In our ever-changing digital world, high-performance computing is increasingly needed – particularly in data centers supporting artificial intelligence (AI) workloads. As enterprises look to capitalize on AI for better decision-making and operational efficiencies, there needs to be an evolution in underlying networking solutions that can handle more data throughput and computational power. NVIDIA’s networking solutions are instrumental in this change by providing connectivity transformation with data-intensive applications through fast-speed, low-latency network architectures. This article discusses the benefits of such a solution in terms of performance improvements and operational streamlining which allows businesses to realize their potential during this era of AI revolution fully. We shall also show how these technologies affect modern data centers and future AI workloads based on examples drawn from various NVIDIA products like BlueField® Data Processing Unit alongside the DOCA software framework.
What is NVIDIA Networking?
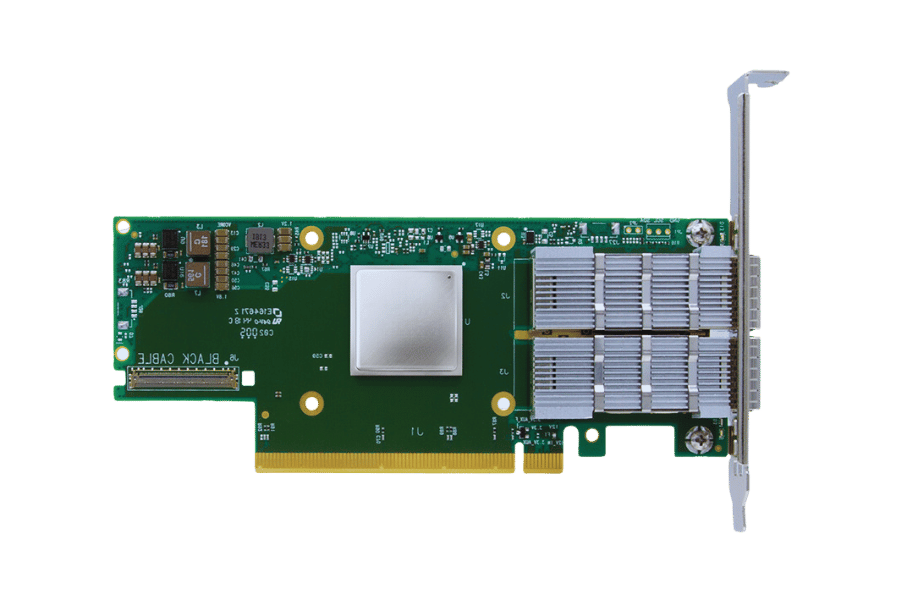
Understanding NVIDIA and Mellanox Integration
The networking capabilities of NVIDIA have been greatly improved by its integration with Mellanox Technologies, which has brought a wide range of data center and artificial intelligence (AI) workload solutions to the table. This blending combines state-of-the-art GPU technologies from NVIDIA with leading high-speed interconnect solutions such as InfiniBand and Ethernet provided by Mellanox. The outcome is a strong network architecture that increases data throughput while decreasing latency, both essential for AI systems dealing with huge volumes of information in real time. Organizations can scale higher and faster to achieve efficiency thanks to this symbiosis that ensures quick, seamless processing across many nodes within a data center. What makes the partnership between Nvidia and Mellanox stand out is their strategic approach toward satisfying contemporary computational requirements and optimizing the networking landscape.
Core Benefits of NVIDIA’s Advanced Networking Technologies
NVIDIA’s original networking technologies offer many benefits that are key to improving the performance of data centers. In the first place, they make possible communication with ultra-low latency, something essential for real-time data processing and AI training. Next, their high bandwidth capabilities enable quick transfer of huge datasets between servers and GPUs, thus accelerating workloads and increasing resource utilization. Furthermore, these technologies have advanced network features such as congestion control or adaptive routing, which optimize the flow of information and minimize bottlenecks in them. As a whole, therefore, NVIDIA’s networking solutions create a more agile and efficient infrastructure that allows enterprises to scale their operations effectively while staying competitive in this fast-changing AI landscape.
