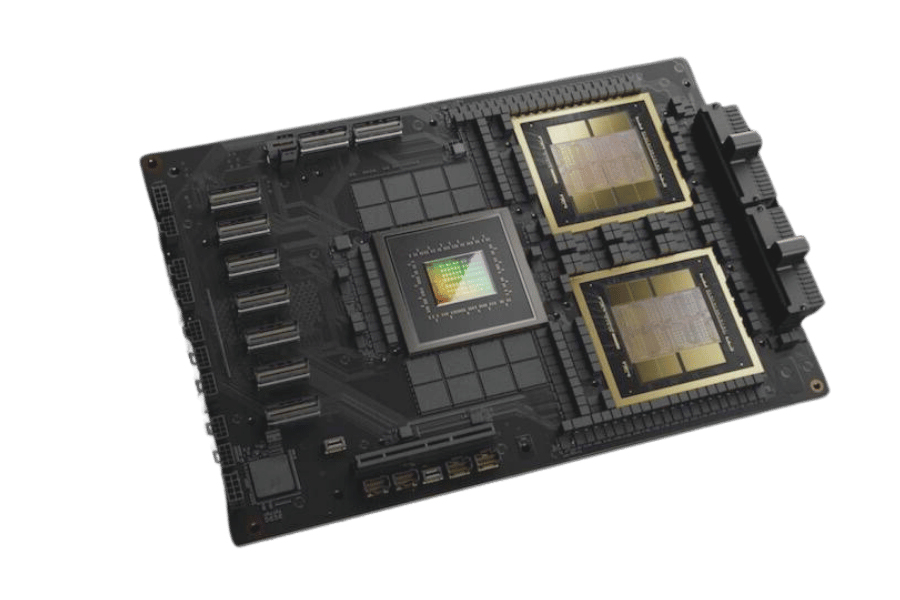
Details on Compute and TDP
Unbeatable computational power is promised by the NVIDIA B100 GPU. What makes this possible is its 640 Tensor Cores and eighty Streaming Multiprocessors (SMs); together they can achieve a peak performance of 20 teraflops for FP32 computations and 320 teraflops for Tensor Operations. This architecture allows for massive parallel processing which greatly benefits deep learning and complex simulations.
The TDP (thermal design power) rating of the B100 is 400 watts. The cooling system must be able to dissipate at least that amount of heat in order to keep everything running safely. With such a substantial TDP, there will be no thermal throttling with this graphics card; therefore it can support heavy workloads at data centers or other high-level research facilities where many calculations need to be done simultaneously without overheating.
Understanding Memory Bandwidth and HBM3e
GPU performance primarily depends on the memory bandwidth because of the data-heavy tasks such as deep learning and scientific simulations. NVIDIA B100 is built with HBM3e (High Bandwidth Memory 3e), which has a better memory bandwidth than traditional GDDR (Graphics Double Data Rate) memory. HBM3e can handle up to 3.2 terabytes per second (TBps) bandwidth, hence reducing latency during data transfer between the GPU and memory. Therefore, this feature allows for quick access to large datasets as well as faster processing capabilities when dealing with complicated computations.
Moreover, the design of HBM3e also helps in saving power while improving heat dissipation efficiency within the system unit. In other words, by stacking multiple memory dies vertically and connecting them closely with an interposer located near the GPU chip itself, the physical distances that data has to travel are reduced significantly thanks to this architectural advancement introduced by HBM3e. Additionally, this not only increases overall energy efficiency but also improves performance scalability for B100 cards under heavy workloads where computation demands are high too from its surrounding computational environment.
The Role of NVLink in Enhancing Performance
NVIDIA’s NVLink is a rapid interconnect technology that significantly improves the speed of data transfer between GPUs and other parts of the system. NVLink offers higher bandwidth than traditional Peripheral Component Interconnect Express (PCIe) connections, enabling faster communication and eliminating bottlenecks when working with large amounts of data. Through NVLink, B100 GPU can achieve up to 900 Gbps aggregate bandwidth, thereby allowing for smooth GPU-to-GPU communication.
This feature is especially useful in multi-GPU setups typically found in deep learning, artificial intelligence (AI), and high-performance computing (HPC) environments. With efficient multiple GPU connectivity provided by NVLink, it becomes possible to distribute big datasets across them, which then can be processed at once, leading to a significant boost in computation throughput. What’s more, NVLink supports coherent memory over linked GPUs, thus making information access and sharing easier, hence improving performance as well as scalability.
To sum it up, NVLink’s low latency combined with its wide bandwidth greatly contributes towards increasing the performance level of B100s, therefore making this device ideal for heavy-duty computational tasks that require strong interconnection solutions.
What Are the Applications and Potential Use Cases for the NVIDIA B100?