Role in Data Center Optimization
The optimization of data center operations is highly reliant on NVIDIA’s networking technologies. These solutions reduce delays and increase bandwidth in order to fully exploit computing capabilities, thus enhancing the speed of processing information and managing workloads better. Besides that, they also incorporate smart congestion control, among other advanced functionalities, which are responsible for dynamic load balancing, leading to the efficient allocation of resources, thereby averting bottlenecks. Such an all-encompassing method improves performance and supports scalability so that data centers can quickly adjust themselves according to variable requirements. Therefore, businesses are able to make their IT systems more flexible and cost-efficient which strengthens competitiveness within the industry.
How Does NVIDIA Infiniband Accelerate Computing?
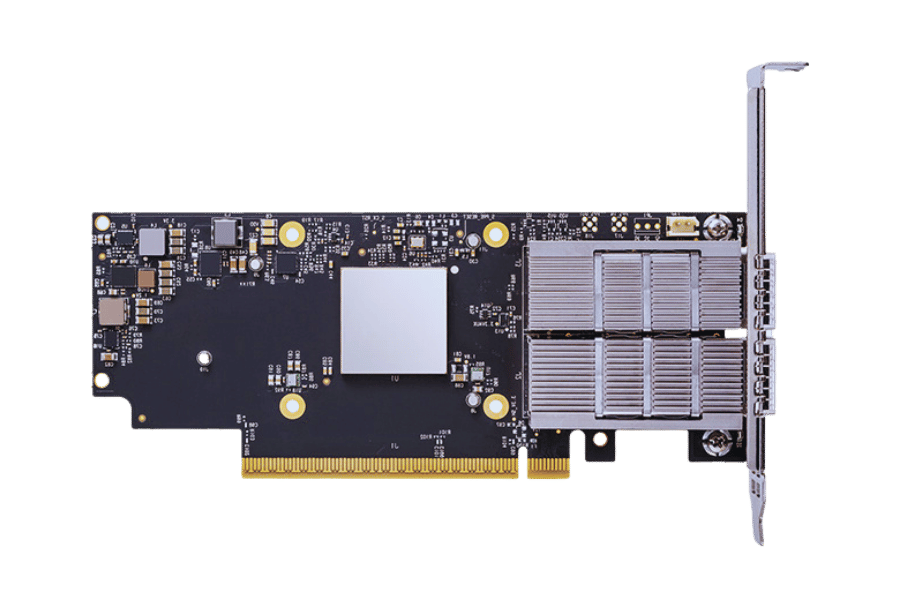
Key Features of NVIDIA Infiniband
NVIDIA InfiniBand technology was built to support high-performance computing (HPC) and data centers at the enterprise level. Some of its main features are its ability to handle complex computing and AI workloads:
- High Throughput with Low Latency in Datacentre Environments: InfiniBand delivers unmatched data rates, often surpassing 200 Gbps, and does it with ultra-low latency – just a few microseconds. This is important for applications that need fast and reliable communication between compute nodes.
- Scalability: With support for thousands of nodes, InfiniBand can scale up to meet the needs of large data infrastructures or supercomputers. It enables easy growth without major network reconfiguration thanks to its support for big topologies.
- Advanced RDMA Capabilities for Complex Compute and AI Workloads: By allowing direct transfer of data between nodes’ memory without CPU involvement, Remote Direct Memory Access (RDMA) reduces inefficiency while freeing up processing resources for other tasks. This improves overall performance through offloads and in-network computing in distributed applications.
- Enhanced Reliability: Among error correction features included by NVIDIA InfiniBand are congestion management algorithms, which ensure integrity as well as continuity of communication required for critical workload performance.
- Support for Mixed Workloads: InfiniBand efficiently handles both computational tasks and data storage operations, catering to mixed workload environments and optimizing resource utilization across different applications.
Such capabilities collectively provide organizations with an opportunity to construct powerful computing environments capable of effectively handling demanding computational tasks, thereby further improving their operational potential.
Impact on High-Performance Compute Applications
Linking NVIDIA InfiniBand into the system allows it to operate much better. The industry findings have revealed that this architecture is responsible for speeding up workloads, especially those dealing with machine learning, simulations, and large scientific computations, among others, which are data-intensive. Moreover, advanced remote direct memory access (RDMA) capabilities can cut down on CPU overheads thereby enabling efficient processing of information and quicker completion times for parallel tasks. Efficiency becomes necessary when dealing with such sectors as bioinformatics, astrophysics, or financial modeling, where huge volumes of data need immediate analysis or processing. Another thing is that scalability within InfiniBand networks supports the growing requirements of enterprises during capacity expansion in computation resources, thereby ensuring sustained peak performance even when demand rises. All in all these technical benefits make Infini Band a must-have element for driving high-performance computing applications since they enable establishments to achieve breakthrough results.
Advantages Of Traditional Networking Solutions
NVIDIA Infiniband has a number of benefits over conventional network solutions that make it more efficient for overall system performance. First, Infiniband has low-latency communication, which allows faster data transfer between nodes than traditional Ethernet networks do, thus being very crucial to time-constrained operations in HPC. Secondly, the large bandwidth capacity offered by InfiniBand ensures good throughput hence enabling bottleneck-free handling of massive data transfers like those involved in real-time analytics or simulation workloads.
Additionally, this protocol greatly reduces CPU overhead through support for remote direct memory access (RDMA) where data can be moved directly between memories without involving the processor thereby freeing computational power for other tasks thus leading to shorter processing periods and better application response times. Furthermore, the scalability of Inifiniband enables organizations to easily scale up their networks so as to meet increasing project needs in terms of both connection numbers and data volumes, something not possible with conventional network solutions, which lack such flexibility.
To put it briefly, Nvidia’s Infiniband is an excellent choice for high-performance computing applications due to its combination of low latency, high bandwidth, reduced CPU utilization, and scalability features that establish a strong foundation upon which future computational advances can be built.
What is NVIDIA’s BlueField Networking Platform?
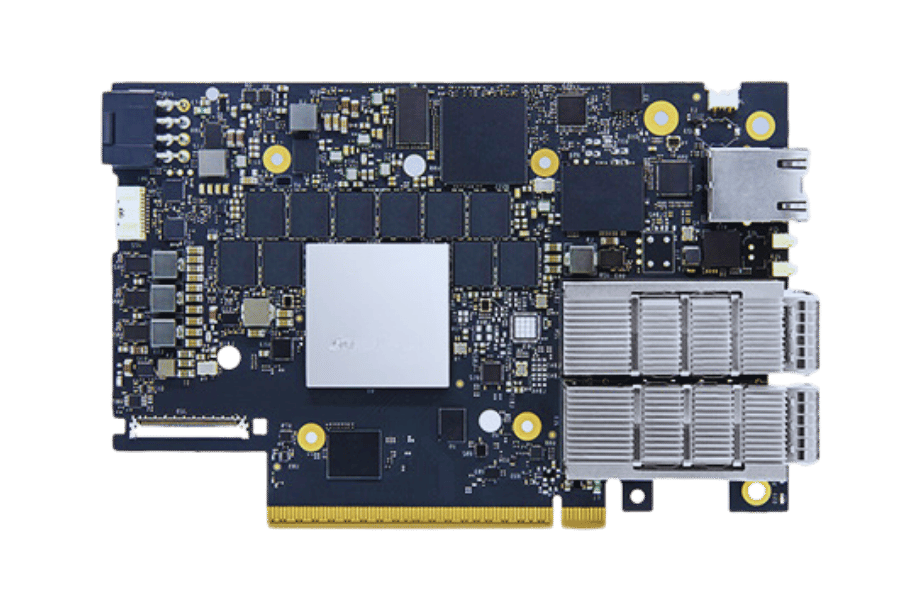
Bluefield DPU Overview
NVIDIA’s BlueField Data Processing Unit (DPU) is a revolutionary system created to develop data center infrastructure through unburdening, speeding up, and protecting fundamental networking, storage, and security functions. It combines state-of-the-art ARM-based processors with NVIDIA’s high-speed Ethernet and InfiniBand networking capabilities so as to increase efficiency and performance in cloud, enterprise, and edge environments. Taking data-centric workloads away from the CPU enables better resource utilization while also enhancing overall data processing speed. More so, this can facilitate real-time insights and analytics by enabling smart data processing through NVIDIA’s software ecosystem. The BlueField DPU brings together networking functions with storage functions as well as security functions into one powerful appliance; this makes it an essential element for any contemporary high-performance scalable data center environment.