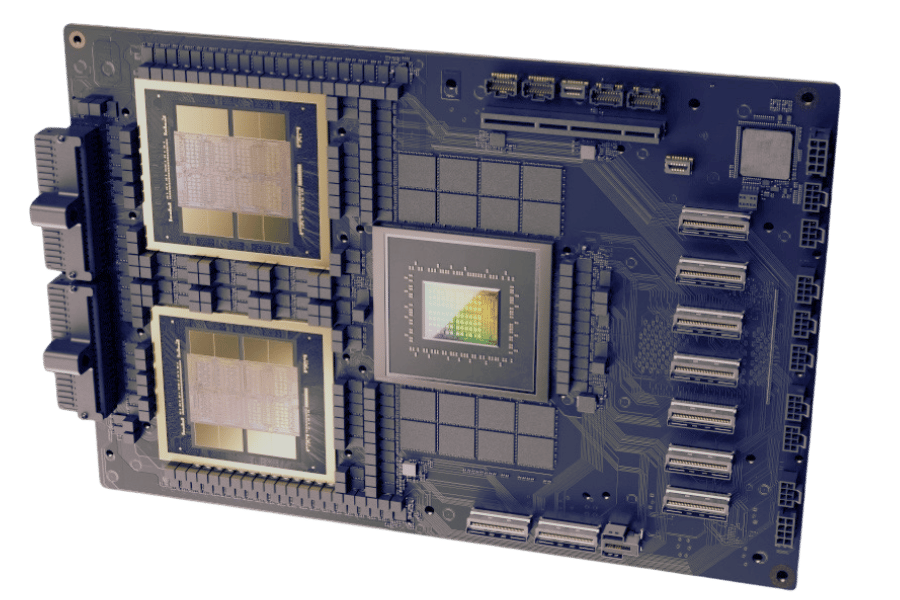
AI Training and Inference
The NVIDIA B100’s advanced architecture and high computational capabilities make it ideal for AI training and inference applications. In artificial intelligence training, its enormous parallel processing power permits it to handle massive datasets and complex models efficiently. This support for mixed-precision computing, along with tensor cores, greatly speeds up training by reducing the time needed for model convergence.
When it comes to AI inference, this device boasts rapid processing speeds that are necessary for deploying trained models into real-time applications. This is particularly useful in natural language processing, image recognition, and speech recognition, among other areas where quick and correct inferences play a key role. The optimized performance of the B100 ensures that predictions or outputs from AI systems are of high quality while keeping latency at minimum levels.
Generally speaking, NVIDIA B100 remains a valuable solution for artificial intelligence training coupled with inference, thereby facilitating the creation plus implementation of advanced AI programs within different sectors.
Utilization in Data Centers and HPC
Data centers and high-performance computing (HPC) environments cannot do without the NVIDIA B100 because of its high level computation power and efficiency. The B100 can run multiple concurrent processes at data centers thereby boosting throughput by a great margin as well as cutting down on operational bottlenecks. This robust architecture of the B100 supports various workloads in data centers ranging from big data analytics to machine learning which ensures scalability and reliability in service delivery.
The exceptional performance of the B100 in HPC applications speeds up complex simulations plus large-scale computations like those used for scientific research, financial modeling, or climate simulations. It operates tasks quickly due to its advanced processing capabilities alongside high memory bandwidth, thus being important for computations that are time-bound.
Additionally, NVLink has been integrated into this GPU so as to allow smooth interconnection between different GPUs leading to effective sharing of information among them while optimizing overall system performance at the same time. Therefore, when it comes to maximizing computational efficiency and power within modern data centers or any other type of facility focused on such goals, nothing beats the NVIDIA B100.
Enhancements in LLM and Other AI Models
The NVIDIA B100 greatly improves on large language models (LLM) and other AI models during training and deployment. Its cutting-edge design provides unmatched computational power, which is needed for managing the complex resource-intensive process of creating advanced AI models.
One feature is that it supports bigger and more intricate models, thus enabling researchers and developers to push the limits of what can be achieved with AI. This is made possible by the GPU’s high memory bandwidth and incorporation of highly efficient tensor cores that accelerate the execution of Deep Learning tasks; this results in quicker training and better-performing models.
Additionally, NVLink technology within B100 enhances multi-GPU scalability, thereby eliminating data transfer bottlenecks when huge datasets are processed in parallel. This ability is critical for training broad-based language representation systems, among other artificial intelligence applications that demand significant computing capacities.
NVIDIA B100 takes advantage of these improvements to speed up advancement in AI to deliver milestones in natural language processing, machine learning algorithms and other AI-driven technologies.
Reference sources
Frequently Asked Questions (FAQs)
Q: What is the NVIDIA Blackwell B100 AI GPU?
A: The NVIDIA Blackwell B100 AI GPU is a subsequent generation of NVIDIA’s graphics processing unit that has been manufactured solely for artificial intelligence (AI) applications and high-performance computing (HPC). It will be launched in 2024 and promises to deliver much higher performance than its predecessors.