Benefits of Bluefield for AI and Machine Learning
NVIDIA’s BlueField DPU can be extremely useful for AI and machine learning because it refines data management and improves overall performance. Here’s why:
- More efficient data flow: BlueField’s architecture allows high-speed access to information, which reduces latency and speeds up data feeds to AI models. This is especially important when training on large datasets, where time taken greatly affects the results achieved.
- Offloading Resources: BlueField moves networking and storage tasks from CPU to DPU, thus lowering computations’ load on main processing units. This enhances resource allocation so that central processors can concentrate on more complex artificial intelligence algorithms without being slowed down by routine data controls.
- Better Security for Data Integrity: The security measures built into BlueField make sure that while being processed or transferred, information remains safe. Such protection becomes necessary in cases where AI applications deal with personal/private records or should comply with strict regulations concerning their safeguarding.
- Adaptability towards heavy computing workloads and complex AI systems: In view of expanding workload sizes associated with artificial intelligence, BlueField’s scalable nature enables it to respond dynamically by providing additional processing power coupled with the bandwidth required to support growth without compromising efficiency.
If maximized, these advantages can enable businesses to achieve optimal efficiency gains through the adoption of AI/ML technologies, which foster innovation across different spheres of their operations.
Scalability and Efficiency in Data Centers
Data centres are a very important part of the management of big data, and there is a need for adaptable solutions. To improve their scalability and efficiency, modern data centers were built on certain principles, which include:
- Modular Infrastructure: The majority of leading data centers use a modular design that allows quick deployment of additional resources when necessary. This modularity ensures that organizations can scale up their operations without disrupting them thus effectively dealing with different workloads.
- Energy Efficiency Technologies: Energy consumption should be efficient. Therefore, contemporary data centers have adopted advanced cooling systems, green hardware, and other renewable sources to minimize carbon output while still maintaining peak performance.
- Virtualization and Automation: Resource utilization is maximized in data centers through the use of virtual machines, which allow several VMs to run on one physical server. Operations can also be made more efficient by automation software, which cuts down on human errors and ensures resource allocation based on live demand.
These strategies combine greatly to improve scalability and operational efficiency within data centers, making them capable of meeting current business needs.
What Are NVIDIA’s Ethernet Networking Solutions?
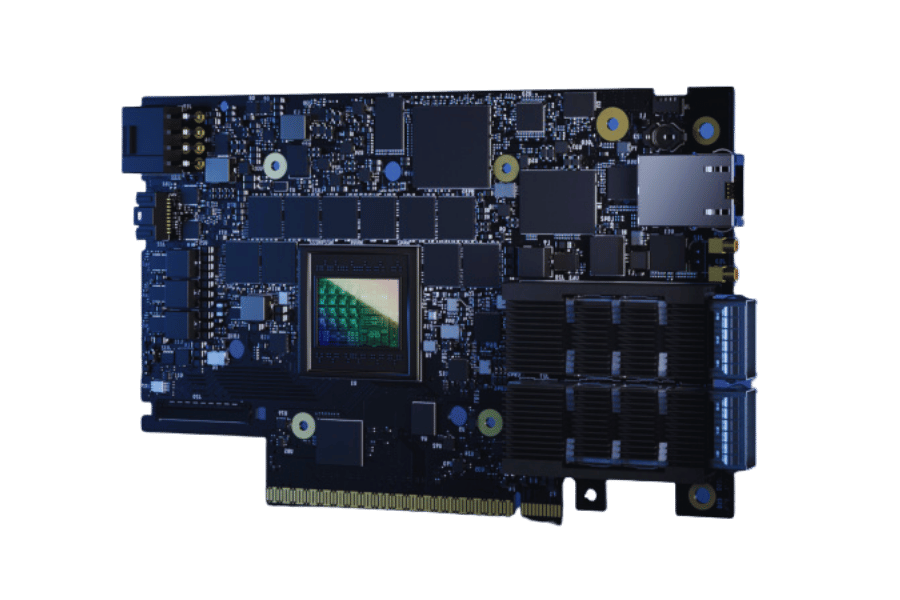
Exploring Spectrum Ethernet Switches
NVIDIA’s Spectrum Ethernet switches are designed to be data center and cloud networking solutions for high-performance computing while providing security services for complex computing. These switches offer a number of advanced features, such as ultra-low latency, high bandwidth, and scalability, which allow them to handle heavy workloads. One of the main features is its support for Ethernet speeds of up to 400GbE that enables faster data transfer rates thus improving overall system efficiency.
The Spectrum family also has integrated telemetry for real-time monitoring & management along with support for advanced network automation protocols like those found in SDN environments; this greatly simplifies operations across various types of data centers and provides better orchestration capabilities among them. Through AI & ML powered intelligent networking solutions that can adapt to changing data needs and optimize resource utilization, Spectrum switches based on NVIDIA hardware greatly enhance performance reliability demanded by today’s digital landscape.
Comparing Ethernet and Infiniband Solutions
Comparing Ethernet and InfiniBand reveals a number of key differences that reflect their different data center application suitability, such as offloads and in-network computing. Its ability to support data rates ranging from 1GbE to 400GbE and beyond has made Ethernet the networking standard because it is versatile, cost-effective, and easy to deploy. This wide use, coupled with compatibility among various network infrastructures, makes it an excellent choice for general-purpose networking.
On the other hand, InfiniBand is designed specifically for high-performance computing (HPC) environments, offering higher bandwidths up to 200Gbps and lower latencies than traditional Ethernet. It achieves this through employing a switched fabric architecture, which allows for efficient parallel processing, especially useful in applications needing fast data transfers like big data analytics or AI workloads.
All technologies have their own strong areas—flexibility/compatibility being one of them for Ethernet, while performance/speed being another one, particularly for demanding applications, when it comes to Infiniband. As companies expand their activities further, whether they go for Ethernet or InfiniBand will depend mainly on specific performance needs as well as operational goals within given environments.
Deploying NVIDIA’s Latest Ethernet Network Technologies
NVIDIA is known for its Ethernet network technologies, which aim to improve data throughput and reduce latency in modern data center environments. The most recent additions to its lineup are the Spectrum series switches. These switches have been designed for AI and machine learning workloads that require high bandwidth connections with low latency. They use advanced SDN capabilities that optimize network management by automating resource allocation based on dynamic adjustment according to workload requirements.
Moreover, by integrating GPU-accelerated networking solutions, NVIDIA ensures that information is processed and transferred faster across networks. Data centers can achieve higher efficiency levels as well as scalability thanks to programmable packet processing and hardware offload among other technologies provided by this company. Therefore, before implementing any of these technologies, it is important for organizations to take into account their existing architecture, how compatible they are with current systems, as well as specific application needs so as to exploit advantages brought about by NVIDIA’s Ethernet solutions fully.
How Does NVIDIA Optimize Network Performance?