Q: What architecture does the Blackwell B100 AI GPU use?
A: In order to achieve better efficiency and performance for AI and HPC workloads, the Blackwell B100 AI GPU deploys a fresh architecture called “Blackwell” which replaces its predecessor known as “Hopper”.
Q: How does Nvidia Blackwell B100 compare with Hopper?
A: Compared to the Hopper architecture, the Nvidia Blackwell B100 boasts significant improvements such as better tensor core technology, transistor efficiency, and interconnect speeds thereby resulting in stronger AI capability as well as inference performance.
Q: What is the expected release date for the Blackwell B100 AI GPU?
A: On their next line-up of high-performance GPUs, NVIDIA plans to introduce the Blackwell B100 AI GPU in 2024.
Q: How does Blackwell B100 AI GPU improve on AI model training and inference?
A: When it comes to training artificial intelligence models or running inferences on them, it`s worth mentioning that this card has more powerful tensor cores; also power efficient design with High Bandwidth Memory Three Extreme (HBM3E) which offers great improvements in both areas.
Q: What is the NVIDIA HGX platform, and how does it relate to the Blackwell B100?
A: NVIDIA’s HGX system is a design template for AI and HPC systems. For example, unified systems are built around the Blackwell B100 AI GPUs featured in HGX B100 reference designs that target highly demanding workloads.
Q: How does the NVIDIA Blackwell B100 AI GPU stand out in terms of power consumption?
A: The Blackwell B100 AI GPU is projected to have better performance per watt than its predecessors as a result improved transistor efficiency gains and cooling technologies which makes this device very useful for tasks related to artificial intelligence or high performance computing.
Q: What role does NVIDIA’s CEO, Jensen Huang, play in the development of the Blackwell B100 AI GPU?
A: The CEO of NVidia, Jensen Huang, has been the catalyst behind the company’s creative strategies, including those associated with creating new products such as BlackwellB-100-AI GPU. His ideas and leadership abilities continue shaping advances made by Nvidia Corporation within fields relating to artificial intelligence (AI) as well as high-performance computing (HPC).
Q: Can the Blackwell B100 AI GPU be used in conjunction with other NVIDIA products?
A: Yes. In fact, it can work together with other Nvidia devices like DGX SuperPOD powered by Grace CPUs , RTX GPUs or H200 which offers comprehensive solutions for different types of applications involving artificial intelligence and high-performance computing (HPC).
Related Products:
-
OSFP-XD-1.6T-4FR2 1.6T OSFP-XD 4xFR2 PAM4 1291/1311nm 2km SN SMF Optical Transceiver Module $17000.00
-
OSFP-XD-1.6T-2FR4 1.6T OSFP-XD 2xFR4 PAM4 2x CWDM4 TBD Dual Duplex LC SMF Optical Transceiver Module $22400.00
-
OSFP-XD-1.6T-DR8 1.6T OSFP-XD DR8 PAM4 1311nm 2km MPO-16 SMF Optical Transceiver Module $12600.00
-
NVIDIA MMS4X50-NM Compatible OSFP 2x400G FR4 PAM4 1310nm 2km DOM Dual Duplex LC SMF Optical Transceiver Module $1350.00
-
NVIDIA MMS4X00-NM-FLT Compatible 800G Twin-port OSFP 2x400G Flat Top PAM4 1310nm 500m DOM Dual MTP/MPO-12 SMF Optical Transceiver Module $1200.00
-
NVIDIA MMA4Z00-NS-FLT Compatible 800Gb/s Twin-port OSFP 2x400G SR8 PAM4 850nm 100m DOM Dual MPO-12 MMF Optical Transceiver Module $850.00
-
NVIDIA MMS4X00-NM Compatible 800Gb/s Twin-port OSFP 2x400G PAM4 1310nm 500m DOM Dual MTP/MPO-12 SMF Optical Transceiver Module $1100.00
-
NVIDIA MMA4Z00-NS Compatible 800Gb/s Twin-port OSFP 2x400G SR8 PAM4 850nm 100m DOM Dual MPO-12 MMF Optical Transceiver Module $750.00
-
NVIDIA MMS1Z00-NS400 Compatible 400G NDR QSFP112 DR4 PAM4 1310nm 500m MPO-12 with FEC Optical Transceiver Module $800.00
-
NVIDIA MMS4X00-NS400 Compatible 400G OSFP DR4 Flat Top PAM4 1310nm MTP/MPO-12 500m SMF FEC Optical Transceiver Module $800.00
-
NVIDIA MMA1Z00-NS400 Compatible 400G QSFP112 SR4 PAM4 850nm 100m MTP/MPO-12 OM3 FEC Optical Transceiver Module $650.00
-
NVIDIA MMA4Z00-NS400 Compatible 400G OSFP SR4 Flat Top PAM4 850nm 30m on OM3/50m on OM4 MTP/MPO-12 Multimode FEC Optical Transceiver Module $650.00