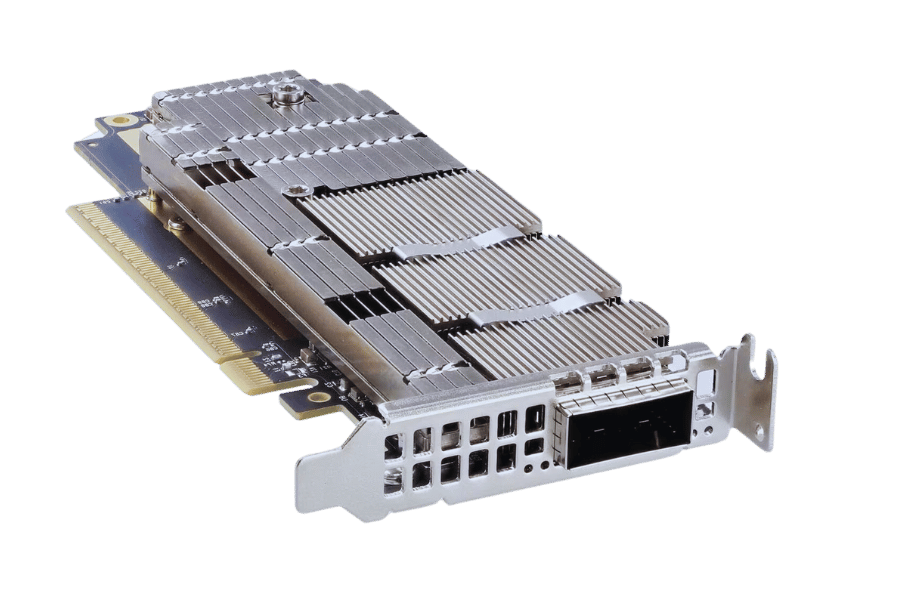
Advanced Networking with NVIDIA
To advance the throughput, reduce latency and improve efficiency in data centers, Nvidia optimizes network performance by means of several key innovations. Initially, RDMA technology was used by the company, which permitted quick data transfer between servers without overburdening the CPU, leading to decreased latency and improved application response time. Another important contribution comes from Mellanox ConnectX network adapters that support high bandwidths as well as low-latency connections, thereby making them suitable for workloads with a lot of data.
Moreover, Nvidia integrates programmable networking technologies to enhance network performance, thereby enabling data centers to configure their networks dynamically according to specific application requirements. By combining these programmable switches with their high-performance networking adapters, such as Spectrum switches, enterprises can automate resource allocation based on current workload demands while managing networks in real time. This all-around method ensures that NVIDIA’s networking solutions meet and exceed modern application needs, particularly within AI and machine learning environments.
Techniques for High-Speed Data Transfer
High-speed data transfer methods are needed to make networks faster, especially in environments that handle large amounts of information. Here are some notable examples;
- Employing RDMA Technologies: This allows for direct memory-to-memory data transfer between computers without involving the CPU, thereby reducing latency and increasing throughput. In fact, this is paramount when it comes to data centers, as they can greatly improve overall performance by quick data processing with the help of smart NICs.
- Implement Multi-Channel Networking: Multi-channel networking uses multiple connections to increase bandwidth and reliability. When an organization spreads its information across different highways, congestion is reduced, leading to faster communication between devices, particularly with network interface cards.
- Optimize Network Protocols: Advanced network protocols like NVMe over Fabrics can accelerate the rate at which data moves between storage and server applications. These protocols boost flash storage performance, which becomes crucial as more demands for information arise.
These methods are necessary, among other things, to ensure that networking infrastructures can support modern applications, given that organizations are increasingly adopting workloads characterized by higher data volumes.
Leveraging AI for Network Efficiency
To automate processes, predict possible failures, and optimize the allocation of resources, Artificial Intelligence (AI) is very important in enhancing network efficiency. The following are some recent developments:
- Predictive Analytics: AI-driven tools that analyze historical network data can predict traffic patterns and potential overloads, thereby allowing adjustments to be made in advance for sustained performance. This method reduces downtime more than any other approach since it improves user experience.
- Automated Network Management with NVIDIA Accelerated Technologies: AI systems use real-time data analysis to autonomously manage network configurations and adjustments. This ensures that the networks adapt dynamically to changing workload demands, improving overall throughput and reliability.
- Anomaly Detection and Security: AI algorithms monitoring network traffic can detect common patterns that indicate security threats. Organizations can protect their sensitive data against cyber attacks by strengthening defense mechanisms through quick identification and response to these anomalies.
The integration of AI into network management not only makes operations easier but also raises the performance ceiling because organizations are striving to meet the growing needs of digital infrastructure. As this technology advances, its ability to revolutionize network efficiency will grow, too.
Reference Sources
Frequently Asked Questions (FAQs)
Q: What are NVIDIA’s latest data center networking solutions for AI workloads?
A: Up-to-date solutions such as Quantum Infiniband and NVIDIA BlueField-3 DPUs ensure high-performance networking. These solutions offer reliable end-to-end connectivity, scalable architectures, and low latency for AI applications and data centers.
Q: How does Quantum Infiniband support accelerated networking?
A: Quantum Infiniband provides the high bandwidths and low latencies required for accelerated networking. It helps to transfer data between GPUs and CPUs effectively, ensuring smooth operation in AI and high-compute environments.
Q: What role do NVIDIA BlueField-3 DPUs play in modern data centers?
A: Integrating software-defined networking, storage networking, and in-network computing with NVIDIA BlueField-3 DPUs is a major step forward for modern data centers. This enables CPUs to offload network tasks more efficiently, freeing them up for heavier compute workloads.
Q: How do NVIDIA networking solutions handle both Ethernet and Infiniband technologies?
A: They are flexible enough to support either Ethernet or Infiniband technologies, thereby accommodating the different needs of data centers or AI workloads. Quantum Infiniband is one of the technologies used to provide high-speed interconnects, while Spectrum-X manages high-performance network interfaces.
Q: What benefits does RDMA (Remote Direct Memory Access) offer in NVIDIA networking solutions?
A: When using RDMA within these systems, distributed AI applications and other types of HPC tasks that involve large amounts of transferred data can perform significantly better. With it, GPUs can communicate directly with storage and other compute resources without going through the CPU, thus greatly reducing latency.
Q: Explain why NVIDIA BlueField® DPUs are good for AI workloads.
A: NVIDIA BlueField® DPUs offload networking, security, and storage tasks from the CPU to accelerate AI workloads. In this way, they free up more computing power for use in artificial intelligence applications, thereby increasing the performance and efficiency of data processing.
Q: What does in-network computing do for high-performance networking solutions developed by NVIDIA?
A: It allows computation within the network itself, reducing data movement and improving overall application performance. This is important for scalable AI systems that involve many complex workloads.
Q: How has software-defined networking been addressed with Nvidia’s new products?
A: Their Spectrum-X platforms, together with BlueField® DPUs, have made it possible to implement automation and manage networks efficiently under a software-defined environment. These technologies allow for dynamic configurations that can adapt to different requirements within modern high-performance data centers.
Q: Why should one use ConnectX network adapters in data centers?
A: ConnectX network adapters offer low-latency, high-I/O throughput connectivity solutions ideal for use in data centers where such capabilities are needed most frequently. Furthermore, these adapters support both Ethernet protocols and Infiniband protocols, thus guaranteeing flexible and dependable network performance across all types of setups.
Q: How can NVIDIA’s networking solutions achieve automation in data centers?
A: Network automation becomes achievable when routine tasks are offloaded through RDMA-enabled adapters provided by Nvidia coupled with BlueField-3 DPUs. This enables administrators to configure networks using software easily while ensuring that these operations consume fewer resources than before, leading to better efficiency levels within them.
Related Products:
-
NVIDIA Mellanox MCX653106A-ECAT-SP ConnectX-6 InfiniBand/VPI Adapter Card, HDR100/EDR/100G, Dual-Port QSFP56, PCIe3.0/4.0 x16, Tall Bracket $828.00
-
NVIDIA Mellanox MCX653105A-ECAT-SP ConnectX-6 InfiniBand/VPI Adapter Card, HDR100/EDR/100G, Single-Port QSFP56, PCIe3.0/4.0 x16, Tall bracket $690.00
-
NVIDIA Mellanox MCX653106A-HDAT-SP ConnectX-6 InfiniBand/VPI Adapter Card, HDR/200GbE, Dual-Port QSFP56, PCIe3.0/4.0 x16, Tall Bracket $1600.00
-
NVIDIA Mellanox MCX653105A-HDAT-SP ConnectX-6 InfiniBand/VPI Adapter Card, HDR/200GbE, Single-Port QSFP56, PCIe3.0/4.0 x16, Tall Bracket $1400.00
-
NVIDIA Mellanox MCX75510AAS-NEAT ConnectX-7 InfiniBand/VPI Adapter Card, NDR/400G, Single-port OSFP, PCIe 5.0x 16, Tall Bracket $1650.00
-
NVIDIA Mellanox MCX75310AAS-NEAT ConnectX-7 InfiniBand/VPI Adapter Card, NDR/400G, Single-port OSFP, PCIe 5.0x 16, Tall Bracket $2200.00